Webinar
Storytime: Innovative Uses of High Performance Computing
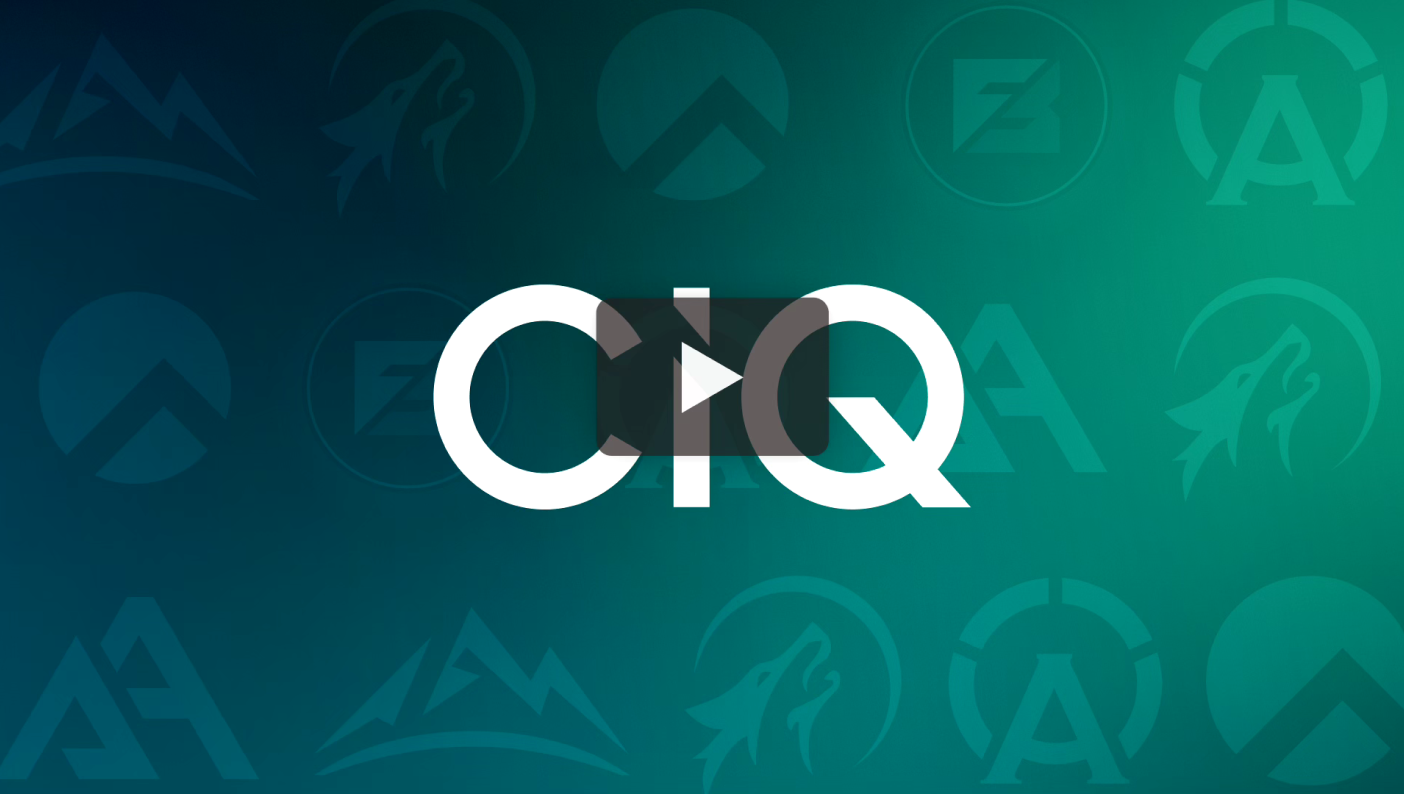
The field of High Performance Computing (HPC) has experienced rapid growth in recent times, leading many to wonder how professionals in HPC got started. This unique webinar offers a rare opportunity for people to learn from experienced HPC professionals about their journey to success.
Join us for an engaging and educational session in which a panel of experts will recount their experiences, their entry into the world of HPC, the obstacles they faced, and their strategies to overcome those these challenges. Our panelists come from diverse backgrounds and have different perspectives on what it takes to succeed in the HPC industry. Whether you’re a student, professional, or just interested in HPC, this webinar will provide valuable insights and tips to help you navigate your journey into the HPC field.
Webinar Synopsis:
Speakers:
-
Zane Hamilton, Vice President of Sales Engineering, CIQ
-
Gregory Kurtzer, Founder of Rocky Linux, Singularity/Apptainer, Warewulf, CentOS, and CEO of CIQ
-
Brian Phan, Solutions Architect at CIQ
-
Forrest Burt, High Performance Computing Systems Engineer, CIQ
-
Jonathon Anderson, HPC System Engineer Sr., CIQ
-
Alan Sill, Managing Director, Senior Director of High Performance Computing Center at TTU; Adjunct Professor of Physics, Texas Tech University
Note: This transcript was created using speech recognition software. While it has been reviewed by human transcribers, it may contain errors.
Full Webinar Transcript:
Zane Hamilton:
Good morning, good afternoon, and good evening, wherever you are. Welcome to another CIQ webinar. Thank you for that great intro. I guess we all just got rick roll'd. My name is Zane Hamilton and I work at CIQ. At CIQ, we're focused on powering the next generation of software infrastructure, leveraging capabilities of cloud, hyperscale and HPC. From research to the enterprise, our customers rely on us for the ultimate Rocky Linux, Warewulf, and Apptainer support escalation. We provide deep development capabilities and solutions, all delivered in the collaborative spirit of open source. So this week we are going to have panel and we're going to talk about stories in HPC. What are people doing with HPC? What are they doing to be innovative and how are they utilizing it today? So I could bring in the panel. Hopefully they're all still laughing. Forrest, I blame Greg. Actually, I do blame someone else and talk to them later. So welcome everyone. Good to see you guys.
Gregory Kurtzer:
I had nothing to do with it, Zane, it wasn't me.
Zane Hamilton:
Yeah, absolutely. I know who it was. I know who it was. I know who both of the people were there. There's probably two, maybe three people involved in this one. All right, so this week we're having story time. I'm excited for this because it's always interesting to hear what people are doing with h HPC coming from outside of HPC. I love to see things and see what people are doing. I know that some of you, especially you that have come from academia, have good stories to tell. So I'm going to start off and everybody introduce themselves. Brian, if you don't mind.
Introductions [1:45]
Brian Phan:
Hi everyone. Good to be back on the webinar. My name is Brian. I'm a solutions architect here at CIQ. My background is in HPC administration and architecture with some expertise in CAE and Genomics.
Zane Hamilton:
Thank you Brian. Forrest, welcome back.
Forrest Burt:
Thank you. Great to be back on the webinar. My name is Forrest Burt. I'm a high performance computing systems engineer here at CIQ. My background comes out of the academic and national lab, HPC sphere. So I've seen a lot of different, more academia research, national lab focused use cases and am excited to share some stories.
Zane Hamilton:
Thank you Forrest. Jonathan, welcome back.
Johnathan Anderson:
Yeah, thanks. My name's Jonathan Anderson. I'm on the Solutions architect team as well. And like Forrest, my background is in academic HPC in the national lab and higher ed space.
Zane Hamilton:
Thank you. Greg, I think you should have to sing your intro personally.
Gregory Kurtzer:
Don't hold me totally to blame. Just for everybody's knowledge, there are Rick Roll wars happening at CIQ. And so yeah, I think I know somebody who gets extra points for this one. Anyway, my background is in biochemistry and then Linux, open source, and then HPC. And now I'm at CIQ, Rocky Linux and doing some other cool stuff with open source projects like Apptainer and Warewulf.
Zane Hamilton:
Thank you. So I'm going to open it up. I know some people have stories. Greg, I know you've always got stories, so I'm not going to start with you this time, but I'm going to start with Forrest. So, coming out of academia, I know you see a lot of different things. There's a lot of probably fun things and some things that are not so fun. But I would love it if you kick us off and share a story of how people are utilizing HPC and something that's innovative and interesting.
How the Peregrine Fund Uses HPC [03:44]
Forrest Burt:
Yeah, absolutely. So like I said, I came out of the academic sphere. So I was at an academic institution for about two and a half years doing research computing work on the research computing team. The first use of HPC that comes to my mind that I was very, very interested in while I was there was some work that a group called the Peregrine Fund was doing while I was there. The Peregrine Fund had a couple researchers on our cluster. They bought a node, so they had some dedicated hardware that they were working on. Eventually we went on and made some modifications, added more RAM and stuff because they purchased some upgrades to their node. But basically, they were in our environment as a third party that was utilizing our resources because we had major cluster at Boise State where I was at.
So we got them onboarded. They eventually decided to take on their own hardware. And the work that they were doing, like I said, was incredibly interesting. There were two different researchers. The first one was working with taking these massive data sets of data points that were collected from these... Obviously this is the Peregrine fund, they deal with endangered birds and that type of thing, habitat preservation and all that. So on our supercomputer, the first researcher from there was using HPC resources to do more massive competition than she'd be able to, data analysis, that type of thing on this data being generated from these birds. So they would have golden eagles, these backpacks that they would put on them out in the field.
And as these eagles traveled around, this data would get pinged back, it would get collected. And they eventually had like 600,000 data points or something like that from all these different eagles, how they did habitat selection, where they lived at, where they stayed at for different periods of time. Before being able to get onto HPC, the analysis that they were doing was pretty basic. They had to consider all of the eagles basically as one bird. I believe as far as habitat choice, preference, that kind of thing goes. It was much more simplified of a model that they were building. After they were on HPC they were able to do things like take these data points, apply different models of habitat selection and stuff to them to view them all as more individual generators of data as individual birds in the set.
That was a really, really interesting project. Like I said, a lot of this data was tracking out eagles travel over North America. And that was interesting. The other side of it that they were working on was also very interesting. They had these systems on wind turbines called an identi-flight system that was essentially a set of cameras on it that would track as a bird was flying towards the wind turbine, the three-dimensional path that it took through the air. And so this researcher had all of this information from these identi-flight devices. And he was able to try to build models to analyze what flight paths are most likely to result in a collision between a bird and a wind turbine. So that wind turbines can essentially be schooled down when an eagle or something like that is on what's perceived to be a collision course.
And that took huge amounts of computational power. That was image analysis, that was like flight path analysis, things like that. So the HPC in that case ended up leading to an increase in the complexity that he was able to look at. But I love this project. These two people were a ton of fun to work with. Like I said, the work was super interesting and was about preserving endangered birds. I did a lot of this out of containers, so they had a really, really specific environment that had a lot of different components to it, a lot of different pieces of software. So this is one of the first major containerized deployments that I did, built out at the time, singularity, now container but a container that had all their different custom tooling, a bunch of different R packages, a bunch of different stuff like that. And continuously deployed that out to them with new updates, new R packages, that type of thing. That was a fascinating project. Like I said, it was interesting to see how they were able to massively increase the complexity of their models and the research that they were doing around habitat preservation. Like I said, wind turbine placement and operation, very interesting, unique work within HPC.
Zane Hamilton:
Welcome Dr. Sill. Always glad to have you. I do have a question for you, Forrest, on that first use case, whenever they're doing that type of data and collecting that data for that data set, were they going back and overlaying that on top of like a map and then diving down and doing more research on the actual environment itself? Once they start using the data and they've crunched it, what are they doing with it? What decisions are they making?
Forrest Burt:
I think it was meant to inform conservation decisions, habitat preservation decisions, stuff like that at the state or national level. So they had these backpacks that tracked these birds far into locations that it would be very, very difficult for humans to observe them in. And they were able to take, like I said, location data, that type of thing. And yeah, overlay it on maps versus like the typography that the landscape had to find out how eagles were picking their habitats, how they were staying in them, what forced them to have to move out of habitats.
Zane Hamilton:
Interesting.
Forrest Burt:
Did I answer your question, Zane?
Zane Hamilton:
Yeah, absolutely. That's great. Thank you, Forrest. Dr. Sill, welcome. It's always good to see you.
Alan Sill:
Hi there. Nice to be here. It's spring break here, so relaxing a bit.
Zane Hamilton:
Oh, that's nice. Thank you for joining on your spring break. So for spring break, do you have any interesting stories or interesting things that you're seeing people use HPC for right now?
Finding the Top Cork [10:35]
Alan Sill:
I'm just trying to pull something out of the hundreds of different applications. Just a regular university cluster, all the innovative stuff is swamped by AI topics these days. And I don't want to get down that rabbit hole. I can tell you a story of how we found the top cork using Linux clusters.
Zane Hamilton:
Let's hear it.
Alan Sill:
Okay. So this date's back a bit. It was the mid nineties. We were trying to find the top cork. And I have to tell you that the experiments were built in a way in which the computing was not expected to be able to keep up with the data flow. So you know what particle physics collisions are like, you've probably seen pictures. Particles, in this case, protons and antiprotons collided in the middle of a big complicated detector. And you get all this information at a very high rate. So the detectors were built to pick out very high energy leptons and very high energy electrons or muons or very imbalanced energy and then to try do tracking around the path towards that electron. So you get a big splash of energy in an outer detector in what they call regional tracking.
You just sort of connect all the little hits of the tracking detectors to try to find the path that particle took. And nobody ever expected you to analyze all of the tracks in an event much less the billions of collisions. You can only write a few dozen of them per second to disk. So we said about trying to solve that problem and at that point, the fastest things we had were alpha clusters, deck alphas, and something called deck net so we could build clusters of these things. And one day a friend of mine came in and said, we built a Linux cluster. And I said, well, why'd you do that? And he said, no, no, trust me. This is going to be good. And within six months I got put in charge of a project to tell people all over the world how to duplicate the cluster we had built at Fermilab, because they wanted to build them too. And this was considered a distraction. So I was working on other things. They said, Alan, why don't you do this? I basically put up a webpage and said, here, do exactly this and if you do exactly this, then you have problems, call me. Otherwise, have a nice day.
But what we found was that they get these clusters built and they would call us up and say, we want to participate in data analysis. And we realized we had stumbled upon a method to get the orders of magnitude and more computing we needed. First of all, we were able to get a lot more CPUs in a cluster than we used to get in single machines. And then we had lots of people building clusters. So we brought up a couple of dozen of these things all over the world. And that basically was one of the streams that became great computing. Oh, we forgot to ask people for their credit card numbers. Jeff Bezos came along a few years later and figured that out and he's very rich and I'm still working for a living. But the top cork was found because we were able to build the next clusters.
Zane Hamilton:
That is very cool. It seems like, especially then, that storage would be the bottleneck in that, right? So I mean, if I remember correctly, there's terabytes of data that get written every time you run those experiments. And trying to put that on spinning discs seems not exactly efficient.
Alan Sill:
Well, it's an interesting question because of how it played out. It was actually the network that was the bottleneck, getting things across all these different distributed computers. But right along then the National Science Foundation had really boosted the speeds of the networks. And then that had evolved into the point where commercial companies were involved and the academic sector stayed engaged. And a friend of mine who just retired from DOE actually started going around asking people a different question. He started saying, what would this look like? And this is the question I want to put into people's heads today. What would this look like if networks were infinitely fast? How would you design it if networks were not a bottleneck? because we were doing all these things trying to limit what we put on the network. And he said, no, no, let us solve that problem.
Tell us what you would do if you did that. And so we told him, and so networks got a lot faster and we started using them and then they, that justified the investment. And so now I actually meet people, faculty candidates, and they come in and say I need a dedicated 64 cores. And I looked at him, I said, what would your science look like if you had an infinite amount of computing? And actually that's the question I think people ought to start asking. And if the answer is ChatGPT, please don't talk to me. But if it's something else, maybe we can talk.
Zane Hamilton:
Oh, that's fantastic, Alan. Thank you. Brian, I'm interested to hear, interested to hear a story.
Using HPC in Commercial Supersonic Air Travel [16:05]
Brian Phan:
So my background is actually more on the enterprise side of things. So I used to sell HPC SaaS products to enterprise customers who are doing r and d for whatever products they're developing. One of the cooler products that a customer was developing was a supersonic passenger airplane. So they're basically trying to bring back supersonic air travel, commercial supersonic air travel back. I know that I think the Concorde was retired back in like '03. So they basically used our HPC SaaS products to run physics simulations for aerodynamics and also structural analysis to basically look at the structural integrity of the plane going at Mach 1.7. At the end of the day, the products that you and me would probably consume are basically commercial flights that could take us from maybe San Francisco to Tokyo in like three hours or also maybe New York to London and also three hours. And this is probably coming maybe in the next three to five years. So yeah, it's very exciting times.
Zane Hamilton:
That's amazing. I remember I always wanted to ride on the Concord, so I was really sad when they retired it. I did see somewhere recently that a group purchased some of the airframes and was trying to revive that program. I don't know where that stands, but I thought that was interesting because it seems like there may be better solutions now than an airframe from the seventies. Thank you, Brian. Jonathan.
Rendering High Resolution Tumor Scans Through HPC [17:45]
Johnathan Anderson:
One of the places that I worked was Mount Sinai Medical School in New York, now known as ICAN School of Medicine in Mount Sinai. And one of the things I really enjoyed about working there was the immediacy and the practicality of the work that we were doing. You could see the line between the research that was happening on the HPC systems and patient care in some instances that was really cool. And there may be lots of steps between them, but it just felt really immediate and practical. And so in theory, that cluster was largely used for genetics and genomics research. And then there were some other groups that were using it for things like molecular dynamics, drug design, that kind of thing. But one of my colleagues there, a guy named Anthony Costa, who is with NVIDIA now, if he's watching, Hi, he was really interested in modeling and was interested in 3D printing and things like that on the side.
And started working with someone in the cancer department or cancer research department or whatever. A research department where he was studying that and they started working on taking CT scans and scans of people's tumors and rendering them in high resolution through runs on the HPC system. And then ultimately rendering them out as physical objects that they could print out with these high resolution industrial scale 3D printers. And he had a couple of stories of patients who, and this my impression of this, this isn't my story, so he'd probably correct this with more color, but there's this sensation of a loss of control when you're undergoing care in a situation like that and with cancer especially, there's some part of your body that you're not in control of and you're not able to understand it. And the tactility of being able to see exactly what it is that is harming you, I think helped a number of patients that they were able to trial this with. And I thought that was really cool that this abstract concept of a tumor that you might have rendered as a physical object that you could see exactly what it is was a really interesting thing that I hadn't ever seen anyone do before.
Zane Hamilton:
That is very cool. On the 3D printer thing, I saw somewhere recently in an article that they had 3D printed a skull, so somebody had a severe brain trauma and they actually 3D printed some sort of material for the skull to actually replace and reform his head. So it seems like they're doing a lot of fascinating stuff, not only with HPC, but in the 3D printing realm as well. Yeah, it's very cool. Okay, Greg, I guess you can talk today, even though I'll get you back.
Gregory Kurtzer:
I was just thinking, could we use HPC to do a deep fake of Rick Asley in one of our webinars?
Zane Hamilton:
Don't tempt Forrest, now you've given him a challenge.
Forrest Burt:
Yeah, don't. Hold on a second, let me get on that. I'll see what we can put together.
Alan Sill:
There are copyright issues I think.
Johnathan Anderson:
I have to go check the board and make sure that doesn't appear now.
Alan Sill:
No, I actually think that they're trying to tamp down on this, the Rick Rolling I think they're trying to assert copyright over it now. So who knows?
Zane Hamilton:
What's the rule, over like seven seconds?
Midrange Computing [21:46]
Gregory Kurtzer:
Per use, like the amount of time that you're allowed to use, like a video or something. And I think our intro this morning wasn't longer than that, but it's I think probably one of the best things that's ever happened to Rick Astley. I mean, he had this major hit back in the late eighties, early nineties, I think, somewhere around there. And all of a sudden he made a comeback, like everybody knows never going to give you up now at this point.
So in terms of HPC stories, the trouble that I have is I did this for so long, I have so many different stories and different stuff that I actually can't pick one, there's so many.
But I can describe a couple different things. So when I first got into this, Berkeley Lab was just creating something that they call the mid-range computing project. And the mid-range computing project represented computational requirements. That was everything between somebody's desktop or laptop and NERSC, a giant user facility, a computing facility for all of the Department of Energy. There's a huge gap between NERSC and your desktop. And they saw that as what was called mid-range computing, and they defined it as mid-range computing. And we were initially funded to go and build 10 systems, 10 clusters, and the scientists, we had 10 different groups of scientists that said they have needs between the laptop and the desktop and NERSC. And it's funny because we've had this conversation before, which is, something like that happens, everybody should band together and buy one system and then share it. But that's not what early HPC was like. And so everybody wanted their own systems and every system needed to be configured differently, both in terms of hardware requirements as well as in terms of scheduler needs and software stack and applications, and we used to joke about this, every system was serial number one, because you're creating it from scratch pretty much for a particular use case. Like there was no cookie cutter-ing that you can do here. It was always, you're starting from ground zero with every system. So we had some users that were along the lines of, so I wrote this Python script that currently runs on my laptop and I don't like having to shut it down when I bring my laptop home.
And so I want it to run more, to geophysics applications, which were massively tightly coupled, massively parallel, and they can definitely run on NERSC, but NERSC just had a long queue weight and they wanted to iterate faster. And so you take this giant span of things that people are doing. And to Alan's point earlier, like the types of workflows are not always exactly the same. So we did one for a group that was doing some sort of gamma ray detection, and they have this big detector, it's this giant sphere. And around this sphere you have some sort of reaction occur. I think it was, don't quote me on this, but I think it was coming off of either a cyclotron or an accelerator. And they were actually doing a collision within this infrastructure and around this sphere were thousands of separate detectors and they detect what happened via the explosion by looking at the aftermath and seeing how all the particles dissipated through this.
But what you have is not a traditional HPC problem that you need to solve because you have thousands of detectors. Each one of these detectors is basically creating a stream of data that you need to pull into a bunch of different compute nodes and start trying to figure out what's happening there. So it's almost like you're doing parallel inferencing or something like that, right? It's a different sort of compute model. So we had everything from that, to we need to run our Python scripts more effectively and on a larger scale, to a geophysics application. I'll talk a little bit about that geophysics application, because that was really interesting. The goal was to do subterranean imaging and there's a number of ways to do that, right? I mean, you can't really take an x-ray of the earth, right?
It's kind of deep, so you can't get very far, right? So how do you actually figure out what is underneath the ground? And a long time ago, people realized when earthquakes occur you actually have the ability via detectors to start figuring out the different densities of materials and what kind of materials are there. So for example, liquids do not transmit S waves, but they definitely transmit pressure waves, right? So pressure waves, you can track through water, but S waves you can't. Just to articulate that, right? S waves go up and down so the water would just absorb it. The pressure waves are like sound, right? So it will emit pressure. And you can hear underwater, not effectively, but you could hear things underwater. So in an earthquake, there's no S wave damage.
But there is definitely pressure wave damage in water. So if you are going through a body of water that's underground, you get different sorts of wave propagation, you can also get different material propagation, right? So just like a lens refracts light, you can refract other sorts of waves through different densities of materials underground. So they started learning about this through earthquakes originally. But the goal is, of course, you can't reliably do subterranean imaging through HPC waiting for earthquakes. So they had to create little mini earthquakes and they did that via basically getting a whole bunch of very sensitive Richter scales, putting it around a large surface, and then digging a really deep hole and exploding a bunch of dynamite in that hole. And then you can see the different wave propagations, reflection of waves and whatnot through a three dimensional space that was occurring underground.
And you can take all that data coming off of all those Richter scales and assemble a 3D model of what is subterranean underground. And it gave people the ability to do everything from just understanding what kind of materials were there based on their densities of course, you couldn't validate, right? But you can make reasonable assertions based on the densities of what you're seeing. But you can also find really valuable things like oil. And so this was used quite a bit through oil and gas. So a lot of oil and gas organizations will use this to try to identify where there's large pits of oil.
Now, anyone who's ever dug for a well somewhere, I don't know if anyone's lived rural, but if you've ever had to dig a well, there's all sorts of ways to figuring out where is the subterranean water, right? Everything from shamans to the two sticks. There are seriously, magic people that have incredible track records of waving sticks and doing song and dance and whatnot. And I'm not being silly about this at all. Like, this is real. Like you can actually pay people to go figure out where you drill your well, and you can potentially save tens of thousands of dollars drilling your well based on the results of this. But if you wanted to go more scientific, there may be subterranean and imaging things that you can do. But with that being said, I don't recommend exploding dynamite underground. When you're trying to find drinking water, it may taint the drinking water. Yeah, it could be bad. Although it might also be bad to blow up an underground oil reserve, right?
Zane Hamilton:
Or a natural gas deposit, that could be fun.
Parallelization [30:33]
Gregory Kurtzer:
Or natural gas. But that was one of the first big HPC use cases that actually I came into. And I got really into that because to me it was very interesting because it was one of the perfect ways you can explain how does parallelization occur in high performance computing because there's multiple ways of thinking about parallelization. But in this particular case, you have a three-dimensional space. And one way of optimizing the compute is to say, well, I can just run this whole thing on one core and one memory space. Sure you can, right? It makes the algorithm real easy. But if you want to optimize that, you have to start breaking apart this three-dimensional cube. And what you do is you slice it into sections.
So you say, this section maybe is running on this core, on this system, this section's running on this core, on this system, et cetera, right? And so now you've broken apart this whole 3D model into cubes, into sections that's running across all these different nodes. Now this gets really tricky because each one of those 3D models, they're not isolated, right? You're going to have interaction with its neighbors. So as a seismic event or a wave propagates through the material and it goes from one section of one cube and it starts interacting with other sections of other cubes, you can think about that in terms of interprocess communication. So now the parallelization has to talk between processes in order to convey how the wave is propagating through various different substrated material. And so you get this really sort of very, very tightly coupled job, where the larger you scale, the more difficult the network problem becomes in terms of doing inter process communication.
So the gist of this would be do one timestamp, whatever that timestamp is, do a timestamp interaction across everything, and then everything has to stop, wait for everybody else to catch up and then transfer any sort of boundary conditions to the neighbors, do another iteration, stop, wait for everybody to catch up, do another. And so you can start to see, wait a second, this doesn't sound very efficient. And this is why the network is so incredibly important. So Alan, you mentioned network, I believe as well. I mean, the network is so critical on these high performance computing systems because the compute is waiting constantly on network. So you have to have something like an infini-band or some sort of very low latency, high performance network interconnect to be able to handle something like this.
And if you don't, you're going to get to the point where it's going to have a negative scaling effect, where if you look at the performance over how many cores or how many systems you're running on, you're going to start to see lower and lower performance. Like, it's not linear. It's not just going to keep going up and up and up, right? At some point it's going to be like, okay, okay, we're tapping out the network, we can't keep up with the network anymore. And that's literally because you have this notion that every job has to always wait for the slowest process in your entire, what's called the M P I universe. All of the processes running, you're always waiting for that slowest one. And it really gives you the understanding and appreciation for how to build systems at scale that are tightly coupled. Nobody does that better than HPC. In terms of building giant systems, though HPC isn't even on the map compared to cloud, right? Cloud is really giant, but they're not tightly coupled, right? They're very loosely coupled. And so to build those tightly coupled resources, in my mind, it's taken decades for us to perfect how to do that properly. And there's a lot of PhDs that have been earned on how to do this effectively and efficiently.
Innovative Uses of HPC [34:51]
Alan Sill:
Yeah. So maybe we should talk about what is holding back innovative uses of HPC? There are lots of cases where HPC works and it's a very healthy industry. Cloud, I think as a whole, I would agree with you, Greg, but certainly it's also grown the ability to access tightly clustered resources. I think Microsoft just brought out an Azure H 100 by 8 option which looks to be very well connected, so you can get at those things. So the hardware's there, to some degree the software is there, lots of work has gone on to make it easier. So what's holding back people from making innovative choices? And as much as I dissed ChatGPT a minute ago, I think one of my most persistent interests, and it is in music and enclosed form programming settings. Now, I don't trust code written by a large language model. Those things are statistical, but when you tightly constrain what it can do, you can have it do things like write API calls and so forth, even do some coding. The usual cautions apply that we've talked about in previous calls about trusting the output. There's no intelligence there, right? It's just these are statistical models. But is that an element? What is it that's holding back people from doing really clever things?
Gregory Kurtzer:
I've got an idea. So I believe it's our approach to high performance computing and what that infrastructure looks like. And of course, this shouldn't be a surprise to anybody on this call, as we've been working on that quite a bit. So, not to sound too biased here or plugging what we're working on. But I think that you bring up a great point. I've done, now numerous, talks and keynotes about the effectivity of the Beowulf and how well the Beowulf has met our needs for literally decades, three decades to be exact, in terms of an HPC architecture. But I do believe at this point we do need to be thinking about this much more carefully.
And looking at it from the workload diversity perspective and ease of use perspective, a presentation I just gave fairly recently at Stanford, I talk about what did the user guide look like to HPC back in 1993, right, when the Beowulf was forming, right? Well, you ssh into your interactive node, you download your source code, you download your data model, you compile everything, you optimize, you profile, you validate, you debug, you then understand the underlying architecture of the system, the file systems, the scheduling systems, the cube structure. And it's not only until then do you actually run your job, then once you've run your job, you now have to validate your results and then pull your results off to somewhere else that you can actually continue your research on, whether that be visualization or whatever.
Granted, 30 years ago that was the way to do it. That was awesome, but how has that changed up until today? It hasn't. Like, we're still expecting the exact same from our users, and a lot of workflows, and a lot of jobs don't run exactly the same way. It doesn't necessarily want to run through a queuing system. And it may not be able to run on a traditional file system like what we're talking about, or it may be dependent on services that are running in conjunction with that or data streams. There's a lot of different aspects to this architecture, which the Beowulf again, has been incredibly important in terms of us creating and us running science and furthering science. But is it what we need today? I'm at the point where actually, I think we need to do better. I think that it's holding us back.
Maximizing Research Output [39:33]
Alan Sill:
Well, so in my own narrow setting, which is the only thing I can really talk about without just pontificating, and I'll try not to do that even in that setting. I have the job of maximizing the research output at my university, that's the way I view it. I tell people that my budget is an unimportant, intermediate portion of the calculation, that I can optimize how we do it. But ultimately, and we've talked about this again in previous calls, that the value that I see is in just as you say, reaching a larger fraction of the research community. And this is an old story. We've been trying to make it easier to use to some degree, we've succeeded and then have to deal with the consequences of that success. If you use cloud methods, you could spin up a cluster in minutes but then you get the bill.
So there's a definite optimization in multiple dimensions of access and productivity and efficiency and I like tools like open on demand from Ohio State University that lets you access an HPC cluster through a web interface and hit a button and get a Jupyter Notebook and stuff like that. Those have been around for a few years, and they help. So what's holding me back from pushing in this direction, it's largely budget. I go to the CIO and the provost and so forth, and I say, look, I could serve a larger fraction of the community, and it's not a power thing here. I'm not interested in building an empire. I just want to serve a larger fraction of the community. But I will have to hire people who can bridge that gap and who can and hear a story that I really like to tell, and forgive me if I've told it before, but it has to do with a different university, UC Berkeley that had a hundred people on its computing staff but was getting grief from the deans of the non-engineering, non hard sciences type schools.
And so they decided to just hire people with computing backgrounds, but who were artists and literature specialists and so forth, who knew computing, but their primary expertise was in all these other fields. And they found that this had an enormously positive effect on the number of users, the diversity in every measurable parameter in very positive ways. The number of jobs run, the research supported. So I think to some degree, you just have to take the challenge on. But it is a budgetary problem. And then coupled with that, there's the problem of finding those people and paying them the lower rates we can afford in academia to do these fantastic jobs. It's a very multidimensional problem.
Gregory Kurtzer:
So, Alan, I'm going to do another, and I'm really sorry, I'm going to do another shameful plug. We've actually been working with universities recently that have had the exact same experience, both in terms of a budget perspective as well as a personnel perspective. And we've actually been helping them to integrate a traditional, not even a newer version of HPC, like I was talking about, but that traditional HPC architecture. And what we're finding is that's a real problem that you're describing and we're seeing it first hand because in our mind, we're lucky to be able to help. It's not what we originally came out to market to do, but we saw that this was a pain point for a number of organizations. And Zane has been part of this, Jonathan's been part of this, Brian's been part of this for us.
Forrest, you may have been part of this actually as well too, but this was something that we made a decision on, like, how can we help these organizations and these academic universities with this problem? And to the point about Berkeley, I was joint appointment with Gary, who's a lot of time on our call. Gary and I were both joint appointments to UC Berkeley to try to help them with that computational side. And I know exactly what you're talking about. Yeah, very interesting.
Making HPC Easier to Deploy [44:15]
Alan Sill:
So you're saying one way to make HPC more successful at innovative uses is to just make HPC easier to deploy, just straight HPC, nevermind making it easier or fancier, giving it nice front ends, just make it easier to deploy at more institutions. I think that's true. I think the number of institutions that have HPC is a small fraction of what it could be. And it is because it's a hard job.
Johnathan Anderson:
Yeah. The little bit of nuance that I've put on that is like, HPC is hard. It's complex, and it's not hard when you've been doing it a long time. The parts are explicable. They make sense, but there's a lot you have to get over that barrier to entry. And when you're building up an HPC center at an institution, there's a tendency to have the staff be increasingly narrow focused on HPC. And the more you do that, the less staffing budget you likely have for the kinds of people that you were referring to, where you bring in people whose primary expertise is something outside of that. And so what I would hope we could help a department do is fill in some of that really narrow specific HPC focused expertise and free them up to have the people that are local and onsite be more focused on the local site research or academics that they want to use that system for.
User Consultants
Gregory Kurtzer:
And Alan, I think you also were alluding to the user side of this as well. So it's even more than just the system administrators in terms of building the systems. It's the user consultants, right? The scientific consultants that are helping with the application stack, helping and working directly with the scientists to ensure that, again, we're not just talking about physics, chemistry and the traditional HPC use cases at Berkeley. I mean, you're exactly right. Again, there was library science who wanted access to the cluster, political science, which wanted access to the cluster. I mean, I never thought when I first got into HPC, I'd be talking to librarians about how to do HPC or political science. Political science I can actually see a little bit more, right? As you're doing modeling of the political landscape and whatnot. But library science, I never would've guessed in a million years.
Zane Hamilton:
Did they ever tell you their use case, Greg? Like, what were they doing with it?
Gregory Kurtzer:
Actually, I don't recall now.
Alan Sill:
Library well, so there's always a little bit of tension within any institution between the librarians and the IT folks. At least that's been my experience. I, I've always gotten along really well with them, but they want to support things that sometimes use computing, like, let's say, making movies. And I often tell that story that, try to find me an academic field that doesn't make use of computing, or at least data in large quantities these days. Every field does. And so the example I often use is making a movie. Unless you're making an eight millimeter art film, you're using computers. So often, the goal is not computing for its own sake, but because you need to do things. We had some folks that came to us through the library from the architecture department that were running around with lidar scanners, doing things like mapping the Statue of Liberty and so forth. And so those things require deploying resources at a scale that the library wasn't used to. So we worked together on that. Yeah, I think that the spirit of just making the HPC simpler to deploy and then let people take it from there, that's probably a good rubric here. The innovation will come when people can access things. And let's face it, the laptop I'm doing this on would've been a super computer not long ago.
Gregory Kurtzer:
I agree with everything you say, but I would push back a little bit in terms of the traditional HPC model is not an easier barrier for many new researchers and scientists to come on. And I think as a result, we're limiting some of the effectiveness of having a large amount of hardware that's designed to run tightly coupled and GPU and accelerated type of tasks. And if we don't figure out a way of, across the board, making this easier to leverage, like Open On Demand has, Jupyter Notebooks have definitely done that, but even if you look at Open On Demand, it's still doing all the same steps that I said. It's just providing you a graphical interface for it. What I'd love to see is absolutely lowering the barrier to where we can open up HPC to people who do not know anything about the systems architecture or the computing architecture and make it so it really is a Kubernetes like workflow, right? It's so simple that people can just define it by dragging blocks around in a visual graphical environment.
High Frequency Trading & Quantitative Finance [49:56]
Zane Hamilton:
So I do have one other use case. I'm going to bring it up and I'm curious if any of you have ever experienced or had experience with it, and I know it's relevant today, and maybe you can all tell me that's not a good topic, but I know there are a lot of financial analysis being done on HPC, which seems like a pretty relevant topic for what's been going on this last week. Have any of you ever worked with any of those or seen any of those and what they do, how they do, what they, maybe we should do more of it?
Gregory Kurtzer:
So high frequency trading is an inter... I don't mean to cut anyone off. I saw a couple of people come off mute. Do you want to jump in? Okay, Forrest, go for it.
Forrest Burt:
I was just saying go on ahead, Greg. I've never dealt with high frequency trading or the quantitative finance side of HPC myself. But I know that like backtesting stock strategies and all that type of thing is a fairly extensive computational process, especially from a numerical analysis standpoint. Taking a hundred years worth of stock market data every day, opening, closing prices, intraday prices, all that stuff. And being able to run mass analysis on that is a huge task. Doing mass options, pricing, black SHOs equations, all this quantitative finance stuff is ultimately pretty advanced math under the hood. So it makes sense that HPC is ultimately the class of resources that they end up drawing on to do their day-to-day.
I'm not sure how much of their like day-to-day, like the technical side of HFT operations are done with an HPC cluster and we're talking about like the analytical computational side of it. Because I know that HFT systems are very high class systems that are meant to provide very, very fast network connectivity back to exchanges and stuff like that. So those in and of themselves might be HPC class resources, but I know certainly at least it's easy to see other research itself and quantitative finance generalize as well to HPC resources.
Zane Hamilton:
Yeah, those systems are typically physically placed directly next to the exchanges from the network perspective. So that's interesting.
Forrest Burt:
Downtown New York or something like that?
Zane Hamilton:
There's a few around there that are very bizarre nondescript buildings that don't fit in. It's pretty easy to tell which ones they are. Yes, they're interesting to enter.
Alan Sill:
Yeah, I don't have any direct experience, but indirectly I've heard from people, even with some of the most recent, high speed fabric innovations, things like the rock board, all fiber connectivity that for some of the first applications of that have been in the financial sector.
Zane Hamilton:
Go ahead Greg. I know you had something to say.
Predictive Analysis [52:51]
Gregory Kurtzer:
I always have something to say, but I think Forrest nailed it, right? There's different ways of looking at it, but at the end of the day, I mean, if you're doing predictive analysis, it's just a data problem. I mean, you're just looking at a massive ton of data and you're computing and you're trying to look for trends and whatnot. That's a computing model. Just like almost every other computing model that we're talking about, very high level generally, right? And a traditional HPC system would do that perfectly. But what I will say is in high performance computing, and I've said this a bunch of times, I hope I haven't said this before on this webinar, and if I have, sorry everybody, but in traditional high performance computing, we have been wearing blinders for literally decades not caring what anyone else in the ecosystem's been doing.
And generally, I mean, that's been totally cool because it hasn't affected us. They're running, they're doing different things. What we're starting to see, and I saw this directly with the advent of containers in high performance computing because it caused me to sit on this fence between the traditional HPC side and what enterprise ecosystem and cloud and hyperscale and et cetera is doing. And it gave me a really different vantage point that we need to do better than just traditional HPC is today, otherwise the enterprise is going to reinvent wheels and we're already starting to see that. And we've got 30 plus years of experience figuring out how to optimize this and do this extremely well on the computing side. But if we don't modernize our architecture, that's going to be that's not going to be effectively utilized throughout enterprise and the rest of the ecosystem.
And I'll stop talking with a quote, which is, as I was describing this to an enterprise organization that was solving a very HPC-like problem and I described the architecture of an HPC system, their response was, "we're trying to get our system administrators to stop using SSH at this point, you're telling me you want our users to use SSH? Next you're going to tell me you want our scripts to use SSH in a passwordless way to automatically deploy workloads too." I mean, it really does demonstrate how backwards in terms of innovation that HPC as an architecture has been, well, the use cases and applications for HPC are in the forefront of innovation as well as the hardware forefront of innovation. But that architecture, again, sorry to keep harping on that, but if we're going to continue to innovate and expect innovation of HPC applications and HPC use cases, I believe wholeheartedly that we have to also innovate the system architecture that we're using to deploy these sorts of workflows.
Zane Hamilton:
And I think that actually answers David's question a little bit. So do you believe that approaches to problems are designed to fit into the additional HPC model rather than seeing HPC morph to work on challenges in their native state? I think that's kind of what you're alluding to, Greg, is we're going backwards.
Innovation [56:15]
Gregory Kurtzer:
We're forcing the applications to still fit that traditional HPC model and not look towards the future and really further innovate. I would love to see more innovation and more workload diversity just continue to increase. I would love to see in architecture such that enterprise organizations don't feel as though that they have to go and recapitulate a 30 year old architecture in order to do their AI training and their ML training and compute and data driven analytics. I'd love to be able to hook into everything from CICD pipelines to DevOps integrate automated workflows, automated build operations, get operations, all of that into HPC.
Alan Sill:
Yeah, I think we can take a hint from networking and let's say specifically telecommunications. The degree to which complexity has been simply covered over in the telecommunications industry is astonishing. Behind your cell phone is the same logical architecture as when individual companies ran individual wires and you could only talk to the subscribers that were physically connected to those wires. That level of complexity has not been gotten away from by architecture. It's actually simply been layered over. And so rather than reinvent HPC architecture, which was largely designed for scaling, we might end up layering it in ways that make it invisible to users.
Gregory Kurtzer:
Alan, as part of a presentation I gave recently, I went back and reviewed the top 500 all the way back since the very beginning. And it occurred to me there were a couple major, major breakthroughs. So ASCI Red was the first major breakthrough on the top 500 in terms of surpassing the teraflop barrier. And then Roadrunner beat the a pedo flop barrier. And then Frontier, as an exiflop, but going back to ASCI Red, that was a teraflop system. Our cell phones of today, right? That was 40 plus million dollars for ASCI Red. I mean, it was a while ago, right? It was in the late nineties, mid-ish nineties, mid-ish to late nineties. But our cell phones are twice as powerful as ASCI Red was.
Zane Hamilton:
It's hard to believe.
Forrest Burt:
It's hard to believe. Really quickly, I just want to say it blows my mind that like 10, 15 years ago we were on like 10 gigabit ethernet for our networking capabilities, but now just 10, 15 years later we're starting to look at four 800 gigabit or better. I just wanted to mention that since we've mentioned networks, it's the networking side of things. How far we've come there, is very impressive as well.
Gregory Kurtzer:
But how has latency been affected with that? Like is latency coming down at the same rate? Generally, no. At least not in my experience.
Alan Sill:
Well, I mean, 400 gig infinite band is routine and that's with low latency. But what I don't see any progress on is getting it into my house at any sort of reasonable price.
Zane Hamilton:
Course not. Well guys, I really appreciate it. We're up on time. Thank you for joining. Thank you for the calls. Those of you who are on my list, you know who you are. I will be coming after you shortly. I appreciate it, Alan, thank you very much. Enjoy the rest of your spring break, guys. I'll be seeing you later. Some of you sooner than others.
Gregory Kurtzer:
Take care. Thanks, Zane.
Built for Scale. Chosen by the World’s Best.
1.4M+
Rocky Linux instances
Being used world wide
90%
Of fortune 100 companies
Use CIQ supported technologies
250k
Avg. monthly downloads
Rocky Linux