Webinar
Research Computing Roundtable - Turnkey HPC: What is HPC?
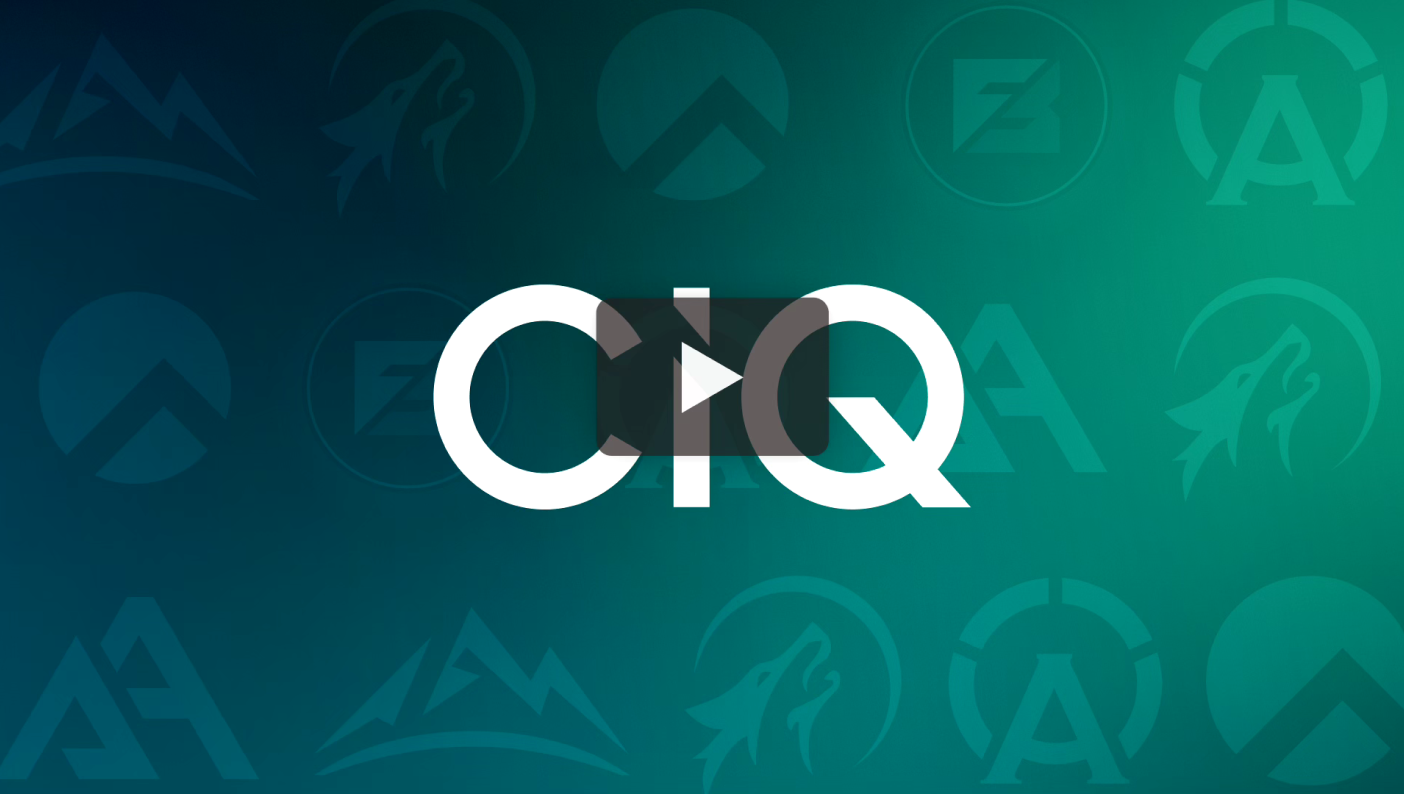
Webinar Synopsis:
Speakers:
-
Zane Hamilton, Director of Sales Engineering at CIQ
-
Krishna Muriki, Senior Software Engineer, KLA
-
Patrick Roberts, Technical Director, Skyworks
-
John Hanks, System Administrator, Chan Zuckerberg Biohub
-
Gary Jung, HPC General Manager, LBNL & UC Berkeley
-
Glen Otero, Director of Scientific Computing, CIQ
-
Forrest Burt, HPC Systems Engineer, CIQ
Note: This transcript was created using speech recognition software. While it has been reviewed by human transcribers, it may contain errors.
Full Webinar Transcript:
Introduction [00:00]
Zane Hamilton:
Good morning, good afternoon, and good evening wherever you are. We welcome you back to another CIQ round table. We had one of these a week before last, and it went over really well so we invited a group back to continue that conversation and see what else we can get from people who are in the industry and actually have real world use cases and talking points. If we could go ahead and bring in the panel.
Welcome back everyone. We have added some new participants this week too. I think last week, everybody got to meet Patrick, John, Gary and Greg as always. So I think we will start with the new guy in the room. If you would go ahead and introduce yourself, Krishna, and let us know who you are and where you are from.
Krishna Muriki:
Hello. I am Krishna Muriki. Right now I am employed with KLA Corporation. But I go a long way with Greg Kurtzer and Gary Jung, both of them here on the panel. Before this, I was employed at Lawrence Berkeley Lab. Greg hired me into that position at Berkeley, and Gary was my math manager and guide. I know the panel very closely. I have worked with Patrick on Slack. I learned a lot from Patrick. Glen, a good friend of mine. I am glad to be here.
I am about 10 months old at KLA Corporation. In this organization, we make wafer inspection devices. In the semiconductor industry, it is very crazy right now with all the chip shortages. The demand for our product is really high. It is a little bit crazy. We are having to stretch ourselves in terms of our HPC designs and HPC technologies. We are adopting to meet these growing crazy demands. This topic is really interesting. I am glad to be here.
Zane:
Very nice and welcome. I am going to go around the screen real quick, just introduce yourselves very quickly again. I think because you introduced yourself last night you do not have to go into a lot of detail, but I will start with you, Patrick, because you are at the top of my screen here.
Patrick:
Howdy all, my name is Patrick Roberts. I currently work with Skyworks. At Skyworks, we design all sorts of different types of chips for all sorts of different things. So sorry, that is rather vague, but we are in all sorts of RF chips, analog chips, digital chips, big and small. I have been around for quite a while. They used to be Rockwell long, long ago, so some of you may be familiar with that. It depends on your age I suppose. But I have been in HPC specifically for a little over 20 years. I have been in the IT industry for 29 or so years. That is about it.
Zane:
Next we have John, aka griznog.
John:
Hi. John Hanks, I have been doing this for a long time. I do not remember what I said last time when I got introduced so I hope I do not contradict myself this time. I was thinking about the topic today and I think maybe I am inherently unqualified to answer this question or even try to answer this question because in pondering it, I think all I do is manage workstations now. They just happen to look a lot like a cluster because of the way I manage them. I do not know that I even do any HPC anymore.
Zane:
No, I think that is great and that is something that is going to be interesting to see. What is HPC to everyone else? I will move next, kind of skip over Krishna because we had you. Greg, you are in the middle.
Greg:
Hi, everybody. I feel like, who is in the Brady Bunch in the middle? Was that Alice?
Zane:
Jan. No, I am kidding.
Greg:
Jan. Thanks. But hi everybody. I have been doing HPC pretty much my whole career. Started with a degree in biochemistry and then failed to continue getting my PhD because I got more interested in computing and Linux and open source than doing science. I have been really focused on HPC and scientific computing ever since. As Krishna mentioned, I have worked with Krishna and Gary for ages at Berkeley Lab. It is great to have this middle line across the board as the Berkeley crew.
Zane:
We will just call it the Berkeley line there. The next person from Berkeley, Gary.
Gary:
Hi. My name is Gary Jung. I manage the institutional HPC computing at Berkeley Lab. Greg and I started the program back in 2003 and it has been going great since. I also have a dual appointment with UC Berkeley. Also, I manage the HPC effort for the institution down at UC Berkeley. Krishna, Greg and I go way back doing this.
Zane:
Glen, you are up next.
Glen:
I have been at CIQ for 16 days. I am the VP of fricking sweet ideas at CIQ. My background was a doctorate in immunology and microbiology. I have a postdoc in HIV research. Then I left academia and fell into HPC with my second postdoc at the San Diego Supercomputer Center. Since about ‘99, bioinformatics and HPC have been my livelihood. I have known Greg since about 2000. So I blame him.
Forrest Burt:
Hey, everyone. My name is Forrest Burt. I am a high performance computing systems engineer at CIQ. I have been with CIQ for about a year. Before that, I was the student HPC system administrator at Boise State University while I was getting my bachelor's in computer science. I got my start in the cloud and in computing and stuff like that in the early 2010s, messing around with the tech that I had available and cloud gaming servers. I am really pleased these days to work with a little bit bigger systems, but I love HPC. I got super interested in it while I was in college. I am really pleased to have been working at CIQ and to be working on everything that we do with Fuzzball and that type of stuff. So great to be here.
Patrick Defines HPC [00:07:56]
Zane:
Thank you, Forrest. Jumping back into the question of what John has started to allude to a little bit, I think that the definition of HPC is going to be a little bit different to everyone here. I would like to go around and ask: what does HPC really mean to each of you, and just tell us what that means. Tell us a little bit about maybe where you got to that point and how you got there. I will start off with Patrick.
Patrick:
I would say, what does HPC mean to me? So HPC is any service which is designed specifically to perform a set of complex tasks, which I know is a very broad definition, but it is very true. Because I have dealt with HPC in all of its various forms from Cray to working on loose grid systems to working on top of very close coupled, very tight systems with InfiniBand and MirrorNet, and just all sorts of different interconnects of all kinds, and it really just depends on what tasks you are trying to accomplish, and what you need in order to meet that task out.
There is a blending of HPC for high performance computing and then there is now high throughput computing, and now there are all these other subvariants, but in my mind, it is all HPC; it’s just with different tasks in mind to accomplish, and different hardware and software solutions to accomplish those tasks. So for me, it is just one really big bucket of designing clusters and computers to meet tasks' needs.
Zane:
No, that is great. Thank you, Patrick. And John, you started down a road of talking about desktops and that is what you deal with today. I know it may have been a little bit tongue-in-cheek, but in reality, that can be something that is really the case. Tell me about your definition of HPC.
John Defines HPC [10:09]
John:
For me, HPC, the primary thing there is high performance. And for it to be HPC, it needs to be high performance, which is why a lot of what I do now is not HPC because I have people getting this massive pile of GPU hardware, memory and cores, then sitting there typing in a Jupyter notebook on that system. It is as far away from high performance as you could ever hope to get, somebody checking out a $7,000 GPU to type and hit enter at a command light prompt.
I think HPC, high throughput computing, those are really the two main designations. And then for me, it is getting even further away from high throughput computing, where we run some embarrassingly parallel workloads, but the most important thing in our environment is on-demand for people interacting. So we are pushing that long tail even further out by pulling in people that do not really need a cluster. They just need a well-managed desktop workstation that has a lot of memory.
Zane:
Very nice. Thank you very much. Krishna, how do you define HPC?
Krishna Defines HPC [11:28]
Krishna:
I think it is changing. I think the definition of HPC is changing. In 2005, when I started my first job in the US, it was at the San Diego Supercomputer Center. The first machine I worked on was from IBM; it is called DataStar. It has a very specialized interconnect, very specialized work servers all put together, but that was not the high-end machine back then. The San Diego Supercomputer Center had a good size machine, DataStar, which is how the HPC machine used to be. The applications that used to run on that application were very optimized, tuned for the IBM architecture, which we used.
These days, I think it is not the case anymore. A lot of technologies have commoditized and interconnect InfiniBand, as it is treated as a commodity. A lot of servers that we use, even the top machines, the top 500 machines are commodity servers from Supermicro and things like that. I think it is morphing. The definition for HPC is becoming a lot more commodities and not specialized machines anymore.
The employer who I work for right now builds stream computers. All that we have is a lot of data streaming at us. We have really high-definition cameras looking at this nanoscale layer width, transistor width, and things like that. It is really high volume data, just a fire hose of data being shot at us.
The biggest machine that my employer built is just three racks, not hundreds of racks or even tens of racks. It is just three racks, which is the biggest that we have. It is all commodity hardware. We do not have a specialized server design team at KLA. We do not have a specialized architecture team at KLA. We all take white boxes, Supermicro, InfiniBand, Commodity, and just try to build. I would consider what we are doing at KLA is HPC because the data volumes that we are dealing with, if that is the definition, does come under HPC. But hardware wise, it is all off the shelf. It is changing. HPC definition is surely changing, is what I would say.
Zane:
That is very interesting. Talking about commodity hardware versus very specialized hardware that it used to be. I think, like you said, things have changed.
Patrick:
I do not think that is inherently a bad thing, though. I really think that commoditization of the hardware has brought HPC into the hands of so many more people. It is actually a very good thing that you can build a super equivalent with off-the-shelf parts. I did it for a very, very small but, one could argue, quite important X86 design company, where they did not have the money to go out and really invest in a massive super to solve their complex problems. But instead, I was able to put together desktop machines, literally on a folded piece of metal.
And I would go to Fry's, rest in peace, and purchase off-the-shelf hardware and literally take it back and have one of my guys put it on a folded piece of aluminum and bolt the bits to the folded piece of aluminum. I would have bread racks from Sam's Club with 44 machines per rack in an open air data center, because it was designed originally for mainframes. It was designed to have an open air concept. And roll in the bread racks of 44 machines. We could do it and add thousands of cores at a fraction of the price that it would take for us to go to IBM, Supermicro, Dell or anybody to have it done.
So just the ability to do that, it allowed that small, little 100-person company to compete with the likes of AMD and Intel. Not necessarily effectively compete, as they got bought by Intel last year, but they were able to compete. Just that alone, that commoditization of hardware, is not a bad thing in my opinion. Yeah, it is really cool to work on those really highly specialized systems, but it also is amazing what bringing that capacity for computing to the general populace can really do.
Zane:
Before I go to Greg for his definition of it, coming from an enterprise background, I have watched people build commodity infrastructure to solve enterprise problems that probably should have been HPC in the past. It is interesting to see the merging of the two worlds where you see HPC becoming more commodity hardware. Maybe those workloads are moving from just general enterprise into an HPC environment where they actually get handled in a much more efficient fashion. I have been excited to see that merging. Now I go to you, Greg. What is your definition of HPC?
Greg Defines HPC [17:08]
Greg:
Before I even mention that, I just want to respond to Patrick's point, I completely agree. We have seen this happen so many times in research organizations, especially governments and academia, where in many cases, people that have never done system administration before are even buying clusters. They are not managing them very effectively, but they are getting research done. Because of small budgets, they are actually able to do very good research on it.
At some point, typically they will graduate and be able to have proper support for those systems. It is not a grad student or even an undergrad or postdoc who lost a bet and now has to go maintain that system in many cases. They can actually reach out to somebody like Gary's group at Berkeley and LBL, to actually solve that problem and manage it appropriately. But hitting commodity has lowered that barrier of entry so high that it’s been fantastic.
Now, to get to the question you asked me, Zane, I have been doing this for long enough to have a very strong opinion of what high performance computing is, or rather was. It was tightly coupled parallel processing at massive scale. I remember times where unless you can prove that your application could run effectively at massive scale, you did not get time. You were actually down revved in terms of how much time you can get and what priority you get to run in, unless you can demonstrate that level of efficiency.
Now, we called it the long tail for a long time, which was all of the scientific applications and workloads that are running on these systems, which are not that massively traditional high performance computing profile. What we have seen over time, at least what I have seen over time, is that long tail has just continued to grow. Griznog, you kind of alluded to that as well, that the long tail has now grown so far out that the long tail is now becoming the dominant form of workload on many of these systems.
I have had many, many arguments over drinks at supercomputing on what is high performance computing, and are these sorts of applications actually high performance computing. It took me a long time to actually get to this point in my maturity as a person to say that high performance computing is now more than just those massive tightly coupled applications. It is anything that is going to spin or peg some aspect of that hardware at 100% and then literally bottleneck based on that subsystem of your hardware.
Whether that is CPU, whether that is memory IO, whether that is bandwidth, you are going to peg something on your system. That is where I am in terms of the definition of high performance computing. It could be a single thread hitting one core, or even a hyper thread on your CPU, but it is pegging something, dang it, somewhere, which is where I draw the line.
Zane:
I like it. Efficient utilization of resources, which is all I am hearing.
Greg:
I am not sure if it is efficient in many cases, but it is definitely useful.
Zane:
Pegging it, resource, whatever. Gary, your definition. You have a fairly large environment to deal with.
Gary Defines HPC [20:53]
Gary:
It is interesting when Greg talks about that, it brings back a lot of memories. It was exactly the way he said, where we are looking at really big usage of the system. We do take a simplistic view of what HPC is. We work with a lot of researchers, large team science, and then also individual researchers. A lot of individual researchers may have a very powerful workstation, they may have a laptop. Then at some point, whether they are doing data acquisition or simulation, they are going to outgrow it. They have to move to something.
What is that something? And then for us that is where we define the HPC. Instead of coming up with one offer for all these people, then that is our opportunity to move them into an HPC infrastructure. And then I think everybody's kind of covered that, but there's enough convergence in the field so that now when you describe an HPC infrastructure, everybody knows exactly what you are talking about.
Greg:
One thing I just want to follow on what Gary said, speaking of memories, we did see a lot of people that started off on a laptop then went to a powerful workstation. Then we initially, when we first started the HPC project at Berkeley, it was around this concept of something called mid-range computing. Mid-range computing we defined it as everything between a workstation and a giant facility like NERSC, and everything in between.
NERSC at that point was limiting people unless they can prove a reasonable amount of performance and efficiency in terms of running on their system. They did not want applications running on that system unless they were efficient on that system, because it was such a big, expensive resource.
One of the big motivations we had for this group was this idea of mid-range, and how do we solve these mid-range problems? To me, it has now turned into what I call sweet spot computing. This is where most people are focused on. For example, in high performance computing, this is the vendor's bread and butter. This is where they are selling the most systems. It is why we are talking about turnkey HPC, honestly, because it comes right back to that. It is the problem set we actually really need a solution for.
Gary:
Yeah, that was a huge gap. I think essentially when we did that early, we helped to define what is known as the mid-range computing segment within the DOE.
Greg:
I think that actually even articulates how HPC was changing even then, Gary, without us even really coining it or thinking about the nomenclature. We were changing what HPC was even then. It started off being those giant systems and then it was moving towards mid-range, and it has been still following in that trend and in that direction ever since.
Zane:
That is very interesting. Thank you very much, Gary. Next we will move to Glen. You have an interesting perspective on this. What is your definition of HPC?
Glen Defines HPC [24:20]
Glen:
When I started in this field, HPC was tightly coupled, pretty large scale machines, Right? You are in that elite interconnects. But when the life sciences started adopting the Beowulf architecture, it rarely had those little latency interconnects because it did not really need it. They were coining at high throughput computing on ethernet.
It is not really HPC. It is just high throughput. But in my head, I was like, same architecture, it does not have your low latency interconnect. It is a different type of parallelism. I still thought that it had to be something like HPC.
Then in my head it evolved to, so, how do I lump those two together? Then that became research computing. Research computing was all these folks, whether it was molecular dynamics or just DNA alignment, and embarrassingly parallel or tightly coupled, it was all research computing. Then the enterprise was doing it. Now what do I call it?
Then to John's point, I have worked on machines with all these GPUs that are running a Jupyter notebook, but you better have that RAPIDS library and that Python tuned, optimized really well so they get the most out of it. At my last employer when we were working on my title, which was not VP of fricking sweet ideas, unfortunately, we talked about, well, why not scientific computing? You are trying to get science done, really, whether it is Johnson & Johnson trying to figure out how to design their next shampoo bottle or if it is COVID genome analysis I have come to call everything that we have just talked about scientific computing. If you had to nail me down to HPC, I would be thinking of systems that try to look like the top 500, tightly coupled, the scale of the top 500. Anything smaller than that is still HPC. I am not sure what my mid-range HPC red line is, but that is how I view the world these days.
Zane:
Thank you, Glen. So, between what Greg had talked about and what you talked about, I used to be on a mid-range team. And mid-range to us just meant the Solaris team and one of our guys called himself the VP of Blinky Lights because we had the data centers.
Glen:
Wow, that is a good one.
Zane:
Not quite as cool as sweet ideas, but pretty cool. Forrest, what is your definition of HPC?
Forrest Defines HPC [27:16]
Forrest Burt:
I would define HPC surrounding the efficiency, which we are trying to achieve with HPC. We have hypothetically a single commodity server that we are trying to do some traditional HPC workload on, whether that be simulation or just to roll them both into one thing, some type of embarrassingly parallel high throughput type of job. If you only have one server, you are not going to be able to get the performance you would need out of it in order to actually efficiently iterate on the work you are trying to do.
To me, HPC comes down to being able to do these really, really complex things in an efficient way, regardless of what the system underlying it ends up being, whether that is a bunch of EC2 instances, which is a traditional tightly coupled cluster, whether that is a cluster that is more meant to provide as many GPUs as possible, just to do mass model training, that type of thing. I think ultimately it comes down less to the comprising of the system itself. Are you building the system to gain performance beyond what you would get with the base component of this system? In that case, I would say you are doing HPC.
I would also touch on the long tail concept because where I very, very first entered this was more so in those tightly coupled jobs. Especially at my last institution, I started to see a lot of researchers coming out of what I felt were non-traditional places for HPC. We were not just having genomics people or simulations people, that type of stuff. We also had education or people from the education department that were trying to do mass data science crunching for their research.
We had people on the high throughput side. We had people that were taking LiDAR data and doing huge amounts of analysis in a high throughput way. I definitely think that in addition to being about efficiency, we are definitely seeing this kind of enterprise. Some of these other use cases realize that they are trying to do HPC without realizing that they are doing it. We see maybe some of the muddying of the waters here as some of these nontraditional entities enter the HPC sphere.
Have HPC workloads changed over the years? [29:35]
Zane:
Absolutely. That leads into one of my next questions, Forrest. And thank you for that. I think we all alluded to it at some point, but have the HPC workloads changed over the years? We talk about the infrastructure getting better, and the machines getting better. Obviously, they are getting more and more dense and being able to be more capable. Have the workloads changed over the years? And I will throw that out to anybody who wants to take it.
Patrick:
100%. Absolutely the workloads have changed. You have some of the traditional workloads, which are still around and NPI-based ones. We touched on this. I can not remember if it was in one of our dry runs or in the actual meeting last week, but we definitely touched base on who is still using MPI. What are your percentages of your workloads of different types and that sort of thing. It was definitely eye opening.
I expected it in some ways, but it still was very informative that the mixed workload is really here to stay. Even in very specific industries, the amount of workloads your singular cluster or clusters are supposed to be able to support is massive. You are supposed to be able to support GPU-based AI training or ML training versus being able to run ultra-fast single-threaded performance or highly parallelized jobs. You are supposed to be able to run it all in one.
All of those require different pain points to overcome in building the HPC clusters. I think we are expected to, as designers and administrators of those environments, we are supposed to be able to provide for all of those in a one-size-fits-all. It is difficult to accomplish.
John:
I think to some extent, the commoditization of the compute part of this has made HPC simple to do on the compute side. Where the actual interesting stuff being done now, and probably more so in the future, is on the storage side. Storage is actually becoming the critical thing about everything we do, regardless if it is HPC high throughput. At least for life sciences, a lot of on-prem clusters and on-prem infrastructure exist today because you just simply cannot move the data sets around. The compute has to be brought to the storage. The compute is more or less secondary to just getting all your data in one place where you can work on it.
Krishna:
Not only that, but the way the compute is being orchestrated or designed is quite different in the enterprise. Enterprise is dealing. I know of use cases where they are dealing with data pipelines at the rate of multiple tens of gigabytes per second constantly coming. That is the data volume they are dealing with. The workflows and applications they are running, they do not have any NPI. No NPI at all. Nobody in that organization knows anything about NPI. So yeah, NPI is not the only HPC. The data volume'-s are a lot higher than many organizations deal with, but these organizations do not have anything NPI.
John:
Lack of NPI in the enterprise pushes me into an old man rant moment about a negative connotation of HPC. In the early days of HPC, I remember about 20 or 30 years ago when I was getting started in this, there was an elitism aspect to HPC. You had to be able to afford to do it. It was a very elite club and not everybody got to join that club.
That attitude carrying forward over the following 20 years from when supercomputing got started, led to us having to deal with things like Hadoop, Spark and Kubernetes and all this just nonsense, complicated, extra complexity, poor performance because that is the stuff that came out of the enterprise. At any point during that, we could have just said, “Hey, enterprise people, how about you help us write a better NPI and let's do this right, efficiently and simply, with a simple scheduler.
Patrick:
I do not think it was that it was not offered. I think that it was not necessarily accepted amongst industry, because so many different corporations were trying to capitalize on that market. Or, well here, let me enter my proprietary standard into this ring. Let's go ahead. And they did not want to do an open source either, generally. They wanted to really make it very tight and hey, well, now that you have entered into our ecosystem, you are stuck.
That is what came about, I think. Then of course you had the competition, as you said, where now we have got Kube and now we have got all of these other competing, not exactly optimal frameworks to put it lightly, to do a lot of the same workload that could have been much better implemented in HPC.
Zane:
Let me ask this, Patrick and John, from an enterprise perspective, if I am looking at this, the way most of those other languages and other tools came out was to be simple and fast. If I look at NPI, it is probably a little more complicated to program correctly and to get it as efficient as it should be. Was it something that from an enterprise perspective, people did not want to take the time to learn how to code correctly for NPI? It was just easier to use some other framework?
Patrick:
It was a major barrier to entry. Because of that barrier to entry, whether it be I did not have the hardware to have that high speed memory interconnect between the nodes, or I am an undergrad, I cannot afford to get time on a super at the lab, or whatever it was. There is always a barrier to entry. Or if it is Intel NPI, do I have to buy into the entire Intel ecosystem? Do I have to go buy an Intel compiler back in the day? Does that mean that now that I am running on my AMD chips, is it going to be slower because Intel spiked the compiler? Whatever it is, it depends upon the environment, but there was always a barrier to entry. Then of course, you had open NPIs; now we have competing standards.
Greg:
I am going to push back a little bit because I think It is very use case specific. The use cases and the applications we were solving in high performance computing were very different from what enterprise was really even looking at solving. Now, the MapReduce kind of focus there, I do agree. We could have done better in terms of cross-pollinating between Hadoop and then Spark and then HPC NPI, NPIO, and other things.
We are starting to see that also happening in AI, starting to. This is a few years old now at this point. But things like, how do we do training? How do we optimize training? How do we start parallelizing training? If anybody remembers some of the early training frameworks, it was not running NPI. They created something from scratch, almost like NPI did not exist. It was a duplication of effort.
To the point, Patrick, I think you made this, it may have been Griznog, but if we would have come together right at the beginning, in a better way, more open way, I think showed more interest in collaboration, I think we would have seen very different outcomes. This goes back to, I joke about this, in all of the organizations I have seen and worked with that have HPC-focused people, traditional HPC-focused people, there is IT and then there's HPC.
Even if HPC is under IT, they do not talk; they do not work together. They do not leverage each other because the technology is both so different and they are solving such different problems. There is also just this legacy mindset on both sides. It is not until fairly recently, in my mind. Containers were the Pandora's box that really started blurring this. It was not really until containers did anyone in HPC even care what the heck was happening in enterprise?
We took off our gloves at that point. On the enterprise side, it was not until just recently did they start looking at HPC saying, how do we do training faster? How do we do better analytics? How do we get more insights from our data? That to me was the crossover point. Now we need to actually all come together and realize we are solving very similar problems. We need to stop innovating on our own and start actually working together and solving problems together. We need to be a big, happy family again.
Zane:
Need to be, yes. Gary, I think you were going to add something to that.
John:
What do you mean “again”?
Forrest Burt:
I just wanted to riff off that, a little bit of a side jump, but back to the topic of how HPC is changing. Something that I really see is the hardware side of HPC changing. It seems for a long time, we had InfiniBand, CPUs, GPUs, and that was kind of the basic components of a cluster as far as the compute part. We start to see now that there are a lot of companies that are starting to invest in creating new solutions around some of these very specific use cases.
Now we see for AI, we are only 10 years off of Alex Krizhevsky, I think I pronounce his name right there, training AlexNet on two GTX 580s. And now, 10 years later, we already have companies that are trying to replace the GPU for AI training because there are better ways that can be done than just on GPU. Some way that I really see it changing is on the hardware level.
We have got new ways of training AI, new much faster interconnects than we have ever had available before, new storage technologies. It seems to me like we are really in a renaissance of HPC hardware, the use of PCIe as a broader interconnect than just to put accelerators into a cluster and stuff like that. It seems like a Renaissance as far as HPC hardware goes. Our clusters are really starting to change from just the basic CPU interconnect GPU type of thing with FPGAs specialized chips.
Zane:
Forrest, I am glad you brought that up because that reminded me of a question I had for Gary last time, when he made the statement that his GPU usage has gone up by 3X over the last few years. What is driving it? Is it something that is more hardware specific since it is difficult to just go purchase whatever you need because it changes all the time? Is that what you are seeing? Is that what is driving it?
Gary:
For a lot of people, they are working with workflows. Somebody wants to put together or string together an infrastructure with a web server and a database and do some computing and move some data, and it is pretty easy. The term I like to use is frictionless computing, because they are able to do it themselves without having to, say, wait for a network drop in a data center and then for somebody to set up the machine and all that. It is a lot faster.
Then probably the other thing is it’s just really easy to prototype things. New tools like AutoML and SageMaker make it really easy for people to get started using machine learning. It is interesting. We will onboard people onto the cloud. We will kind of prototype it using AutoML or something like that and they will get going. Then sometimes what happens is they will find out it costs a lot of money and then we will go back. Now they see how efficient it is and how this helps their science, we will go back and transition onto our on-prem HPC using maybe slightly different tools.
Vector Processing [43:16]
Zane:
Interesting. I was just curious because it is interesting to see how it is taking place. I talk to a lot of different customers who are HPC, who are trying to, like you said, try things out on a cloud before they actually make the investment to see if it’s going to work for their needs or do what they expect it to do. Thank you.
We have a question from Mr. Ignite, so before we pop it up, I can go ahead and start reading it. Nobody has really talked about the vector processing as a part of HPC buildup. The example that is given is a medium scale IOT collection and analysis of billions of devices generating tens of bytes per second, driving anomaly detection, pattern recognition, and other applications. It is an interesting topic. I do not know if you guys have any thoughts on that.
Real-Time Stream Processing As Part of Cluster Work [44:01]
John:
I would be curious to hear if anybody has ever done anything on a cluster that was real time. The closest I have ever got was a group that wanted to produce a weather forecast every day. They had data arrive and then they had a deadline to get stuff out, but I do not recall ever having anybody who wanted to do real-time stream processing as part of a cluster workload.
Gary:
Actually, Krishna, Greg, and I could probably have an example of where we are currently building a system for a gamma ray detector and the system is going to be the first level trigger of the data coming off the detector. It is going to be a Kubernetes cluster. We are going to run different containers depending on what is going to be analyzed. We are using, instead of FPGA hardware, they figured It is going to be a lot more flexible to do with a cluster. It is currently being put together, engineered and put together right now.
Detector Research [45:12]
Greg:
I figured Gary and Krishna would have some examples here. There were multiple different examples of people doing detector research. Basically, you can think of a detector as a very simple idea of one. You have some sort of reaction happening within a spherical enclosure. Then you have a whole bunch of detectors all the way around this spherical enclosure. It is monitoring all of the different types of rays and what is happening at the center of this sphere.
All of these different detectors are creating streams of data. Then these streams of data have to be computed in parallel in real time, so you can see and visualize what is happening within that experiment. Sometimes these are actually in real time, but in many cases it is near real time. Which basically means now you actually have to look at it and then decide, do you want to rerun the experiment? Do you have good data? Then feed that back into your process flow. It needs to be real time or near real time.
As Gary just mentioned, if you are running it through Kubernetes, it is an extraordinarily different architecture than what we have been doing in high performance computing. Without even knowing the specifics on it, I would say probably it is a bunch of services, which are running and doing the equivalent of an inference on each one of those data streams coming in, and then computing on those via GPU. This is like a performance critical service that is running.
Kubernetes is a good architecture for managing services. I am curious about the efficiency that you are getting, because I have actually had really bad results with regards to Kubernetes. Just to go on a quick tangent real quick, we actually were talking to some people that were doing something along these lines with Kubernetes. Because Kubernetes is so big and bloated, it was utilizing about 15 to 30% of the underlying hardware resources just to run Kubernetes.
Now let's scale this up. Imagine buying a 5,000 node cluster or even a 500 node cluster and 100 nodes out of your 500 nodes is just running your scheduling system, your orchestration system. You are basically losing 20% of your system to run just for Kubernetes. At a small scale, I think Kubernetes would do it. As soon as we start scaling bigger, we have got to come up with better ways of doing that.
DDN Appliance For Real-Time Stream Computers [47:51]
Krishna:
Another approach I have seen in the industry for these real-time stream computers- is a traditional way If you look at a DDN appliance, a storage appliance, they usually come in pairs. They come with some fault-tolerant resilient designs using basic technologies like Corosync, such that services can flip from one controller to the other controller automatically if there is a failure in top controller. Luster services can move on to the bottom controller.
That is the basic components that anyone would use for fault tolerance. I am sure that even Kubernetes is built on some base components like that. In the industry, I have noticed, if you build a real-time streaming computer, where your machine goes into your production pipeline and they need real-time feedback of the quality of the product being manufactured, and if this computer is down and that quality is not being generated depending upon the value of the product you are making, it can cost multiple hundred thousands of dollars per day of production loss. That is very costly.
This real-time computer giving the feedback on the quality of the product is a very critical component. When you are designing such a computer, fault tolerant designs, having backup components is so critical and things falling back automatically from one server to other server is such a critical thing in your design. If you do not have it, you are costing hundreds of losses to your company, to your product. That is something that I am noticing heavily.
Not everybody in the enterprises is adopting Kubernetes. They are just trying to accomplish that using the proxy Corosync services, which have existed for a long time and trying to put together everything, like having redundant switches, redundant network cables, run from servers to switches and have services for failover from one switch to the other switch. If there is a switch failure or a network cable failure, those are another tangent and extension to HPC design that I am seeing in the enterprise, which is very critical and needed when you are building a real-time computer for these use cases.
Zane:
From my past experience, most of what we were trying to do was as much analyzing of data at the edge as we could so that we were not sending massive amounts of data back to be processed or analyzed. We were looking for that anomaly and then whatever data was driving that is what we were sending back. From a real-time usage, I feel like just because we were trying not to send a lot of data that may have been irrelevant, we were doing a lot of pre-processing at the edge. Then only moving back what was necessary for the next type of action or the next time event that needed to be taking place.
But that is an interesting question when you are talking about massive data. Like in Greg's example, it is not really an edge use case, because everything is right there together. You are trying to do it in the place that it is taking place. From an IT perspective, when you have billions of sensors out, that amount of data streaming back could be something that would be overwhelming. A lot of that at the end of the day just gets dropped on the floor and you never even look at it. There are a lot of different ways to approach it when you look at IT. It kind of depends on what you are trying to accomplish, in my opinion.
Customized Hardware For Real Time Computing [51:49]
Patrick:
My history with this was back at Bell Labs. We were monitoring numbers of phone switches in real time. And in order to do that, it was required, this was quite a long time ago. This was the era of the 5E, that should explain how old this was. They were quite new. It was one of the first digital phone switches. But it required massive amounts of customized hardware to monitor these switches.
Today, I do not see the investment in that type of customized hardware for real-time computing as much. It is kind of uncommon to see really, really specifically focused hardware for real time computing like we used to see quite a lot. You would see real-time Linuxes. You would see real time that were made for all sorts of different RTC clocks and RTC controller cards and things like that, to ensure absolute peak timing and synchronization.
We see some of that today, but not nearly what we did maybe 20 years ago. I think that is either because the performance of machines has gotten so much better and we are close to an okay level, or our standards have slipped a little bit. Or maybe a merger of both, I am not sure.
Zane:
That is great. Thanks, Patrick. We do have a question from Sylvia. Have the programming languages and interfaces changed over time?
Changes Over Time of Programming Languages and Interfaces [53:39]
Greg:
I think It is a great question. Yes. When I was first getting into this, Gary, you may attest to this, we actually had to help a lot of scientists and researchers upgrade to Fortran 77, because they were running predecessors. I forget what Fortran it was before 77, 60-something. That was the year by the way that the spec was created.
Yes, it has definitely changed. We have seen a lot of that. Now, on parallelization, we have seen NPI really taken off. We have talked a little bit about the different forms of NPI. The specification has definitely evolved over time. That specification has created a lot of compatibility. So even though we have many different NPIs, coding for any of those NPIs should be the same no matter what, if you are adhering to the strict specification. There are some alternative or optional pieces of the specification, but as long as you adhere to the strict specification, it should be NPI compatible no matter what.
Now, every programming language is going to have its own aspect or interface to NPI. You could have, for example, C bindings, Fortran bindings, Fortran 90 bindings. More recently, we have seen a lot of interest in higher level programming languages like Python. Python has absolutely taken off in scientific computing, to the point where I actually think it is probably one of the most dominant programming languages nowadays. I am curious from others: are you seeing the same thing? And what is that like in industry-specific areas like life sciences? Is it similar there too?
Python and Scientific Computing [55:35]
John:
Our software stack is almost entirely Conda at this point. There are a few applications in there, Bowtie, same tools, that kind of stuff that is not in Python. But for the most part, at least 70 or 80% of everything is done in Python. Maybe with a mix of R in that too.
Greg:
Glen, are you seeing the same thing in your area of life sciences?
Glen:
Yes. Things have evolved from Perl to Python. Python has really done a fantastic job in adding libraries. Then of course, Conda to support the researchers. It is, I think, the most popular language. There is a lot of R in there. From folks who come from a statistical side, they seem to like R a lot. But Python is, this is just my opinion, but if you are trying to do a lot of other things than just statistics, Python is much better. You are not bringing up a web server in R. We see Python doing a lot in the life sciences.
The folks that are using C are the folks writing the algorithms, whether it’s for DNA alignment or drug docking or on the GPUs, trying to get their stuff onto the GPUs with CUDA. Those are the folks using the low level languages. Everybody else is a little bit higher up at the Python level. There seems to be a little bit of a push to, I can't remember the name of the project, but actually getting some actual typing, static typing as opposed to just dynamic typing with Python for some things in research. I am actually interested to follow that. Yeah, that is what the trend has been.
Greg:
It is funny for me to even consider and think that HPC has changed so much over the years. We are not even focused solely on compiled and purely efficient languages that we are running across a whole bunch of different languages. I think there's even some Rust that I have heard of, Patrick, I think you've mentioned Rust as well, coming into HPC.
Rust And HPC [58:07]
Patrick:
There is Rust. Also there's still some Ruby creeping around in the EDA world and moving in there. But primarily these days it is Python and Rust and again, some Ruby and of course, sadly Perl still exists. The Swiss army knife.
Zane:
You are making Greg cry.
Greg:
Okay. Are we done with the webinar yet? Come on.
Patrick:
No, I love the Swiss army chainsaw, but I also hate it. If you have ever walked into a project where you have had somebody whose been in their own Perl world for years and years and years just designing it and designing it and designing it, and just adding on. Then you try to take over that project and figure out what was actually done with this stack of Perl scripts, it can be fun.
Zane:
Then you rewrite in Bash in like 10 lines.
Patrick:
And make you hate Perl.
Zane:
Yes. We have a new question from Dave. I have seen so much SciPi and NumPy usage at scale these days. There are a lot of compiled time optimizations to be considered for those libraries alone, Intel compiler and KL.
Scientific Libraries For Higher Level Programming Languages [1:00:20]
Greg:
Yes, there are a lot of scientific libraries that are also becoming more and more available for Python and other higher level programming languages like SciPi, NumPy and others. I have not seen, and I am curious if others have seen, architecture optimizing math libraries like MKL, Intel MKL. Are there interfaces for Python for these? Or are we moving away for the specific need of high performance, absolute tuning, profiling and building the fastest programs ever in exchange for more general purpose HPC applications? I am kind of curious. I know we are at the top and I am really curious about people's take on that. Maybe that is where we leave off for our next round table.
John:
It has been at least seven or eight years since I have built a Blaze library to do specific optimizations. I think at some point the hardware got fast enough. People, certainly at the level I have to deal with, do not care about getting the last five or 10% out of anything. The next generation of processors will solve that problem for us. They focus more on writing a better algorithm in general, rather than trying to get a little bit more compiler optimization out of it.
Glen:
When Intel came out with their Intel Python and then MKL and Blaze and Boost and things like that optimized underneath, we were really excited to use it, but it just did not seem to play well with the typical Conda, PIP tools. When we tried to install it, it just kept blowing up on us. We gave up on it because we thought we could get a lot of good performance gains from it because everyone was using Python, but it came down to just usability.
Or just trying to introduce it into the already mature Python environment everyone was familiar with. We did just try to use these libraries and things like that; it just did not work. We eventually dropped it. Other than that, we have not built or compiled anything specific for tuning or a library in it in a really long time.
John:
My most frequently used C flag is M2 and Generic, just because I want it to run everywhere. I do not care if it’s fast, I just want it to work everywhere I am going to run it.
Patrick:
See, that is what it really comes down to, is that you can make snowflakes that work really, really, really well one time. Making something resilient that can run everywhere all the time and reduce your issues is so important to HPC in my opinion. If you are constantly retuning, retuning, retuning for different architectures and different last 2% of optimization, then how much time are you losing to get that 2% when you could just throw more hardware at it, throw more cores, throw more memory or throw a cloud at it? That is the other aspect, is now that you can fairly easily scale up. It is easier to do that than it is to optimize just down to that little tiny little bit at the end.
Zane:
Thank you for that, Patrick. We have one more question before we wrap up. Are there analogs to MKL for things like the ARM64 architecture?
ARM Clusters [1:04:24]
Greg:
I do not have an answer specifically for the question. I would assume if there is not already, that they are coming soon. I just wanted to say hey to Dave, It has been a long time since we chatted. It is great to see you here.
Zane:
And thanks for all the questions, Dave.
Greg:
Does anybody else know if there are optimized versions of math libraries for ARM? Dave, we are going to probably have to reach out to some people that are running some ARM clusters. I know Sandia has some pretty good size ARM clusters as well as maybe some ARM people. We will hopefully have an answer for that either in the chat or maybe for our next webinar.
Zane:
I am taking notes. Well, guys, we are a little bit over and we do appreciate your time. We know you guys are busy and thank you for joining us again. Krishna, it was nice to meet you. Gary, John, Patrick Glen, Forrest as always. Greg, thank you very much. Guys, go out, like, subscribe and we will see you next week. Thank you very much.
Built for Scale. Chosen by the World’s Best.
1.4M+
Rocky Linux instances
Being used world wide
90%
Of fortune 100 companies
Use CIQ supported technologies
250k
Avg. monthly downloads
Rocky Linux