Webinar
Research Computing Roundtable - Turnkey HPC: Hardware Infrastructure
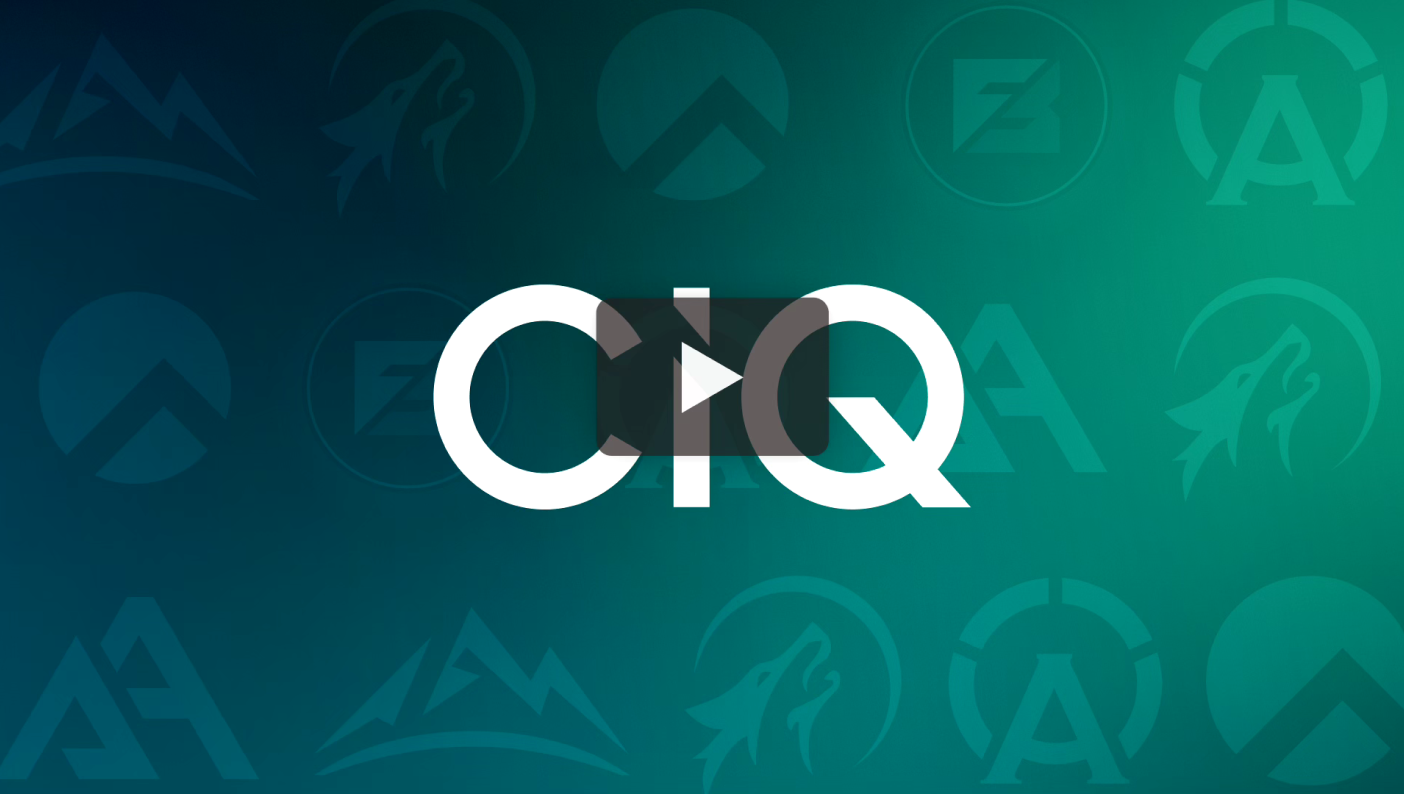
Up next in our Research Computing Roundtable series, our HPC experts will be discussing Turnkey HPC and will be focusing on hardware infrastructure. What are the most important and critical decisions one should be making about purchasing their HPC resource.
Webinar Synopsis:
Speakers:
-
Zane Hamilton, Director of Sales Engineering, CIQ
-
Gary Jung, HPC General Manager, LBNL and UC Berkeley
-
Jonathan Anderson, Solutions Architect, CIQ
-
Forrest Burt, High Performance Computing Systems Engineer, CIQ
-
Glen Otero, Director of Scientific Computing and Genomics, CIQ
Note: This transcript was created using speech recognition software. While it has been reviewed by human transcribers, it may contain errors.
Full Webinar Transcript:
Zane Hamilton:
Hello everyone. Welcome back to our third in the series of HPC Turnkey Solutions. This week we want to talk about hardware. We know that purchasing an HPC system can be a really big investment, especially for small research companies. We've been getting a lot of questions about HPC procurement and what people should be thinking about when they go through to purchase a Turnkey Solution. We have a panel here today, let's just dive right into it and bring them in. Welcome. We have Gary, Jonathon, and Forrest. We may have someone else join here in a little bit, but I will let you guys introduce yourself. Gary, your first on the list. If you want to give a quick intro.
Gary Jung:
My name is Gary Jung, I run the scientific computing group at Lawrence Berkeley laboratory. We run the institutional high-performance computing for Berkeley lab. I also run the HPC for UC Berkeley, they have an institutional program there. I manage that also.
Zane Hamilton:
Very nice. Jonathon.
Jonathon Anderson:
My name's Jonathon Anderson. I am a solutions architect with CIQ, but in past lives, I've done a number of HPC procurements at a bunch of different levels and am happy to talk about it.
Zane Hamilton:
Thank you very much. Forrest, welcome back as always.
Forrest Burt:
Thank you Zane. Great to be on the webinar. I'm Forrest Burt, I'm a high-performance computing systems engineer here at CIQ. I do a lot of work with our fuzzball product working on different high-performance computing workloads and that type of thing. I got my start in HPC working in the academic national lab space and got to see a major system get put together over the course of a couple of years while I was there. I've definitely seen a little bit of the procurement side of things as well. Once again, thanks for having me on.
Zane Hamilton:
Absolutely. Thank you. I think the first question I want to ask is, is there really a one size fits all when it comes to purchasing a HPC-and we talk about turnkey for what this is-is there really such a thing? Is it one size fits all? And I'll start off with Gary's top list again.
Purchasing a TurnKey HPC Solution: Is there a one size fits all?
Gary Jung:
If you have a lot of money you could build a system where it is one size fits all, because everybody has different things that they want to optimize for. In real life, a lot of people don't have that luxury of being able to buy a system that can fit all. They have to go for what fits most of what you want to do. When you build a system, you do have to take into account the requirements of what you're going to be doing with the system. There is a requirements phase that's going to be translated into an architecture, and that's a critical part of doing a system.
Zane Hamilton:
Absolutely. Jonathon, what are your thoughts on that?
Jonathon Anderson:
I think the closest thing we have to a one size fits all solution–that we didn't have when I first got started–is the public cloud and that you can get started with a really low investment, trying things out, seeing how your application runs across different architectures, different accelerators, different environments, and different processors; but it's not going to be optimized for you. That'll give you some experience to then determine how you might want to deploy something on-prem that would be optimized for your solution. That's the name of the game for a HPC environment is tuning it and optimizing it for the kinds of workloads that you're wanting to run.
Zane Hamilton:
Thank you, Jonathon. Glen, you popped in an odd angle here. I'll let you introduce yourself and then I'll throw the question to you. Then we'll finish up with Forrest. Do you believe there is a one size fits all HPC?
Glen Otero:
I'm glad I came in late. I don't want to answer that question. Glen Oterro, I've been at CIQ a couple weeks, and I'm the director of scientific computing and genomics AI and ML, until we can bring on other experts in that area. My passion has been HPC bioinformatics, before there was short read sequencing. Happy to join and talk to other folks battling with these issues from a research perspective. That's what I've been doing for a long time now.
Zane Hamilton:
Now you've got Jeremy. Glen, you don't want to answer that question?
Glen Otero:
Do I believe there is a turnkey?
Zane Hamilton:
One size fits all turnkey HPC solution, do you think that exists? Or is it even something we should think about?
Glen Otero:
No. Having tried to be in several different situations and organizations where we've tried to do this with small, medium, large. At Dell we created essentially a turnkey HPC system for genomics; everybody liked it but they said, "Just change this little bit to it" or "Could you swap out the hard drives for these hard drives?" I think you can get really close. We used to talk about it like getting people started on third base instead of starting from home plate running to first. I think you could get people, like everybody 80% of the way there, then the last 20%-I think I heard Jonathon talking about it at the end-is site specific or application specific tweaks from the hardware and software side.
Zane Hamilton:
Excellent. I think it's a great point. Forrest, I know that you've been through building out a large system and going through procurement. As a part of that, would you say there's one size fits all?
Forrest Burt:
In general, at the moment I'm not sure if there's really a well-defined concept of that. I think that at a certain point, within the last 15 years, we switched over from more of a state where there could be an easier concept of a turnkey system. In general, as HPC is complexified–especially with AI and new accelerators over the past 10 years–the needs for a cluster have gone beyond a high speed network and some CPUs that we can run stuff on. As that's complexified and then whole other fields of science have propped up around that, like AI, it's become necessary to complexify what we would call the "Beo" Wolfe model in order to handle these new use cases. At one point there may have been an easier concept of turnkey HPC, but at the moment there's so much complexity and HPC has developed so much that it's a little bit of a difficult concept to put together.
Zane Hamilton:
Keeping on the same topic, what would it take; what would answer that question? What would make an HPC capable of being one size fits all? If you could fit everything into a box that would work for everyone, what would that look like, Gary?
Making an HPC capable of being one size fits all
Gary Jung:
I had started off saying, if you had a lot of money then you would essentially buy something with a lot of memory, a lot of CPU, good IO and good interconnect. It just becomes really expensive when you do that. Some places they can do that; they can buy something, but essentially you may be spending money on something. It really depends what you want to do with the system, if you're doing something that's going to be very general purpose for like whole institution–and a lot of us are doing that for academic institutions or research institutions–where instead of building a whole bunch of little systems, which could be harder to manage in one large, you are trying to build something that fits the 80%. You just have to be really careful about requirements gathering. You could figure out where that 80% is going to be and try to aim for that. Then there may be some compromises for the rest. How many compromises you make is where the budget comes into play.
Zane Hamilton:
Glen, do you think that we should be thinking about this more vertically inclined–for a specific vertical in life science, or in some sort of mechanical engineering, those types of verticals–is that more of a fit of where you could actually probably get closer to having that beyond 80 or 90%?
Glen Otero:
I was going to say yes, until I just remember what Forrest said about artificial intelligence and machine learning. What made the life sciences a little bit simpler was you didn't really need the low latency network. You could just have a bunch of boxes in ethernet and you could do just fine, right? Then your bottleneck was still IO, but you didn't need the communication between nodes. It simplified it. Now that data is massive. The ability to wrestle with that-whether it's Hadoop and spark and in the cloud data bricks-now it's completely different. It's not just HPC bare metal, on-prem, and your provisioning tool, whatever it is, right?
You've got to now figure out Pig, Hadoop, Spark; when you tried to do cloud on-prem, It used to be-what's the one I'm trying to think about-the on cloud open source tool that was hot for a while, OpenStack and Ceph-you were trying to address those things in a different way. Those were all problems that you had, but you didn't have the low latency problem, but you had to get your data in somehow. You hit a point where it's really now a problem of the data size–if you're under a petabyte I think you can manage it in a typical HPC centric way, or legacy way, "Beo" Wolfe way with NFS or cluster feeding your nodes–once you go beyond that, or you want to start aggregating different data sets, then you're in the new world now where we live. Which is why I think the cloud was really perfect for genomics, because people are just putting these massive data sets out there that have all this gravity and you've got to go compute there and try to combine results. It used to be simpler, but now the data sprawl has made it not so simple.
Zane Hamilton:
Thank you. Before I ask another question, does anybody else have anything they want to add to that? Jonathon? Jonathon's thinking.
Jonathon Anderson:
I don't think so right now. We'll see.
Zane Hamilton:
We talk about low latency between nodes and the network stack. I know everything seems to be getting faster and better; have we seen a lot of increased performance in that layer of everything? Or is that quickly becoming the bottleneck of everything–CPUs are getting faster, memory's getting more dense–is that interconnect communication layer, that network layer, is that something that's holding us back or is it keeping up?
Performance of Latency Layer
Jonathon Anderson:
In my experience, the interconnects have outstripped most applications, the ability to take advantage of it. That was the case even in the HDR 200 era and even more with 400, at least that's what I've seen. There are certainly applications that need all of that bandwidth, but if we're talking about a one size fits all base level, it's to the point where you basically don't even need current gen interconnects for your entry level cluster, because even low latency ethernet is good enough for most people.
Zane Hamilton:
Forrest, did you have something you wanted to add?
Forrest Burt:
I basically agree with Jonathon there. What I've seen is that it seems like if anything CPUs–with Moore's law and that type of thing–is where we're starting to see the leveling off of performance, because GPUs are getting faster and faster. We're seeing novel accelerators coming out that are going to replace the GPU in some places. Just like Jonathon says, these higher performance networks, like the 400 type networks, in a lot of cases you can't even keep that data pipeline moving. I would definitely say that networking is not a place that we're seeing bottlenecks up.
Zane Hamilton:
Gary, whenever we start looking at a turnkey HPC solution–whenever I start thinking about HPC–I think about needing that high throughput, maybe that's something that you don't necessarily need in a way to optimize and save cost. Would you agree?
Gary Jung:
Restate that.
Zane Hamilton:
I mean, if you look at the cost of some of the high speed interconnects, it obviously drives the price up. If we can–like Jonathon said–ethernet's probably capable of a lot of use cases. Is that something that maybe we should stop looking at, everything has to be super high throughput all the time? Is that a place where we could optimize that?
High-speed Interconnects: Optimizing Ethernet
Gary Jung:
I wouldn't go as far as to say it is not completely needed. You may not need to put as much into the interconnect. When we started doing clusters a long time ago, everybody was worried about having a non-blocking fabric. I think you can get a lot of mileage out of an infiniband blocking fabric and you can still get the low latency. And to Glen's point about the data, we use the infiniband for moving the heavy lifting of moving of data. For us–a lot of the applications now–a lot of people are running Python jobs and that hits the file system really hard. I'm concerned about making sure that you have a good enough pipe for the IO to handle all the IO that is going on.
Since we're talking a little bit about bottlenecks and optimizing–things that are important when I think about building a system–the thing that hasn't grown really fast relative to everything else is the memory bandwidth speed, if you look at the mid speed of the processor to the memory subsystem, that really hasn't increased a whole lot to keep up with the amount of cores that are now available and the speed of the memory. When people are picking out or building a system, that's something they should look at carefully is the processor choice for the memory bandwidth, not so much some of the other factors.
Zane Hamilton:
That's a great point, Gary. Thank you very much.
Jonathon Anderson:
We saw that in very real world terms too, where we had what seemed like very similar CPUs between two different clusters at a past site. We couldn't figure out whether they weren't the same exact CPU, but it seemed like they were closely clocked and all of that. The same application was running at very different speeds between them, it ended up being the amount of Ondi cash and per core cash there that made the memory performance that much better on one of those clusters than the other. That directed our CPU choice for a future cluster.
Zane Hamilton:
Very interesting. Forrest, did you see anything like that when you were building out?
Forrest Burt:
Nothing specifically related to that that I can think of right off the top of my head.
Zane Hamilton:
We talk about optimization for making sure we consider things during these hardware purchases. What else do we think we could optimize, Jonathon?
Optimizing other Hardware
Jonathon Anderson:
From a hardware perspective, a big question is CPU versus GPU. I think the public cloud is a really big win here because you can test your application on a variety of different GPUs before you make a purchasing decision and then decide; we need single precision performance, or we need double precision performance, or we're doing inference we don't need any of that, and select the right GPU for your workload. Then allocate the budget that way.
Forrest Burt:
Maybe to tie that into what we were just talking about, that's one of the big bottlenecks in GPUs. I'm not sure if it's much with some of the latest stuff that's coming out. One of the big basic bottlenecks there is the data movement from the host of the GPU that can really slow down simulations. Something else to look at is: what are you actually using to interconnect these GPUs and stuff with the plane that they're going into? Is that something that has the memory bandwidth speeds that are going to be necessary to efficiently move even just data between the host and the GPU? And a similar thing to the processor, that's also something to consider with GPUs; what's their memory bandwidth? What can they actually transfer into time to make sure that, especially when doing AI, that doesn't become a bottleneck.
Zane Hamilton:
I think we had a question about GPS actually. How do we decide how much of our budget should we put in GPUs? That's a good question. Because it seems to be all over the place in terms of price. Gary.
Budget: How much of it should go towards GPUs?
Gary Jung:
I was just thinking about–as we're talking about GPUs–you could almost ask the same question: is there a one size fits all GPU? Because there's quite a big selection of GPUs, whether you go with Nvidia or AMD. One of the critical things when we choose a GPU is the amount of onboard memory going to be needed with the GPU. That seems to be a critical thing that is growing. What you may choose for–what might be suitable for your GPU workloads today–may not be what they would be tomorrow because the size of the jobs that are going to be running on it may need something bigger. At some point we're going to run out, they're not going to be able to make a GPU big enough. The critical things that people are looking at are: nickel, and things that will allow you to run across multiple GPUs. It's an interesting problem. But I would tend to try to buy the GPU with the biggest memory that you can afford right now.
Jonathon Anderson:
I have a question for Gary and his experience there–so just what I've imagined anyway–you were talking about memory performance and memory bandwidth at the CPU level; where I see people actually appearing to need high-performance and low latency interconnects is in multi GPU workloads, where they really do have a workload that's bigger than any of the GPUs memory that you can buy. Is that what you experienced, that the GPUs differently than CPUs have enough memory bandwidth internally to be able to benefit from that when you're doing multi GPU workloads? Today, that's what you really want to be looking at; these non-blocking low latency 200 gig, 400 gig fabrics.
Gary Jung:
I think we're going to be there. It's something that we're all thinking about right now is how we're going to get there; because at some point, you're not going to be able to connect up enough GPS or maybe you have some existing system where you bought the GPU last year and the memory size is a little bit under, or your job size is bigger and you want to keep your investment viable. Working on distributed GPU solutions is a big thing that has to be done.
Glen Otero:
What I would add to that–with regard to budget–you'll have companies that will definitely say, "You've got these high end GPUs" and they won't necessarily mention their low end ones, right? So we did some testing; at our high end GPU would finish our job in an hour and a half, but I would go with the lower power GPU–maybe use one or two more–and I would get like 80%-85% of the performance and it would finish in two to two and a half hours. Well, if that's sufficient for my purpose–if that fits in my window of what I need to get done in a certain amount of time–then I can save a ton of money by buying just a few more of the low power GPUs to get that job done.
I say that because if you can save money that way and buy the model of GPU, it can have a big impact on how much you spend versus just having to get the latest greatest all the time and figuring out how you're going to power them and feed them. If it's really not going to benefit you from how much work you get done. I understand from a pure performance standpoint, but you can actually save a lot of money by testing on other platforms that are less powered; they suck up less power and cost less money. That's something that a lot of people don't think about. From the genomics world, it's not a lot about this interconnected communication. It's a different space.
Zane Hamilton:
That's a great point, Glen. One of the things I wanted to ask you–since you're focusing on AI and ML–are there things outside of GPUs that are coming now that people should be taking into consideration or planning for that next gen, what's coming next?
Next Gen: Beyond GPUs
Glen Otero:
There are dozens of AI specific chip bakers coming out specifically to attack those problems. I can't think of the one off the top of my head, It's like cerebricks or cera...?
Forrest Burt:
Cerebras, the Wafer-Scale Engine.
Glen Otero:
There's probably another three or four that start with C E R E. I've seen them have success at some centers that work on genomics and have a lot of genomics researchers. The heterogeneity of HPC clusters in that space is just going to continue. We're going to fill in the long tail of what it'll be for tweaking that last bit of performance. It's like, "Oh, my GPU gets me 80% of the way there" to "But if I get this specific chip, it'll go much faster." It's like, "Okay, well cost benefit analysis." I think we'll see that in the next three years, the use of those in the space is going to really explode; as long as they're in the ballpark with the GPUs, which is hard not to be, they're not cheap.
Zane Hamilton:
As far as I know, you and I have talked about this quite a bit; are you seeing customers that are going that route and starting to implement those types of things for AI and all specific workloads?
Customers: Implementing AI-specific Workloads
Forrest Burt:
I haven't seen any customers here specifically looking into that type of thing. I think a lot of that stuff is still in its infancy. It's really just been–as far as I can tell in the past couple of years or so–people have realized that we can do better than the GPUs, specifically for AI. I know that there's cloud offerings that are out there at the moment to get people access to some of that stuff. I know for example: AWS, at the moment, you can spin up some of Havana labs, Gaudi accelerators, and stuff like that. There must–in some places–be a want to mess around with that technology, because we're seeing some of these major cloud providers making an effort to give a low cost and easily accessible option to people for those.
I think at the moment it's still in its infancy, especially the AI stuff. Something that we have seen is FPGAs. Those are starting to get deployed at different sites around, because people are starting to realize that signal processing. Another example: video and coding. People are starting to find that those can be effectively incorporated into HPC and can provide good results there. So we're seeing some stuff like that. I think specifically on the AI side, it's going to need to go a little farther for some of those chip makers to really start to proliferate out into the market.
Zane Hamilton:
Thank you. Glen, are you seeing any of this in the environment that you have? I mean, it seems like it's continually growing and evolving. Are you seeing any of the newer technologies come into that?
Growing and Evolving: Newer Technologies
Glen Otero:
The FBGAs have been around for a while, actually for more than a while. Specific ASICs to do appliances just for blast alignment–I'm talking like 20 years ago. When during the human genome project we just had to get all this alignment done, places like insight pharmaceuticals, they just had these ASICs computers that were just doing blast and that's all they did. And that benefited them. But what happened for everybody else, is that they were this unicorn in one trick pony that was like, "Oh, I can't make it do anything else so it doesn't really help my HPC cluster." You see this cycle of like, "Oh, they're really good at... I really need this to do this blast alignment.
Well, it's come back around again to like, "Oh, it really helps me with short read alignment." Again, the cost versus what you get out of it is difficult to justify. In my experience, the GPU has been better for that type of workload. Mostly because the GPU can do a lot of other things; the GPU will allow me to do AI and ML, this FPGA won't, because all the FPGA solutions are all closed. You can't get in there and mess with anything and reprogram. If you do, you're hiring really expensive programmers. I think just the Swiss army knife has always been winning out in the genomic space, unless you are sequencing a million genomes, or the UK genome project where you can afford getting a few machines that are going to be 24/7 doing one thing, and you've got another HPC cluster doing everything else.
Zane Hamilton:
Gary, are you seeing this as well?
Gary Jung:
I'll just have to agree with the last two and I don't have any direct experience, but I was just chatting with somebody and it is making me think of the analogy of people using specialized hardware for crypto mining where they had done that. I've done some consulting for people where they didn't want to do that. They wanted to stick with something that was more flexible as Glen was saying, because of things like hard forks which would cause you to have to redo everything. You get more life out of something that's more flexible, like a standard GPU offering. I just clarify one too, about Glen saying not having to purchase the most expensive GPU. I agree with that. When I was talking earlier about buying something with the most memory you can get, that doesn't necessarily mean the most expensive GPU. Look for something maybe where it's a little bit down on a skews, but still has a lot of memory and you might be able to save some dollars.
Glen Otero:
No, I was going to try to sum that up; clock speed isn't everything. But that's probably not the greatest summary. There are a lot more trade offs than people realize when it comes to trying to budget and size their cluster, which again makes this whole turnkey thing difficult.
Zane Hamilton:
Forrest, you and I have talked about this before is people who have an existing system. They're talking about adding something to it, or if they're going to buy something new, will a piece of hardware fit their needs? Is that something where the cloud can come in so you can go try those things out, see if it actually fits your need before you spend the money, test that, and make sure you're going to get that return that you want. Is that something that people should be thinking of doing?
Adding Hardware to Existing Systems: Using the Cloud
Forrest Burt:
I would say absolutely. Like we've touched on here, there's a lot of different models, different GPUs, all types of different hardware out there that's available. Instead of having to actually reach out to a company, establish a business relationship there, get a hold of that technology and set it up at your site. That's a lot of logistics that the cloud immediately abstracts from you. Absolutely, if you have the ability to hop onto the cloud. In some cloud providers, things that make it easy to get up and running with–I'll continue to use the AI example–but with deep learning tools you don't have to spend as much time tinkering around with the configuration of the actual test environment. Of course you may want to for some considerations, but for quick testing–there's even stuff like that available–the cloud is a great way to get access to a wide variety of hardware at once. Without having to invest everything that it takes to actually bring that infrastructure to you otherwise.
Glen Otero:
There are bare metal clouds out there as well. I specifically tested the latest and greatest from one GPU company bare metal in a cloud provider. That's definitely doable as well. You don't have to make adjustments for performance degradation; you can get right on the bare metal in many cases.
Zane Hamilton:
If you have a workload that you're not going to run as often, or if it's something that just comes up, is that a good alternative to fix that other 20%? If a turn HPC gets you 80% of the way; is that a good alternative for maybe "I don't need all of that. Maybe I can just utilize the cloud when I have those apps that will run."
Glen Otero:
I think. The difficulty has been figuring out what the 20% is. Like the old adage of 50% of my marketing budget is wasted, I just don't know which 50% of it is. We find that customers are just struggling with finding out which 20%–let's say–is causing the most problems or can be offloaded and fit in that model. I definitely agree that that's a viable solution.
Jonathon Anderson:
From a compute perspective, that can absolutely be the case, but people shouldn't forget about their data load also. If they have a little bit of data, that's easy to move up to something to run, or if the cost of storing that data in the cloud over time doesn't override the savings of running your instances only when you need them in the cloud. Then that can be great, but it's very easy for the cost of your storage to incrementally just go up and to the right forever. At a certain point, it certainly becomes cost effective to bring that in house, but determining that transition point can be difficult.
Zane Hamilton:
Excellent. Gary, I know you were nodding earlier, does the 80% and using the cloud, is that something that you see as possible or a good idea?
Gary Jung:
We at Berkeley lab–I had mentioned one of our earlier sessions about our cloud usage–we have a large on-prem footprint but our cloud usage has gone up too. I would just say, generally it has tripled in the last two years–our cloud usage for scientific computing. The way we've identified people is we have a large institutional system and people run on it and then they're having problems with it, or they need a specialized software stack. Sometimes it's just easier to do it on a cloud. We had somebody who wanted to run galaxy–which is a BioStack–if you wanted to build by a galaxy on and put it on your system, you're talking about maybe a couple of weeks investment in building up all the software to get that all up to date running on your system. Then you have to keep it up to date. Whereas, there are companies that sell all pre-built and turnkey on a cloud. We don't have a huge amount of usage of it. It makes sense for us to peel that off, put that onto the cloud. It actually works out, even though it may be expensive to run, it's a lot less than maintaining it for us for the amount of usage we use.
Zane Hamilton:
That's a great example. Thank you very much.
Glen Otero:
There's one thing I'll add to that too; there's organizations out there that also want to flip that model, they would really like to be able to automate 80% of their workload and make it efficient enough that running it in the cloud is cost effective. Then spend the 20% bringing all the exotic, new, latest, greatest cutting edge stuff on-prem that would be too costly to host in the cloud or isn't even available in the cloud. They would like to be able to do it that way as well. It's just figuring out how to make it, what fits where? On that sliding scale of effort versus cost.
Zane Hamilton:
I think one of the last things I want to ask you guys, I'm going to throw this up for each of you to answer. Obviously, you guys have been through the procurement process–you bought HPCs, you built HPCs–what are some of the lessons learned that maybe you could share that would help someone who's going through this right now to make this decision? I'll start off with you, Gary.
Procurement Process: Lessons Learned
Gary Jung:
I'm thinking about people who are doing it for the first time; I think it's really important to try to analyze the requirements and stick to that, and know ahead of time what you're going to go in for. One thing we didn't talk about– and the reason why I think that's important–if you don't deal with vendors all the time where you don't look at technology a lot then it can be easy to get swayed by a vendor who comes along and offers you a great deal on something. Unless you really know your requirements and can stick to them, you may end up getting a solution that might not work out well for you in the long run.
Somebody could say, "Oh, there's a great power nine solution." If everybody's developing on Intel, it doesn't really matter how good of a deal it is. That's an extreme example, but that does happen with people–especially at institutions where the choice and the approval may not rest strictly with the technical people who can advise that. Then the other thing I'm going to say–that we really haven't talked about–I'm a little less concerned about picking compute hardware and vendors these days, because a lot of the market has converged. But the storage: picking the storage, storage solution, and the storage vendor are critical, because without that the whole cluster's dead in the water. I'd say that's a really critical thing, more so than maybe the compute.
Zane Hamilton:
That's a great point, thank you. Mystic Knight–welcome back as always–makes a comment, the biggest issue they're having is the GPU bandwidth between the motherboard and the GPU card. How are folks dealing with that bottleneck and planning their workloads?
Forrest Burt:
From a programmatic level? I can't really speak too much because I haven't done a ton of GPU programming for a little while. On a programmatic level, just making sure that your code is running as optimally as possible. If you're not moving data back and forth unnecessarily, things are not going where they need to go. Like I said, I haven't done a lot of GPU programming in a little while so if anyone has more specific tips.
Jonathon Anderson:
Beyond the trying to save money part of the conversation; there are solutions in this field, both of the major GPU vendors have mechanisms for linking multiple GPUs beyond the bandwidth limitations of the motherboard and the CPU. You've got an NV link for Nvidia, and I forget what AMD calls their solution. Its infinity fabric is what they call it. At least Nvidia though–I'm sure that AMD has something like this too–there's mechanisms for getting direct memory access between the network and that fabric and GPS on it. There are solutions, but it is the tip of the spear of HPC development in reducing that very bottleneck right now. That's where the research is happening.
Forrest Burt:
Is a GPU direct RDMA in the end video side they were talking about? That comes up with the memory problem on GPUs as well. In order to do some of these really common operations–like what we do on a host like RDMA–it does on some level require specialized stacks and hardware like NV links. That is another layer of complexity within the GPU thing, you can't just connect those together node to node. Sometimes those require specialized interconnects dedicated to themselves, like the NV link type thing.
Zane Hamilton:
Thank you guys. Forrest, you're next on the screen, I'll let you answer that lessons learned question.
Forrest Burt:
One of the biggest lessons I got out of it was–from the human side–to make sure that the technical people still have the final say that they need to have, versus administrative higher levels. A lot of the times–even from not just the technical standpoint–the people that are putting these clusters together know about what's going to be most effective to even get the hardware and we even had issues at times with, "Oh, there's three dozen boxes that just got delivered at a site 250 miles from us. They're calling us saying, 'what are we supposed to do with all this hardware that's arriving here?'" Making sure that there's considerations for things like getting all of the hardware from one place, instead of trying to piecemeal it out from half a dozen to a dozen different organizations–like some institutions will try to do because they're required to do it that way. Essentially I would just make sure to consider–even an administrative standpoint–the opinions of the people that are going to be building and managing this cluster, because a lot of the time the logistics themselves of even just getting the cluster there can become a pain. Oftentimes there are better options than what might be coming from a higher level.
Zane Hamilton:
Good point, Forrest. Thank you very much. Glen, you're next on my screen.
Glen Otero:
I'll add a little bit onto what Forrest was saying; if you're getting a cluster for the first time, it's likely not to be the last time, so I would really plan for power cooling in space for your next cluster–which will be like in three to five years. Particularly, if you're going to be using GPUs, liquid cooling is probably going to be in your future, right? Depending on how much of it you're using. I'd be concentrating really hard on how I'm going to deliver the power cooling as this beast gets bigger and runs hotter for the next couple years. Because the last thing you want to do is run into.But that's one of the reasons we found people were jumping to the cloud many years ago is they just ran out of space for keeping years of clusters around and other things like that. But more importantly, if you can't power it and cool it–especially at the rate we're seeing all these different processing chips come out–you're going to shoot yourself in the foot without finding it a proper home.
Zane Hamilton:
That's a great point. We didn't even talk about liquid cooling yet or some sort of liquid immersion. I will let Jonathon answer this one and maybe if we have a minute, we can go back and talk about that for a second.
Jonathon Anderson:
My big philosophical lessons learned for procurement is to embrace incremental growth and heterogeneity from the beginning. This is something I think I went too far on in past lives of what even is a cluster, and it's really just a bunch of compute, and you can tie it together in any way you want. There is value in discrete concepts of this is a cluster and this is its head node and that thing. Some of the most successful deployment experience I've had is where we had a nominal cluster that we just added nodes to all the time, whenever researchers had the budget. That cluster had like a 10 year lifespan by the time I left it and it had nodes in it from all the way from the first year still.
It had all kinds of GPUs and all kinds of fabrics, just bolted on and grown. We got really good at managing the scheduler around, we had ways for everyone to use all of the resources, but then have dedicated access to their own. It was a really good situation for everyone that put money into the pot, because they all benefited from what everyone else had put in. That was true as a total some of its parts sense, but also with the access that they had to all of these different technologies and from doing the incremental growth, you can deploy not what you think you might need or what someone's trying to sell you, but what you've demonstrated you need because you ran a smaller thing on a little part of it and then you thought, "Wouldn't it be better if we had more of this?" or "Wouldn't it be better if we had the next generation one?" and when all you do or those big multi rack cluster deployments, it can be easy to get into the mindset of thinking that you have to make all your decisions up front and put all of your money into that one basket with one vendor or one technology. There are very good ways to be successful buying a few nodes at a time and running. Then from that experience buying some more.
Zane Hamilton:
That's a good point. Thank you very much. We have a couple of minutes. If we want to dive into liquid cooling and where that is going and what we're seeing. I see a lot of different vendors out there doing it. I saw TAC actually had one where it was full liquid immersion. It makes me a little nervous when somebody talks about putting liquid in a data center–let’s just call it an old habit–but it seems a little bit odd. Gary, have you seen that taking place?
Liquid Cooling and Immersion
Gary Jung:
Most of our purchases now use direct liquid cooling. We've used liquid cooling in rear door heat exchangers–we've been doing that for over 10 years now. We first started off using passive liquid cool doors. That really helped. We're maxing out our data center–some of the things that Glen mentioned about running out of power and cooling–we're doing it, we're there. Now the issues are: you're using up your water loop, what are the options? Do you add another cooling tower to the roof of the building? Do you add more pipes? Do you put in bigger pipes? Our strategy right now for cooling is to get more heat into the same water without having to make big infrastructure changes.
The way that we've done that is by going with instead of passive cool doors, you go with active cool rear doors. That meets multi passers through the door and you can set the speed. Then we take the water from that and then we do direct processor cooling from the water with that. This way we're increasing the Delta T of the water without having to change our infrastructure. That really buys us a lot more for cooling without having to make huge cost prohibitive changes to our building infrastructure. That is our strategy right now.
Zane Hamilton:
Do you think if someone is new coming into this building–their first cluster–do you think that's something they should plan on or plan for is some sort of alternative cooling method beyond just air cooling? Should they be thinking–with liquid immersion–should they be thinking about those types of tools?
Gary Jung:
It may depend on the building. A lot of it just depends on what building you choose. If you have access to water, then that's going to be important. There's a lot of important things about selecting a site for a cluster, other than power and cooling. For example, a lot of new buildings are green construction used lightweight aggregate, and they can't take heavy racks. People may go along thinking that they're going to do this, and then ask our facilities people to put all the stuff in and then come to find out like, "Oh no, you can't actually put that into the room, the floor is not going to support it." There could be like a whole discussion on site choice for data centers.
Zane Hamilton:
No, that's great. Thank you. Forrest, I know you and I talked about this whole thing before too. It's cool. Your thoughts on it?
Forrest Burt:
I haven't seen–for example, the cluster we put together that I've discussed procuring–we were not doing liquid immersion or anything more advanced like that. It seems like liquid immersion itself while, people have been doing that to their gaming PCs for years–correct me if I'm wrong–but I'm not sure if that's an incredibly old thing in HPC. I think liquid immersion is just starting to make its way to those GPUs. Much like Gary, I saw us end up going with the indoor cooling system. The rear door of the cluster basically has water circulating through it. I don't have as much to say about data center design but I've definitely seen, even on clusters that are not doing more advanced liquid cooling. On a cluster that was not at a major major institution, like one of the much larger, very big national labs. What might be considered a smaller institutional cluster, people are starting to do that type of thing. I think that type of indoor rack cooling system type stuff is very common out there at this point.
Zane Hamilton:
Thank you. Glen, how much of this have you run across?
Glen Otero:
Not much actually. The systems I've tended to work on haven't required it, but the reason is they've also designed their racks so they leave open slots. They don't fill a rack with GPUs, because they'll plan for enough space where they don't have to make that density in a single rack and then have to liquid cool that. They tend to leave enough space they can still passively cool their GPUs. Again, I haven't dealt with any crypto mining clusters where that's the thing to do. I think with more efficient AI type chips coming along that could offload some of that stuff from their really hot running GPUs that we might still be able to get away with that in the genomics AI space.
Zane Hamilton:
Very cool. Jonathon, I know that you have some thoughts on this one and just power consumption in general.
Jonathon Anderson:
I think liquid immersion is mostly a science experiment. I've seen sites where it exists, but I've never seen anyone doing it in production in a way that wasn't about immersion. I direct to chip stuff. There's a couple of good products on the market. That thing still scares me when you're bringing the water into the node. We're seeing more OEM integrations–I think that's viable, but I think anyone doing any data center site readiness analysis right now should be planning on rear door heat exchangers.
The last site that I was at our data center was built out with an expectation of what had–in that time been the classic best practice of an enclosed hot aisle–we had a lot of air movement, and it was about as good as you could possibly do with a standard enclosed hot aisle row. It started capping out at how much we could get the heat out of the air with that much air volume. I think anyone looking at building out a data center like that today–even if they don't need a rear door heat exchanger today–the issue with our deployment there was that the aisle was too narrow to retrofit rear doors onto it without redoing the entire layout. You should at least plan out how much space you would need to add that in for the future, I'd advise anyone who's even looking at hot aisle and closing a hot aisle that they would go to rear doors instead, because it's basically the same thing, only more efficient, less air volume, and you can do it per rack rather than for the whole room.
Forrest Burt:
I just want to say this should go without saying, we always joke about backups. Obviously, if you're going to have a data center and have your cluster and all that sitting inside of it, there needs to be extremely good monitoring and reporting about the status of that center in general. The last thing you would ever want to have happen is, come into work one morning and the cluster is destroyed itself because the cooling system went off in the middle of the night or the whole building is burned down or something like that as a result. While it should go without saying, I do just want to make sure to point out, if you are investing all this money in a data center and all that, you need people, you need engineers, you need systems, all that stuff to make sure that when warning lights go off–at least at some point–someone gets woken up somewhere.
Zane Hamilton:
Absolutely. It is interesting. We have run across a couple of instances where that's not necessarily taking place. That is very important. Thank you, Forrest. Before I read Mark's question, Mystic Knight actually said we have come full circle; in the 1980s, super computers were almost all entirely liquid immersed. I guess we're finally coming back to that now. Thank you for that. Then Mark's question is, "What about smaller clusters? When does it become necessary to go from a closet cluster to requiring a professionally built and maintain data center?" I guess it was scale because we do run across a lot of customers, especially smaller startups, like customers who are doing this in a closet, just in the building. Jonathon, you want to say something?
Small Clusters: Closest Cluster to Professional Data Center
Jonathon Anderson:
I guess it depends on your institution. My background is in university space where there's a lot of people who want to run a closet cluster and there's autonomy in that, there's benefit and there's immediacy in prototyping, and it's your equipment and you can mess around with it right now. In my experience you're much better off pooling your resources with like-minded people at your institution than doing something in a closet. The benefits that you lose out on in doing that–the benefits you would've had from the closet–you're better off getting in the public cloud to do that immediate prototyping. Then you don't have to even worry about your closet burning down, or filling up with water because your water leaked. I honestly think that by the time you're talking about a cluster, you're really better off not building a closet cluster. You should either centralize that and get benefits of scale or outsource it and get the benefits of scale in a different way.
Zane Hamilton:
Gary, this might be something that you're dealing with. Your thoughts on that?
Gary Jung:
If it's going to be more than a half a rack, then you're probably going to run out of power cooling in your office space. You may get a little further in the lab space because they may have heavy equipment in there and they may have more cooling and power, but it's still going to be ultimately limited. Maybe another consideration to think about would be, if you are moving data from other institutions or collaborating with other people, then for your site or your institution, where is your network egress and where is that? If you have the big high speed pipes coming into the data center, but then you happen to be in a different building from the data center, then the next question may be, "How do I get the network connected up from my office?" There's other considerations other than just the straight housing of it.
Zane Hamilton:
No, that's a great point, thank you. Glen.
Glen Otero:
I'm in violent agreement with Jonathon. Keep that short and sweet.
Zane Hamilton:
Perfect. Forrest, do you have anything to add to that one? If not, I think we're out of time.
Forrest Burt:
I would agree that collaborating with the other people that are doing the same type of work that you're going to be doing and that have a similar interest in getting a cluster together is probably going to be one of the most effective ways to determine exactly what the scale of the operation you need is because at that point, you're taking it from your individual person or your individual lab and hypothetically creating a resource that other people are going to find out about and one on. At that point it's good to just get the stake culture together. I completely agree.
Jonathon Anderson:
One of my favorite little pithy sayings is that nothing is more permanent than an interim solution that works. I think closet clusters have a strong tendency to become that where you start prototyping something and it works really well. As a result, you start depending on it and it wasn't built to be depended on. It was a thing that you built in a closet and it behooves people to plan for that dependency. That cluster is going to be important and you should treat it like it is.
Zane Hamilton:
I think that's a really good point, Jonathon thank you for pointing that out. I really bet everybody's got a story around that. With that, I will wrap it up. I appreciate you guys joining us today. Thank you very much. Thanks for the questions and we look forward to seeing you next time. Thank you.
Jonathon Anderson:
Thanks Zane.
Built for Scale. Chosen by the World’s Best.
1.4M+
Rocky Linux instances
Being used world wide
90%
Of fortune 100 companies
Use CIQ supported technologies
250k
Avg. monthly downloads
Rocky Linux