Webinar
HPC by Industry: Bio
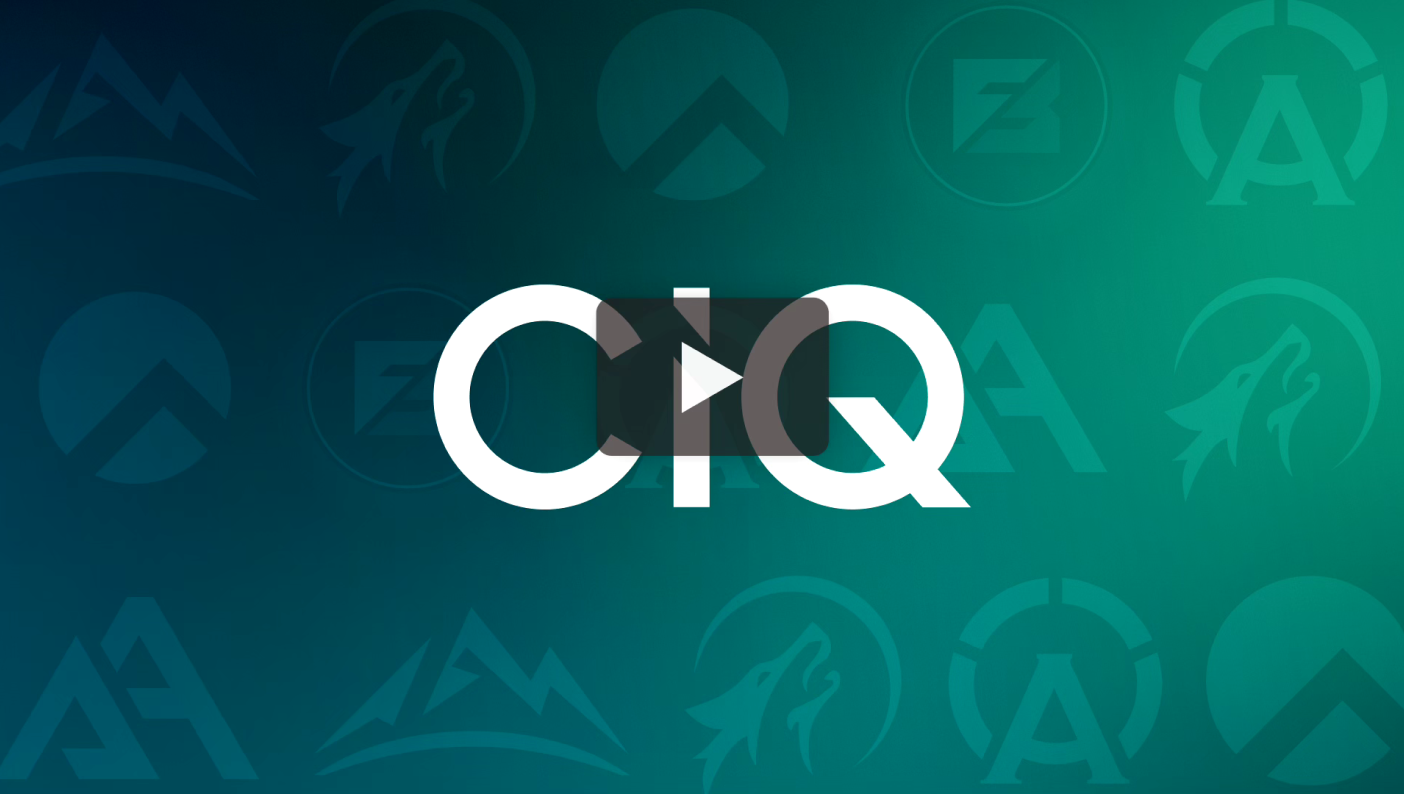
Next, our Research Computing Roundtable will discuss Bio in the HPC industry. Our panelists bring a wealth of knowledge and are happy to answer your questions during the live stream.
Webinar Synopsis:
Speakers:
-
Zane Hamilton, Vice President of Sales Engineering, CIQ
-
Forrest Burt, High Performance Computing Systems Engineer, CIQ
-
Jonathon Anderson, HPC System Engineer Sr., CIQ
-
Glen Otero, VP of Scientific Computing, CIQ
-
Brock Taylor, VP of HPC & Strategic Partners, CIQ
-
Gary Jung, HPC General Manager, LBNL and UC Berkeley
-
John Hanks, HPC Principal Engineer, Chan Zuckerberg Biohub
-
Alan Sill, Managing Director, High Performance Computing Center, TTU
Note: This transcript was created using speech recognition software. While it has been reviewed by human transcribers, it may contain errors.
Full Webinar Transcript:
Zane Hamilton:
Good morning, good afternoon, good evening, wherever you are. Welcome to another webinar. My name is Zane Hamilton. I'm the Vice President of Sales Engineering here at CIQ. For those unfamiliar with CIQ, we're a company focused on powering the next generation of software infrastructure, leveraging the capabilities of the cloud, hyperscale, and HPC. From research to the enterprise, our customers rely on us for the ultimate Rocky Linux, Warewulf, Apptainer support, and escalation. We provide deep development capabilities and solutions, all delivered in the collaborative spirit of open source.
Today we're going to have an HPC panel. We will be talking about HPC specific industries, and today we will be talking about bioscience. We bring everybody in, that would be great. Nice to meet you, everybody. Welcome back, Alan. Let's do interviews real quick. Gary, I'm going to start with you since you're next to me. Can you introduce yourself again?
Gary Jung:
Hi, my name is Gary Jung. I'm the scientific computing group lead at Berkeley Lab. My group manages the institutional HPC for Lawrence Berkeley National Laboratory and runs the HPC program for UC Berkeley.
Zane Hamilton:
Thank you. Dr. Slli, can you hear me? Alan, can you hear me? We'll come back to you, Alan. Glen?
Glen Otero:
Hi, I'm Glen Otero. I'm the director of Scientific Computing at CIQ and biosciences.
Zane Hamilton:
Excellent. You're going to be doing all the talking today. I'm glad to hear it.
Glen Otero:
I hope not. I'm going to give that to Brock.
Zane Hamilton:
Absolutely. I see John jump up in the corner. Let's go to John.
John Hanks:
Hi, I'm John Hanks. I'm Assistant Manager at CZBioHub, and bio stuff is about all we do.
Zane Hamilton:
Brock, I can't remember if you've been on before. Brock, have you?
Brock Taylor:
Longtime listener and first-time caller. Brock Taylor, Vice President of High Performance Computing and Strategic Partnerships. Since it is my first time as a longtime HPCer or specifically on the systems side solutions, architecture, building, and running clusters working end-to-end hardware and software. I'm happy to be here. If nothing else, make fun of Glen during any downtime of the round table.
Zane Hamilton:
Everybody enjoys that.
Brock Taylor:
Yeah.
Zane Hamilton:
Go to Jonathon. Welcome back.
Jonathon Anderson:
Thanks, Zane. Jonathon Anderson, I'm an HPC Systems Engineer with CIQ.
Zane Hamilton:
Forrest, back from the new office. Excellent. In the new office.
Forrest Burt:
Indeed. Yes. Enjoying it quite a bit out here. Hi everyone. I'm Forrest Burt, I'm an HPC Systems Engineer here at CIQ.
Zane Hamilton:
Excellent. How about now, Alan?
Alan Sill:
I'm the Managing Director of the High Performance Computing Center at Texas Tech University. One of several co-directors of the multi-university National Science Foundation's Cloud and Autonomic Computing Industry, University Cooperative Research Center, which is a mouthful.
HPC in the Field of Bioscience [03:42]
Zane Hamilton:
Thank you. If you guys have questions, go ahead and post those in the chat. We're going to start bioscience, which is a very broad field. There are some key areas that HPC plays into that. What do you guys see as those types of fields, and what do those applications look like? I will pick on Glen first because he is still mute in the middle. Just let's wait. All right, Brock, go ahead.
Brock Taylor:
Well, it's very broad drug design is one area I would say it's molecular dynamics in drug design. If he could say it, Glen would probably jump in with genomics or bioinformatics. Then parts of what I would love to talk about today, too. There's a lot of crossover into things like simulation and mechanical engineering. Medical devices and things must go through similar simulations and testing to be incorporated into the medical field. I saw a presentation a couple of months back. I want to say something different from what it was. Still, it was a device implanted into the body, which needs a very defined lifetime of operation and durability because if it fails, it could potentially be fatal to the patient. Simulations and things are going on. That's a crossover that's not necessarily thought of as biosciences but related to the biosciences field. High performance computing is very key to some of those tests.
Zane Hamilton:
In a minute, Brock, I'll come back and ask you a question specifically about that. But now that Glen appears to be off mute, if we can hear him is a whole different story.
Glen Otero:
Brock got us started well. Molecular dynamics come into play in basic research on how proteins fold and, particularly in drug design, how drugs dock to different protein receptors. What I've spent most of my time on in the space, Brock also mentioned, is bioinformatics. That is involved in a couple of different fields. One is like predictive health analytics. You take bioinformatics genomic data and some electronic health records. You try to do some modeling and predict how often someone or the chances someone would have to come back into the ER with sepsis after treatment, for example. Precision medicine is the kind where bioinformatics and genomics is kind of pointed to.
Suppose someone were to have been diagnosed with cancer, and we were able to sequence their genome and compare it to their normal somatic cells. In that case, we could pick out those mutations in the tumor and pick drugs that would specifically work for that patient's mutations. Their background and not rely on just any old clinical trial that's been done in the past. Then, of course, AI with regard to, let's talk about the genome for a second. If I want to take all the human genomes, I can get my hands on, for example, and I want to look for how DNA replication starts. What sites do they start at? Well, I know the basic pattern, but I want to expand on that so I can build models and train with all sorts of data, looking for new sites for DNA replication or different types of sites and things like that.
We can learn things about the genome as well. Last but not least, modeling disease spread like pandemics, for example. A lot of that has been done with recent or current. I should say, the Covid pandemic. It's being done right now for the outbreak of Ebola in Uganda. It happens every time there's an outbreak of things like that. Those simulations take in massive amounts of data. You've got an environment. You've got traffic, you've got weather patterns, you've got air traffic, you've got other geological, environmental factors to be built in. It's not necessarily just a computing problem; genomics and modeling of these pandemics are massive data problems.
Zane Hamilton:
Excellent. John, this is a place where you spend most of your time.
John Hanks:
You've covered the wide gamut of stuff that we do in bioscience. I could only add to that when you think of the word bioscience, biophysics, biochemistry biogeology. In my view, and it's clouded by having a couple of biology degrees, it is the most important science, period. Bio is a prefixed bio that gives us a context and a reason for doing all that other science.
Zane Hamilton:
Thank you. Gary, what are you seeing here?
Gary Jung:
Let me add you to the list. We have a lot of large instruments in the laboratory. Some areas require imaging that requires the HPC systems to reconstruct the image. Some of the pipelines I'm thinking of are cryo-EM and tychography.
Zane Hamilton:
Thank you. Dr. Sill?
Alan Sill:
Only my mother calls me that.
Zane Hamilton:
But it's fun. I don't call you that on purpose.
Alan Sill:
There's a huge range of things already covered in the comments, and I agree. I can't resist pushing back on our Chan Zuckerberg colleague here. I will immediately demolish an old meme for physicists. I'm a particle physicist by background. Lord Kelvin once said that all signs are either physics or stamp collecting. But I'll immediately demolish that because that was maybe true when I was a high school student. But since then, the information science of biology has come along. That's what we are talking about here, and the light years of progress in just a few years of work are astonishing. What I want to add to the previous comments is how we approach biology topics as in the information sciences.
Even there, a huge range of tools dwarfs anything available even just a decade ago. We've discussed many of these before on this broadcast, everything from in browser apps where you can run Python or Jupyter Notebooks to things that used to require a whole cluster to do that you can do without leaving your browser even holy cow. Up to things that occupy the biggest clusters. Beyond single machines or clusters, there are the collaborative aspects of using technology in biology. A few years ago, the NIH started a cloud project. If you've heard previous broadcasts, we've discussed the economics and the pluses and minuses of cloud versus on-premises and why many big scientific outfits still run on-premises resources before. But NIH did something which required the cloud to resolve, which they shared among biological sciences, practical and efficient. There are all these dimensions in addition to the science itself. I'll stop there.
Zane Hamilton:
No, it's great. I will go back to Glen in just a minute. There's one thing that keeps coming up. Jonathon and Forrest, do you have anything that we've missed?
Jonathon Anderson:
I couldn't find something that's been missed by this so far.
Brock Taylor:
I actually want to jump back in. Well, Forrest, you go on if you have some.
Forrest Burt:
HPC is huge in biological sciences, and we're seeing amazing advancements powered by HPC, especially around AI and stuff in the field. I was quick; I didn't hear anyone say it by name, but some of the advances that HPC and Biosciences have powered are like alpha fold, which took huge amounts of data about protein folding and could predict then. I've always heard decades' worth of protein folding research within just the matter of weeks that it took to utilize the AI model to generate all of it. But I didn't hear anyone say that use case specifically, but I wanted to mention that.
Zane Hamilton:
Thank you, Forrest.
Brock Taylor:
That's something that Alan said that made me remember drug trials today, that the FDA still mandates human trials. You have to have those trials. We're moving to an age where we've got data now where you can simulate populations of people, which can speed up drug testing. It also can reduce the points where you have to do things like double blinds, where some people who drugs could help don't get it because you've got to see the impact. There's much to unpack when discussing using simulation for FDA approvals. Do you trust that the simulation output is correct? The ethical element is the models trained for enough populations.
We've got to consider many different things in terms of using that data and that technology. AI, HPC, and the underlying computational technologies, I think, have a way that can help impact the health of the planet and people worldwide. Still, it can change the game of getting targeted drugs to market faster than, again, you look at the Covid 19 vaccines and the short amount of time we have, and you have to make these tough calls. Do you push a drug out there without full knowledge of what it could do?
Gap Between Practitioners and Biological Sciences and the Computing Technologies Required [15:17]
Alan Sill:
Can I ask a question about a gap that I think we still need to eliminate: the gap between many practitioners and biological sciences and the computing technologies required? Many researchers who use our systems just self-admittedly say they need to figure out how to get started, whereas others are sophisticated. I'm sure you've seen this.
Zane Hamilton:
I figured Brock would have an opinion on that one, but Gary is something we've touched on before. Is that the case from what you're seeing? Are there people who are good at it and those who are bad at it in the middle that seem to not be in the middle?
Gary Jung:
This is just a generalization, so it doesn't apply to everybody. Still, as a whole, people in life sciences have used computation more recently than some of the other scientific disciplines. I see it as a new generation of researchers who don't want to understand some of the underlying technology and say code. That's becoming something that I'm seeing. The last thing, an implementation barrier, is that when researchers are working with sensitive data, securing a server or their workstation to work on some of this very sensitive data is one thing. But then, to move it to something that requires high performance computing means that you have to apply those security controls to a research cluster, which is a huge undertaking. For many institutions, that's a big step that's hard to implement because many cybersecurity experts have yet to have the experience of applying the controls to what we do every day with clusters and workflows and stuff.
Zane Hamilton:
Thank you, Gary.
John Hanks:
When we were talking about this, I was thinking about something that happened to me as an undergraduate. I was sitting outside a dean's office, waiting my turn to talk to an advisor. Sitting in the advisor's office, the person ahead of me said I like science, but I'm not good at math. The advisor, without any hesitation, said, oh, biology, you should go into biology. I cringed then, and I cringe now. But many of the problems we face with educating users on how to use these systems stem from that attitude towards biology that is still prevalent in the educational system. People who go into biology think they're not going to be doing math, and they think they're not going to be doing computing. That's not true anymore. You only do biology now with doing math computing. There are a few people out there who maybe go out in the field and describe new species or mating habits of a beetle or something, but as a biologist goes, those are becoming rare. The biology coursework across the educational system has not caught up to that.
Zane Hamilton:
That's interesting. And I've never thought about that before, but now it makes me wonder if Glen's a mathlete. And I didn't know it.
Glen Otero:
John makes a good point because there were two tracks at the University of Illinois. There was one for the engineers and one for the biologists. I had my track of calculus and whatever came after calculus. I didn't get into differential equations, but the engineers had a different physical chemistry course versus mine. Mine dealt with lipids and micelle and stuff like that. The engineers' course dealt with just surfaces and materials. There was a clear difference, but we were all using pencil and paper. But now, like John said, at minimum, you're using Excel and a laptop in the field.
But you still get all sorts of other data if you're studying your frogs or fungus on frogs. You've got data from people coming worldwide that you have to compare it to. It would help if you understood the statistics being run into your spreadsheet, but now you want to compare it and normalize it to different data sets worldwide. You no longer have that option to understand the math you're working with. Gary, I've been forced to grudgingly accept that biologists don't want to understand what's happening behind the scenes in their pipeline and just want browsers. Because I thought that once we started minting bioinformatics with actual bioinformatics degrees, some of the first places were Santa Cruz and Stanford, which would catch on, and more people would have that training.
But it's just turned out to be the opposite. Things like the Galaxy and Jupyter Notebook are just rampant, which is fine as a tool, but they do not want to grasp what's behind it if you're making a diagnostic call on a tumor. Like, I have a real problem with that. I've come to grips that like that's going to continue, and I'm still, I'm still fighting against it. But that's actually. I dealt with a lot of that at TGen. It's like the bioinformaticians knew what was up and created a completely different set of problems on the cluster. The other users just helped them figure out how to use a cluster. Everything seemed to fall in those two cams.
Zane Hamilton:
Brock, I know you've been waiting.
Brock Taylor:
You still have to understand the science behind what you're doing, and a simulation or a model can spit out a number. But if you need to know what that number means, not only can it be just wrong, but it can be dangerous. That's that element. You can be confident that you've got an answer, but is it credible? Do you have an answer that makes sense and will make an impact? In the manufacturing world, you're going to say you would design a product that can't be designed right or wouldn't work. It's a huge problem, and on top of it, we're compounding the problem because the technology underneath to do the computation is rapidly diverging.
It's no longer a CPU world. It's a CPU plus GPU world, and now there are FPGAs, different types of accelerators, and different things coming online rapidly. There are all kinds of different performance libraries and utilities and things that all need to be coming together. It's just getting more and more complex. Eventually, you get out to a user base that's already opposed to having to learn a lot of things. They turn them off completely. People move away from using the simulation. Combating that abstraction of complexity and making it consumable is a key industry element with which the HPC crowd needs to do a better job.
Alan Sill:
I'd agree with you. Beyond that, it illustrates, so we've talked about the user familiarity, but also the code that's out there is often, as you say, not optimized for current generations of computers. I have single-threaded things and users pounding on me to let them run two weeks in a row, which I keep telling them is a bad idea for many reasons. I can only let this go by mentioning that the NSF, CSSI proposal, for those of you who are in the field of computer science software infrastructure, there are plenty of opportunities for people to write proposals and get involved with making the code better. I would be delighted if you did. That's not due till all of December. You have plenty of time.
Zane Hamilton:
Alan, do you have a link to that we could post?
Alan Sill:
I'll find one. Yeah.
Students' Desire to Learn HPC [24:45]
Zane Hamilton:
Thanks. That'd be great. Now I'm going over to Forrest and asking since you are probably the closest to this from an educational standpoint of the most recent coming out of education. I know that you spent a lot of time around this. You're passionate about education and how people come through this field. What did you see going through school and then as an admin from people's desire or wants to learn this stuff?
Forrest Burt:
Interestingly, some of the earliest people I interacted with were interested in automation and reproducibility and containers, and stuff like that were people within the bioscience. I believe specifically in the bioinformatics sphere. I worked a lot with a lab at Boise State that was very interested in modernizing its HPC efforts. They were very interested in things like containerization and the next flow. People at a Ph.D. level are interested in creating these systems. They are interested in seeing what can be done in this sphere of computing to improve the operations for themselves and the people working in their lab.
Some of the first people I ran into were interested in taking like conda and figuring out how to convert their conda environments into containers they could deploy in biosciences. In general, I see a lot of the push toward abstracting this. The overlap comes from the fact that, much like AI, this is a science domain that overlaps another field that people build careers around. It's difficult but as inefficient as it would be to have people in HPC trying to learn all these domain sciences to help optimize, it's inefficient to have domain scientists trying to learn all these different computing things.
Unfortunately, there are side effects to that. An example is the different ways that Python installs packages. We create all these different paradigms over time when we leave a lot of this computing effort to the domain scientists. It's important that, as I said, there are people at all levels of the experience. Some people are looking for reproducibility. Some people are looking for containers, and others are looking for Jupyter Notebooks. We must make sure that we're doing all we can as HPC people to apply our knowledge to these domain science problems. In a way that optimizes for the domain scientists not having to spend tons of their time learning these specifics of computing. We would want to avoid learning the specifics of all their fields.
Where Does the Data Issue Come From? [27:45]
Zane Hamilton:
It does. Thank you. One of the things I keep hearing that Glen started out talking about, so I'm going to pick on him first, but it's going to be interesting to hear John, Gary, your take on this. You said earlier that bioscience has a big data problem, so there's a data issue. Where does that come from?
Glen Otero:
A couple of different places. Real quick, though, to piggyback on what Forrest was saying, and I believe Alan brought this up at a prior webinar, the rise in the advent of the research software engineer is exactly due to what Gary and Forrest just said. There's this gap between researchers who want to do research and researchers who know how to code. Or engineers that know coding. Now we're getting more people like mathematicians that will step into that role and say, okay, biologists, tell me your problem, and I'll code it for you. But you can go look that up at the other prior webinar. But they're forming unions now. They have large online organizations and meetings in the UK or the US.
That'll continue to happen. DNA sequencers are a major one that used to be the biggest data creator in space. If you run a human genome, an aluminum sequencer now, like the raw data that comes out as a terabyte, and you'll end up with, after variant calling, maybe it could be like a half a terabyte BAM file or VCF file, a couple of hundred gigabytes. If you want to study a thousand people, it gets really large. But what's actually because I will be creating more data in the long run. Gary mentioned the cryoEM studies turn out multiple terabytes per experiment in data. There's a lot of imaging out there that a lot of artificial intelligence has been applied to. Whether its X-rays or MRIs or CT scans and other things, we're giving all that data to train AI on picking out broken bones or Alzheimer's or different types of cancer from biopsies. That's the majority of it. There are others with whom I hope others will chime in.
Zane Hamilton:
Yeah, Gary.
Gary Jung:
Well I think Glen did a pretty good job. I don't think I have anything to add to that.
Glen Otero:
The hospital records themselves. I forgot about that one.
Gary Jung:
Yeah.
Zane Hamilton:
John, I've got to believe this is a problem that you have to deal with.
John Hanks:
It's a huge problem. I mean, huge, no pun intended. The shift for me has been from when we were fresh on the heels of the Human Genome Project, and in my head, at least, I was thinking, when you're going to store a genome, you're going to store a human genome. For all of us, there will be one human genome stored. That's the storage problem. That is so naive and stupid. It's ridiculous because your genome changes over time and throughout your body like you have many genomes roaming about your body right now. The problem is beyond exponential in how much data there is to store, and that's before you consider that you're not just your genome. You're your genome plus the genome of every microbe living on your skin and in your intestine.
As an organism, the problem grows and grows and grows. Now you want to do a longitudinal study, collect that genome every month for 10 years, and see how things change over time, how the microbiome changes over time so that the storage problem just in genomics is enormous. It's only going to get worse. People don't want to stick this stuff in a deep archive and never look at it again and have it just in case they want it online so that the next upgrade to blast comes around. I can rerun all my pipelines, start to finish, on all my old data. You don't just have a data storage problem. You have to keep data online where I can get to it and rerun an analysis problem. That's just genomics has thrown on top of that the fact that every year, the amount of pixels a CCD can capture goes up, and people put them on faster and faster microscopes and want to catch live streams now, not just individual images. Post-process those with GPUs and AI, whatever. We never get on top of the storage problem coming down the line.
Glen Otero:
One thing I should have mentioned is that proteomics will have a lot more data. It's similar to what John was talking about. Now you want to look at the metabolism in your blood in a person's blood over time during, with, and without treatment. Then you have to compare that to a population. It just feels out of control. What also needs to be improved is I've been a big proponent of compression for genomic data. We could store more in a smaller space, but the storage vendors want something else. I would bring up compression, then they're like, shut up, go away. You're killing my sales. I was like, don't you think the customer would want us to store twice as much data on your storage than you're letting them on about, and then they would keep buying your storage? The incentives there were just completely at odds with each other. It is a real problem, and the cloud was meant to deal with it in some ways. You could put out this data that everybody could share, but as John said, it's like, the amount of it, and the types of it are just daunting.
Size of Storage Needed [34:09]
Zane Hamilton:
I guess I thought about it, like you said, John, from the genome perspective, but if you're taking an individual from the human genome, what size are we talking about? What size for a person are we talking about at one point?
John Hanks:
That the size will depend on the sequencing technology used and how much of the original data you want to keep. If somebody way back 10 years ago, you may have had people who didn't just want to keep the sequence data. They wanted to keep the images from the sequencer because the sequencing technology was changing. They can reduce errors by reprocessing the original images. In those cases, you're talking about multiple terabytes of data you would capture from the instrument. If you want the raw FASTA files, those compress well, and you wind up with megabytes to low gigabytes for a person's sequence data. It depends on how much of what the instrument captures somebody wants to keep to reprocess later or if they wish to keep the FASTA. To get an idea of how big things are, you can look at NCBI or any reference databases because they store the actual sequence.
For people that have instruments and are collecting data off the instruments, a single run can be in the terabyte, two terabyte range for an organism like a human. That'll depend on the coverage that they do. Do they do 50x coverage or 10x coverage or whatever? Any multiple of the number of times they want to run a particular set of sequences. It's variable and depends on what the people want to do with the product downstream.
Alan Sill:
My favorite definition of big data is in the language. To answer your question, I can't remember who said this, but I've quoted it ever since. Big data is everything we used to throw away. The receipts are all the videos from how long you linger on the shelf in front of the canned peas, and now you couple that with the idea that has revolutionized the biological sciences. They used to talk about junk DNA, but now they realize there is no such thing. It's all information. I'm going to make a prediction here. If we still need to be at the point where we can no longer regard these instruments as just raw data sources, we will have to bring intelligence to the instrument. We're not far from it.
As a particle physicist, we got a few decades to jump-start you in generating too much data. LHC collision rate is 36 million crossings per second. Each one has multiple interactions. Each event can produce many gigabytes of data. There's no way we let all that data just come out of the machine, right out of the instruments surrounding collisions. We have for decades put many levels of processing that we call the trigger. My favorite definition of the trigger was from a colleague at the University of Wisconsin that said a trigger is designed to throw things away. It couples with the previous definition. You only select out of those billions of bytes of data that can happen every second, billions of gigabytes of data that can happen every second.
We only select a tiny fraction of that to write, store and distribute worldwide computers. The largest single computer infrastructure is the LHC computing grid I helped design. I'm proud of it. But the prediction here is not a competition for data. It's that you're going to have to bring intelligence to the instruments. You'll have to make those decisions, those image analyses, and the design of your experiment before you come to the instrument. When you run a particle physics experiment, you design your trigger before the collisions happen. You decide what you'll keep before you turn the thing on. We're going to get to the point with these instruments that you can't keep every single frame of every single image scan that you do. You can only keep some of the output. You're going to have to design the data reduction to happen there. And still, you'll have a huge amount of data.
HPC Performance and Why Does it Matter in Bioscience? [39:03]
Zane Hamilton:
That's an interesting point, Alan, and it leads into the next topic here. If we talk about HPC and performance and why it matters in bioscience, what you just said, the more performant we can get, does it lead a researcher to want to collect more and analyze more? It's the opposite of what you said, what we need to do, but are we going the other way? Let's talk about HPC performance and why does it matter in bioscience? Jonathon, you haven't talked to me for a while. I'll let anyone who wants to talk.
Jonathon Anderson:
Something Alan said sparked a question in me. I love that quote that you gave, that big data is everything we used to throw away. It's no coincidence that big data has, at least from my perspective, I'm not a bioscientist. My experience has been, and my observation has been, that big data has come up in response to the advent of machine learning. The incentivizing value function of big data has been that when we write learning algorithms, they find things in the data that we didn't understand were there in the first place. I read an article recently, I need to remember where it was, but the idea is that we keep teaching the computers what we already know instead of allowing them to learn things from first principles. By pointing them at a bunch of data every time we do that, they discover things we didn't understand where they were in the first place. We slowed that process down by trying to encode our value functions or our understanding of the area that we're researching ahead of time. Zane, your question was about performance, but the performance here is less of an issue than the storage problem, which is still part of the same thing in my mind, like the capability of the resource. How much can we store? How fast can you get through it?
Alan Sill:
Are we going to have dolly and stable diffusion making up genomes now?
Jonathon Anderson:
I don't know. Is it true that we keep learning things we didn't know before in the data we thought we could throw away before, like how do we make that decision? That's what incentivizes keeping everything because we keep finding stuff that we almost threw away. I don't know what to do about that.
Glen Otero:
There are two lines of discussion I want to bring up. One is fun because it's biologists versus physicists. Alan brings up a really good point. My first thought was, on the instruments, well, at least I'll talk about Illumina sequencers. Hey, Illumina sequences this genome, and they'll send you the file with the mutations. Just the VCF file. To accept that VCF file, you must agree with how they analyzed it. The problem Illumina runs into all the time is like the rest of the research community says, no, your algorithm doesn't find the indels and the deletions properly. Or it doesn't. It needs to do the copy number variation properly.
I have to redo this myself anyway. There is a data reduction process happening. I think with our algorithms and our knowledge of the genome now, to Jonathon's point, we don't have it systematized enough where we can just say, this is not a mutation, or this is the right copy number variation. We'll get there with enough data and enough runs. Biology is a system that we don't understand as well as many of the physics systems out there. The second point was to get to the performance bit again. Illumina has bought this FPGA company, Edico Genome, that I used to work with, and they sped up that analysis several fold.
Depending on the size of your cluster, like on the cluster I used to run on, my previous employer would take 12 hours to run a variant calling analysis just on CPUs. But Edico Genome on their FPG, I could do it in under half an hour. Same 40x coverage, so really, really fast. Illumina's taking these cards and shoving them into their sequencers, just like, Hey, we can get you that result like that. Now the problem is that the FPGA's code is locked. You can't see it. Do you run it against your CPU code to see what's happening? As you said, we're in this battle of trying to time the treatment.
If I run it on an FPGA that is six, or seven times faster, I can get to a drug for a patient in a day. Like any other clinical lab test, which is what we want. What one group will throw away data is somebody else's, someone else's garbage is someone else's, what is it? Dinner? I don't know. They'll say, no, you're throwing any wrong things. We need to look at this. It's a really big issue, and it's being tackled. We're just not, we don't have an understanding, as well as Jonathon, brought up what we can all agree upon to keep or throw away, and maybe we'll never agree upon it. Hopefully, that gap will shrink. It's a big problem as the data gets larger, and we're trying to get to treatments faster.
John Hanks:
From this perspective, the responsibility should be on the person redesigning the experiment to throw away data as part of the experiment. There is some relief coming from that. Somebody was telling me just in the last couple of weeks about one of these single molecule long read sequencing technologies that are up and coming. They'll have the ability when you slice your sequence to put it into the sequencer to tag it so that when that single molecule reader picks up that sequence, it'll look for your tag. Your tag will only be attached to the strands you care about, and it'll immediately throw away the things you don't care about. On the chemistry side of the instrument, it'll be able to filter out and look at strands that you have tagged that you want to sequence.
Alan Sill:
I'll respond quickly to the data volume question because I was trying to make a serious point here. Yes, the design of how you keep data is crucial. And when you design triggers and particle physics, you spend a lot of your time designing cross-checks. But my statement, and I'll stand by it, is inevitable. You're going to have to learn how to do this. Your instruments are getting better and will generate too much data. It's something like three and a half or four terabytes a second that the LHC can process. The LHC IT systems can process as intake. You have to get it down to that level. The statement is that the instruments are improving fast enough, as was pointed out, and this will become inevitable.
John Hanks:
I have an anecdote that will refute that. When I was at the Broad Institute a long time ago, we ran out of storage. We pulled in the sequencing group and said, you need to hire someone to go through your six petabytes of data and figure out what we can throw away because we're running out of storage. Or do we need more money for storage? And without hesitation, they said, here's a million dollars. Buy more storage.
Alan Sill:
Yes, but the instruments are going to grow faster than storage will.
Brock Taylor:
The collider is a really good example of that. The data is coming so fast that you can only capture some or lose valuable stuff. We should have scheduled about four days for this webinar.
Glen Otero:
The part about the LHC I am envious of is this project. Everything trickles down onto that. If we only had one sequencing center like the broad in the US, it might look better like that. The board still has dozens of petabytes of data out in Google's cloud. That's part of the issue. There are these multiple large data centers, and many universities have their sequencing centers. That's good because we're developing better algorithms for many things. You're right. The instruments themselves will, just like John was saying, we are either going to have to go back to targeted regions of genomes that you're interested in or some other kind of triage of data, hopefully. Or be able to process more, which is where the GPUs and the FPGAs have really helped us in the past. We could not rely on more GPcores or new silicon to be able to get us out of this, out of this problem by itself.
John Hanks:
That'll work, except for people who want just to put a sample intake of your dirt. Wash all the DNA out of it, put it in there, sequence every strand, then blast that to see what was in the original sample.
Glen Otero:
Agreed.
John Hanks:
There's always going to be somebody who wants every strand of DNA out of a sample sequence so that they can see what was in the sample.
Glen Otero:
We're doing that with sick people coming, and we don't know why they're sick. Everything gets swabbed and sequenced. If you want to do that for a million people in the next pandemic, you're not throwing anything away.
Zane Hamilton:
Gary, I think it's something you wanted to add something.
Gary Jung:
I was thinking about what Alan was saying about processing the data at the instrument. It's an HPC problem. At the lab, there's a project to do a gamma ray detector that will run in conjunction with a radioactive isotope accelerator. The detector will generate so much data that we use an HPC cluster. This is an example of light processing. We're using an HPC cluster as the first level trigger of the data coming off the detector to calculate the path through the detector and what segments it hits. Sizing that and figuring out how many GPUs you need is an HPC problem. A design decision that they made of using a cluster instead of FPGA is that the thinking is that we could use different containers depending on the experiment or what we're going to be running through the detector. It gives us some flexibility so that you're just not locked into one way of throwing away data.
What is the Biggest Challenge in Genomics Medicine? [51:17]
Zane Hamilton:
We have one question, and I've got another question for Glen and Gary. What do you think is the biggest challenge in genomics medicine? What is the biggest challenge in genomics medicine?
,
Glen Otero:
Outside of the size and the growing size of the data. It's who owns it when it comes to the, when it comes to the, to the gen to genomic data. If you get your genome sequenced in the past, it's undoubtedly been resold or used for other purposes. 23andMe, all these places that will sequence data, typically sell it to pharma companies because they want to find people to do trials on. People have found that they need to be more palatable lately and want to own their data. There's a problem there, though, because you've got hospitals, clinicians, and physicians that have legal liability and things like that.
At my prior employer, there were projects where we were recruiting people like in Alzheimer's studies. Therefore we were going to do their sequencing. They would own that and only be able to opt-in if they wanted their data shared with other companies looking to create cohorts, for example. It's like ownership and privacy issues because how do you protect some people's data and not others? You get to varying levels of what you can share with others or companies. I was also working with a company trying to encrypt this data and send it around and only give people access to certain parts of a genome for these reasons. If we don't want genome data to go the way of social media, we need to get our hands on that. I don't see any leadership, but I'm not really tied into DC about what's happening with these things. But different groups are trying to do that. It seems to be parochial at this point.
John Hanks:
The privacy issue is fascinating and funny. I find the idea that we try to keep sequence data private utterly ridiculous. It blows my mind whenever somebody talks about anonymizing sequence data because there's nothing more uniquely identifying than your sequence. It literally cannot be anonymized because it points to you. We are shedding that unique identifier everywhere we go. We're shedding it as we walk through the environment. It's just a matter of improvements in sequencing technology until I can sequence you by picking up your Starbucks cup that you just threw away and know your sequence and follow you around town. With my phone, probably.
Alan Sill:
Ancient Hawaiians used to have a prohibition against gathering hair clippings or any other material from royalty. Maybe they were onto something.
John Hanks:
This is a problem. It's too late to address it as a privacy problem. It must be addressed as what's legal to do with your data problem, not how to keep it safe. I mean, we are all on the internet. Your data's not safe. There is no such thing as safe data. It does not exist.
Instruments in a Bio Environment [54:57]
Zane Hamilton:
It's very true. Going back, Glen, We've talked about instruments in a bio environment. What are some of those instruments? Where are we going with them? For me, who's not in that field? I can think of a microscope. You mentioned that earlier, but what other things are out there? Gary pointed back. I will not repeat what he said, but it is very complicated to use radioactive isotopes. What are other tools out there that we're using?
Glen Otero:
One that's creating a lot of data in the proteomic space is the mass spectrometer. We haven't seen much data on the HPC side because the programs used to analyze that data are all shut down proprietary, like Thermo Fisher, and you have to run them on a PC. Mass spec is one. Sequencing technologies are moving towards long-read sequences. The thing that impresses me most is what John brought up. I can spend a thousand dollars now and get a DNA sequencing kit sent to my house. It's the same one they put up on the space station and ran genomes up there.
They're going to get smaller and more convenient to use. If I can sequence a genome for a thousand dollars in 10 years, can anyone do it for a hundred dollars? What do we do when everyone's sequencing everything in their backyard? IClouds are going to look a lot different than it is. I have yet to think about other sequencers. I think about data capture. If you're swallowing a pill now, it sends signals to either patch on your arm or your Bluetooth, and people are also collecting that data about your heartbeat, your blood pressure, whatever it's monitoring. That is more invasive, more data, and more time points. It is garbage if anyone looks at the transmitted Fitbit data. Consistency-wise. I mean, it's just so noisy. Those are the problems we're going to see more of.
Brock Taylor:
I joked, asking when I would get to meet my digital twin. A digital twin is a model representing a physical object. It's very big in manufacturing right now, but you can imagine where we'd be headed if everybody had a digital one or more digital twins that exist. You use a digital twin to simulate things happening. Again, manufacturing, like an airplane engine, can do digital twins of an airplane engine, and you start to see when a digital twin fails. Why does it fail? You fixed the engine problem, the engine before it ever failed. Well, this is the problem that Alan's talking about. When you get to digital twins of people, the microelements may be sequencing themselves all the time. That pill you swallow that goes through and reports for five hours is now 24/7. That's flowing data, and you've got to make those decisions. What data do you keep? What just gets thrown away? It's exciting and scary to think about for all of those reasons. It's data about you. Everything identifies who you are, what you are, and then what you do with that data. If we saw everything about ourselves and how we might respond or even die, what does that do to our psyche? It's amazing to think about.
Alan Sill:
I want to challenge the audience to write the words, digital twins of people, down on a sheet of paper and sit down with their favorite screen script writing program and generate our next science fiction content for us before it happens.
Zane Hamilton:
To Brock's point. I've got a digital twin. I want them to have a seat on an airplane that fits me. I want to be comfortable.
Glen Otero:
Remember that's getting transmitted when we talk about pills that get swallowed or your Fitbit or whatever that's going Bluetooth over home networks. Even if it was inside a hospital, those networks and everybody's home networks are not great security wise. That's a concern. You think about it like you're reading off stuff on Alexa into your doctor's office or to iCloud, where your doctor's CRM or Epic has access. That's terrifying from a hacker's perspective.
John Hanks:
There's no such thing as privacy, and it's crazy to see people going on and on about how we need to worry about privacy, given the state of the world. Even if privacy still existed, which it doesn't, people would happily sign it away in the ULA to access the next video in the TikTok stream. It just doesn't exist anymore.
Zane Hamilton:
That's a good point. Well, guys, I appreciate it. We are up on time, and we could keep going for a couple of hours and drill into this. Hopefully, we'll come back and pick another topic, but we'll continue this discussion. I appreciate it. Everyone out there watching, I appreciate your time. Thank you for joining us. Go ahead, like, and subscribe, and we will see you next week. Thanks, guys.
Built for Scale. Chosen by the World’s Best.
1.4M+
Rocky Linux instances
Being used world wide
90%
Of fortune 100 companies
Use CIQ supported technologies
250k
Avg. monthly downloads
Rocky Linux