HPC in the Cloud (vs. on-prem)
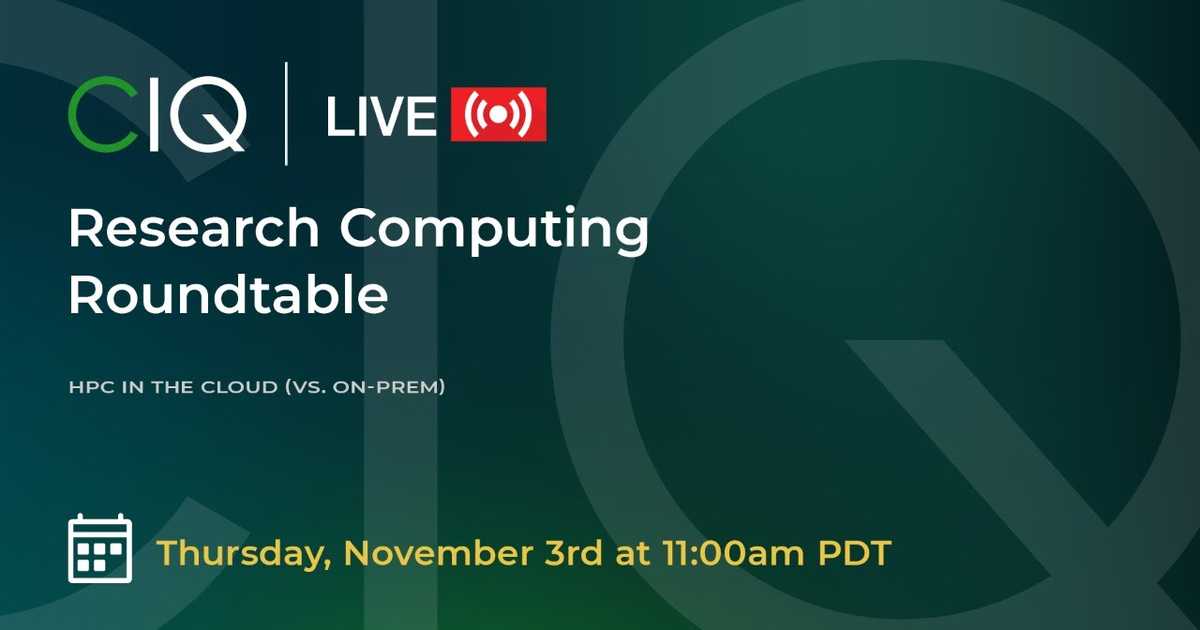
Our Research Computing Roundtable will be discussing HPC in the cloud vs. on-prem. Our panelists bring a wealth of knowledge and are happy to answer your questions during the live stream.
Obviously, the fundamental difference between cloud vs on-premise software is where it resides. On-premise software is installed locally, on your business’ computers and servers, where cloud software is hosted on the vendor’s server and accessed via a web browser and other client software.
As well as accessibility, there are a raft of other things that need to be considered when making a decision about where to host your software, including software ownership, cost of ownership, software updates and additional services, such as support and implementation. Here we will explore the pros and cons.
In this webinar, our Apptainer (formerly Singularity) experts will discuss Apptainer use-cases and features that directly help life sciences.
Webinar Synopsis:
Speakers:
-
Zane Hamilton, Vice President of Sales Engineering, CIQ
-
Gary Jung, HPC General Manager, LBNL and UC Berkeley
-
Forrest Burt, High Performance Computing Systems Engineer, CIQ
-
Jonathon Anderson, HPC System Engineer Sr. CIQ
-
Gregory Kurtzer, CEO, CIQ
-
John Hanks, HPC Principal Engineer, CZBiohub
Note: This transcript was created using speech recognition software. While it has been reviewed by human transcribers, it may contain errors.
Full Webinar Transcript:
Zane Hamilton:
Good morning, good afternoon, and good evening, wherever you are. Welcome to another research round table with CIQ. My name is Zane Hamilton. I'm the Vice President of sales engineering. CIQ is focused on powering the next generation of software infrastructure, leveraging the Cloud, hyperscale, and HPC capabilities. Our customers rely on us from research to the enterprise for the ultimate Rocky Linux, Warewulf, Apptainer support escalation. We provide deep development capabilities and solutions, all delivered in the collaborative sphere of open source. Today we're going to have an interesting topic. We're about HPC in the Cloud, which brings up a lot of emotion and excitement. I'm looking to bring everybody in. Welcome, Gary and Forrest. We'll have a couple of others join here shortly, but let's start with some intros. Here's Greg. Gary, what are you going to do?
Gary Jung:
I'm Gary Jung. I'm the Scientific Computing Group Lead at Lawrence Berkeley National Laboratory. My group runs the institutional High Performance Computing for Berkeley Lab. I'm also UC Berkeley HPC manager for their, High Performance Computing.
Zane Hamilton:
Excellent. Gary, I'm looking forward to having this conversation with you specifically. Forrest, it’s been a while. Welcome.
Forrest Burt:
Good morning, everyone. My name is Forrest Burt. I'm an HPC systems engineer at CIQ. I've been here for about a year and a half. Previously I was in the academic and national lab space as a systems administrator for a few large scale cluster systems at Boise State University and the Idaho National Lab. It's great to be on and discussing HPC in the Cloud.
Zane Hamilton:
Jonathon, welcome back.
Jonathon Anderson:
Jonathon Anderson, System Admin in a past life, very many past lives, solutions architecture with CIQ.
Zane Hamilton:
Greg, introduce yourself.
Greg Kurtzer:
Hi, I'm Greg. I'm with CIQ, Rocky Linux. I spent a long time working with Gary at LVL and Berkeley. This topic has come up a number of times in many different contexts, so I'm looking forward to this.
Leveraging the Cloud Today
Zane Hamilton:
Looking through a lot of what Gary put in, I'm not sure where to begin the topic because one of the things that most people want to talk about, talking about the cloud, is cost. I want to save that for a little bit because that could be a webinar in and of itself. But let's start. Gary, how do you guys leverage the cloud today?
Gary Jung:
It's part of our portfolio for providing computing for the researchers. One of the things we have done is we have put in place a master payer agreement to make it easier for researchers to use the cloud. Because of that, we did that a few years ago. Then we've had quite an uptake on cloud usage. Currently, we have about 200 users slash projects on a cloud, representing about 15% of our scientific workload.
Zane Hamilton:
That's interesting. That's higher than I was assuming you were going to say. Forrest, Jonathon, I know we run across this quite a lot, and that's one of the reasons that we exist. Whenever we're talking to our customers, what are you guys seeing?
Forrest Burt:
I've seen in space, especially in the academic sphere, that it's really a bell curve in terms of people's utilization of the cloud. At the enterprise level, the cloud is ubiquitous. There's not a large enterprise out there today that isn't trying to use the cloud in some way, shape, or form. For example, one of the other major sites where a lot of HPC happens is in academia. Academic institutions form a nice bell curve here. Some institutions are involved with the cloud to the point where they're deployed on multiple clouds. They have, for example, Lambdas and stuff like that that they've set up. They're involved with the tooling that the cloud provider provides to interact with our platform.
On one end, you have places like that that are super involved. They've got multi-cloud interacting with the cloud tools themselves. There's a great middle where some cloud operations are going on. They've got some GPU bursting stuff to provide more capability for people around AI. The other end of the spectrum, which I originally came out of in the academic sphere, is where there's not any cloud activity going on. Everything is traditional on-prem computing. You have a couple of big supercomputers at your data center or elsewhere. And most of your researchers are using those. In enterprise, the cloud is essentially ubiquitous. There's nobody that isn't using it. But across the academic sphere, it forms a very nice bell curve with some people who need to start using it. A lot of people are starting to get into it. And then some people that have major cloud operations going on.
Zane Hamilton:
Thank you. Jonathon, where have you been seeing cloud?
Jonathon Anderson:
Mostly, again, it is like Forrest. My background is, typically in, in the academic side of high performance computing. And there, I've mostly seen cloud computing used where it's been in an academic field that wasn't traditionally co-evolved with HPC in the first place. Many of them are GPU accelerated because much of the complexity with the non-prem HPC environment comes from the distributed memory, multi node fabric part of it. If you can get by with as many big data workloads as possible with increasingly dense single node workflows, many of those come up scaling up in a cloud context. Many tend to be what we would otherwise call embarrassingly parallel, and they can be spread out that way.
It is more of a community thing. Suppose you're coming from a community of a practice or an academic field in humanities, big data, or even genetics and genomics stuff these days. That's new to what we call high performance computing. They're coming up through the cloud. They see the benefits of a cloud computing environment in traditional HPC environments. There's a difference in technique between the two, and crossing that boundary is sometimes difficult in both directions.
Zane Hamilton:
Thank you, Jonathon. I want to point out before we get to John to let him introduce himself and answer the first question. We would love to have you guys tell us about your views on the cloud. Ask us questions and let us know how you use the cloud today. John, welcome. Introduce yourself briefly.
John Hanks:
I got here late. Should I give a history of my use of the cloud?
Zane Hamilton:
Absolutely.
John Hanks:
I've been doing life sciences stuff for a long time, and my first introduction to the cloud was in 2008. When we tried to move a sequencing pipeline and an entire sequencing operation into the cloud, it did not work. It failed miserably, and we burned through our introductory credits in a very short time. And since then, my entire career's interaction with the cloud has been migrating things back on-prem that should not have been put in the cloud in the first place. I have a long experience bringing things back home from the cloud. Only have a little experience putting things into the cloud.
Why Do You Use The Cloud?
Zane Hamilton:
That will lead to an interesting point whenever we talk about the cost. That always is the biggest driver of why things come back to the data center of the cloud. Before we get into that topic, when we look at the cloud and why people do it, it's always a question of why. We've talked a little bit about it. Jonathon, you touched on it a little bit about where people come from today and what they're comfortable with. But from an enterprise perspective or a business perspective. Why are people even looking at putting things in the cloud? Gary?
Gary Jung:
The cloud provides many bells, whistles, and convenience, and many things are readily available. We've done a few things to encourage the use of the cloud. As I mentioned, we have a master payer agreement, so the researchers at our institution don't have to use their credit cards to do this. The other thing that we do is realize that tons of services are available. Even though it may be easy, once you know what it is, you don't know what it is. We have consultants who provide consulting help to get users onto the cloud. To make a choice or figure it out. We ask them, what are you doing?
Sometimes the cloud is the right use. And then we're seeing a lot of younger researchers who have used the cloud in college or other places, and they come with that knowledge already. They're interested in continuing to use the tools they have already used. We have a number of reasons why people move to the cloud. And then, other reasons have to do with resiliency, that you may not be able to get as good of enough time on-prem because you're not multisite.
Greg Kurtzer:
I want to ask Gary a quick question. The last time I worked with Gary, we talked about clouds and how to integrate with clouds. We were talking about the pragmatic side of it, like how do you do it? How do you integrate with the scheduler? How do you create a uniform environment between on-prem and cloud, and how do you decide what jobs are best capable? You've come a long way since I've worked with you on this. What does the infrastructure look like, and what jobs are running there versus on-prem and data? How do you handle all this?
Gary Jung:
That's an interesting question. What you're describing is a hybrid environment where you're using both. What we're doing in our case is a lot simpler is that some researchers are using the cloud exclusively. Or the amount of integration between on-prem and cloud is different from what you would want because it's a very difficult problem. Some solutions are coming out, but they're expensive or complex. Or it is a challenging thing to say. I'm just going to burst into the cloud. People say that all the time. We'll do that when we need to use the cloud. And that's a harder problem to solve than people make it out to be. To get to your question, our use cases are more like we'll pick a certain use case and stick them in the cloud entirely. Somebody may want to do something, and autoML may be the right tool for them to do something quickly and easily. We'll do that, and then it'll run entirely out there.
Greg Kurtzer:
It sounds like then it's a completely separate instance or cloud HPC system that you built. Did you build a bale wolf style system up in the cloud?
Gary Jung:
We've done that too. People have asked for that or have some things work well in a cloud. There are a lot of domains where they're generating a lot of data, and they want to make the data available for download to other people. That's like a variable workload that works well with a cloud because you can auto scale. You do the data release a couple of times a year, and you need a whole bunch of computing power for that, and the rest of the time, you hardly need anything. There are things that we will choose to put out there, and they work well on the cloud.
Greg Kurtzer:
Cool, thanks. It's interesting
Shadowing HPC
Zane Hamilton:
I've got a question about that whenever we get to the cost part. It's an interesting problem we see with the cloud when we start talking about egress data or having people move data out. I have a costing question about that. People move back to the data center out of the cloud, not only for cost but also for control. John, I'm assuming that's a little bit of some of why you're doing it. People like to call it shadow IT. I'm assuming there's shadow HPC as well.
John Hanks:
Everything driving what I do to migrate stuff back is always around cost. It's never around control or capability or anything. A job is an application, and the application runs on a computer. Where that computer is irrelevant, it doesn't matter how the application got started as long as it runs—the control thing I don't understand is the direction of migrating back on-prem. A significant amount of work makes it into the cloud strictly because that's where the person trying to do the work can avoid their local IT organization. Or their local HPC group that needs to get their stuff to run correctly. That is a more insidious problem to me because that stuff that goes into the cloud doesn't need to go to the cloud. After all, it's just getting around local politics. I've run across numerous examples of this over the years of people using the cloud only because, due to policy, that's the only place they could get a machine they could control and make work.
Zane Hamilton:
That makes sense. I've seen what I mean from the enterprise side. It's always getting around policy that's always been the thing. You end up having data in places that it shouldn't be or is not supposed to be somewhere, like the cloud.
Budgeting To Use the Cloud
John Hanks:
On the cost side, maybe somebody here can explain this to me because this one blows my mind. At my previous employer, we were given a go all cloud directive to move entirely into the cloud. And we very easily and conservatively showed that it would be a 10x cost increase to go to the cloud. And for people whose titles start with C, their answer was, we don't care. It's OpEx, not CapEx. I want to know out there in the world why OpEx is free money, and CapEx is not. Because I've asked this question many times, I need help explaining it to myself, but this has repeatedly come up as a reason to go to the cloud because you can pay for it with OpEx.
Zane Hamilton:
I don't have a great answer for you, but I've seen that as well, and I've only seen it last for short amounts of time. Even though it's OpEx, I've seen that come back time and time again. Usually, within that first year, they realize they're paying a lot more money.
John Hanks:
It inevitably boomerangs, but I need help understanding the thinking that gets us to throw that boomerang. I've always needed help to grasp the accounting side of it.
Jonathon Anderson:
My impression and experience of this from having that same conversation in the past is that it's easier for the finance people to budget for. If you're doing cost recovery in an academic situation or rolling your costs into a profit margin, it's easier to see the incremental costs of those things and apply them to whatever you're getting money back in from. And the second is that you can stop paying for an OpEx anytime. Whereas if you pay all up front for capital expenditure that you stop using, that money is sunk into that resource, and you can't recover that as easily.
Zane Hamilton:
I mean it to your point, John. It is some accounting magic to use. But I've seen people have budgets and discuss how they can budget. One of the cloud migrations they did before they budgeted, and we talked about this before, was about $800,000 a month, and they got their first bill, which was almost 3 million. Even budgeting was off, and they were okay with it for a very short time. Then they pulled it all back in. It didn't even make it a year before. We can't even budget this because it was way higher than we had anticipated, and we spent a lot of money just trying to figure out what we would have, and we were wrong.
Jonathon Anderson:
And to be clear, when I say easier to budget, I don't mean actually. I mean to write the numbers down.
Zane Hamilton:
Absolutely. Gary, I don't know if that's the budgeting part of it.
Gary Jung:
I see something that could come across better to people making decisions. But it is tougher to do politically to get large funding for a large capital project. That could sometimes take years to do and a large workup to do that. Going to an OpEx is more of a solution where you're backing into it where you don't do the right thing, you do the default thing. And that's just my opinion.
John Hanks:
Many drivers, I'm tongue in cheek, mentioned getting around bad IT and controlling your machine. But I suspect many cloud drivers are political and policy based, not technical. You're not going to the cloud for technical reasons. You're going to the cloud to get around exactly what Gary just said. If you propose to build a cluster on campus, you will be inundated by people who know how to build a cluster better than you and who want to build that cluster instead of you. This is a bike shed problem of all bike shed problems. You can avoid that by just taking your money to the cloud, spending it once, and walking away with your result.
Zane Hamilton:
Forrest is laughing, I'm assuming he's never seen that before.
Forrest Burt:
It is more psychologically palatable for people to pay a small amount for disposable instances in the cloud. Every month, disposable instances in the cloud can be spun up and down, and there's not really much attached to them. It's easier to be more palatable for people to be involved in a model like that. It takes multiple years to plan a cluster and get all that budgeting in place. For example, the academic space had huge financial considerations, like where the equipment was sourced.
We can only buy part of the cluster of money places. The government would prefer that you piecemeal it out to 20 different companies and then because that's how their contracting works. It would seem basic to echo what Gary said. The actual effort it takes to stand up a cluster and the permanence of that type of thing everybody names their clusters, but who names their cloud instances or whatever they're using for something. In the end, there's more permanence attached to this obvious statement. Still, in the end, there can easily be more psychological permanence attached to an actual machine you've named and built from the ground up. You've spent more time working to create than there is with seemingly disposable cloud instances that are a lot cheaper and a lot more disposable.
Greg Kurtzer:
That's a really funny point. Gary will remember this, when a scientist takes their research funds to build a system, not only does it get named, but it gets photo opportunities. They take photos with it, standing next to it, touching it and posing with it. There is something very psychological about having that physical resource that you've just spent a huge amount of money on. Knowing that this will be used for our science to solve this problem. It turns that into a tangible win in speaking. I completely agree with that.
Gary Jung:
Did they show the program managers, show this is what we're doing this, look what we have, then they wrap it all up and what they're accomplishing with the dollars? It's part of it.
Greg Kurtzer:
For a while, I've held the opinion that I'm not as negative to cloud as John. But I have had my share of complaints about it. Again, I'm very pragmatic when I look at things, and my complaints are just about what makes sense. Like how do you do it? How do you implement it? How do you put together a system that makes sense, drives science, drives research, and solves problems? The main issue I've had with the cloud for so long is it's coming from. It's a mandate from management coming above saying, we have a cloud initiative, and we have to solve this cloud initiative. And not a question of this cloud thing, how cool is it?
And will it solve our problems? We need to be buzzword compatible. By the way, I'm not talking about LBL or DOE right now. I'm just talking in general. I've seen this over and over and over again. And, if we look at it from a pragmatic perspective, there's this thing out there with a supposedly infinite amount of resources, or at least that's what they'd like to tell us and like us to believe. There's this infinite amount of resources. It was more expensive. We have to deal with data. We have to deal with the movement of jobs and whatnot. But is there a way this can be a valuable resource to science? And how do we maximize that resource?
Jonathon Anderson:
I've been saying for a long time about the value of the cloud. Greg, you're talking about the infinite data pool, and people imagine scaling up infinitely. And there are certainly, in our CapEx versus OpEx argument, there are ways that that is a good thing where if you're building an application that serves the world and you want it to be able to scale up as the world comes online elastically. But if that's not your business model, the benefit of the cloud is that you have a functionally infinite product quality. Imagine that you divest doing it properly to someone else, but now you can carve the tiniest slice of it off and get all of the benefits of someone else having done everything the right way.
And you can scale down to a small prototype level and still benefit from that huge infrastructure. Where the benefit of the cloud comes in for research computing and even high performance computing is, that you have new workloads, new workflows, new research interests that aren't certain and whether they fit into any of the traditional existing on-prem infrastructures. We're talking about cloud versus on-prem, but there's a two-dimensional matrix where the cloud can mean either someone else's computer or the new culture of API driven computing and infrastructure as a service. That can exist on-prem too. But maybe there's some new way that someone will construct an infrastructure that better fits their research or workflow. That's something that's easiest to do in the imagined infinite thing that I can take a tiny piece of and do whatever I want with it.
That's what John was calling getting around the policy. But if you do that intentionally where you're like, we have yet to have a solution for you, play over there. Do something that works for you, and then once you have it, we'll help you scale it up. And chances are, if you have a large but relatively fixed workload that you're working towards, that's bringing it back on-prem. But you've got a lot of value in not doing the wrong thing and having to buy a bunch of infrastructures that wasn't the right thing and just having to keep it long term.
Organizations Making It Simple
Greg Kurtzer:
I'm going to jump in on that note. This is a loaded question because, as those of you who have been following for a while know, we've been working on this problem. But I am curious, in a more traditional sense, how organizations give cloud access to researchers as an introduction. You need to provide more than just cloud resources. You got to install the operating system. Install all of the applications, the libraries, and the connectors for inter process communication. Are you running a schedule? A lot of shadow IT costs now come into our shadow HPC costs that come into play when doing that. You need to do more than hand over cloud resources at least effectively to researchers generally. How do organizations make that simpler for people?
Jonathon Anderson:
That question comes from a state where HPC is a mature concept inside an organization. But if we go back even just ten years, especially 15 or 20 years, even with what we call HPC today, the answer to that was you give them grad students, and you have them figure it out and do it. That still happens with what we call traditional clustering. If you're talking about that early stage where you're doing something new, like you can't scale that, you have to throw people at it. And the cheapest people are grad students.
Internal Clouds
Zane Hamilton:
We build our internal cloud for researchers. And that's something I've been running across more and more in the conversations I've been having. Forrest, you and I have talked about this quite a bit when it comes to the cloud, and there are many things that the hyperscalers can purchase some of that more expensive hardware. You get to play with it for the first time and see, does it make sense to bring it back in. I think Jonathon, you alluded to that, but you and I have talked about that, and I think you've seen that before where people are doing that. Correct me if I'm wrong.
Forrest Burt:
As far as building out an internal cloud for people?
Zane Hamilton:
Internal clouds and then being able to try things in the hyperscalers in the cloud before they invest in friends. This leads to the hybrid cloud, which we'll talk about next.
Forrest Burt:
I have not seen so much internal cloud type being built out. Like, I haven't been involved in a lot of that personally. But it's becoming more and more of an interest to places as they realize they can invest in their infrastructure and provide those capabilities similar to the cloud. But I would say, in general, I haven't worked a ton with that personally—those types of internal clouds. I'm sure they're out there, especially with some cloud providers trying to provide their appliances. People can even stay on some of their platforms. I'm sure it's going on. But I would equate that with the concept of on-prem computing more than an internal cloud.
Zane Hamilton:
It's been interesting because I've recently talked to people who have open stack environments. What do you think of as an on-prem cloud in the enterprise?
Favorite Names Of HPC
Rose has a good question. I will open this up to everyone. Please share your favorite names of HPC. Greg, I know you have a favorite.
Greg Kurtzer:
I've got a good one, and my whole goal with putting up my hand was so that Gary doesn't get it before me. When I was at LBL with Gary, when we ordered clusters, they would typically come fully built and assembled in racks. Depending on our vendor, they'd be integrated or coming out of the merged center, and you'd roll them in. Worst case scenario, sometimes you'd get pallets of nodes, and you have to put the nodes into the rack - many vendors would do that. We ordered one cluster, and I'm not mentioning the names to protect the guilty. Still, we ordered this cluster from a vendor we didn't order from before--a big tier-one vendor--, and it came in. They won the procurement, they won the deal, they came in, and it came in on pallets. Nothing was integrated, like PCI cards and memory chips. Everything was in separate boxes. When we were done with this, the amount of extra cardboard we had to deal with was massive. We called it Ikea.
Zane Hamilton:
Nice. That's awesome. Well, you stole Gary's story, but Gary, do you have a favorite other than Ikea?
Gary Jung:
You mean about clusters, of building clusters?
Zane Hamilton:
The name of a cluster, yes.
Gary Jung:
Oh, I don't know. I'll give that some thought. We've built a lot of clusters, Greg and I. I was adding it up the other day because I was trying to recall them all. But over the last 20 years, we've put together 65 clusters.
Zane Hamilton:
No way.
Gary Jung:
Yeah, a lot.
Zane Hamilton:
That's a lot.
Greg Kurtzer:
We had one, I'm not going to mention the person's name because the scientist will be, but we had one system. This was early on that got broken into, and when we rebuilt it. We didn't know what to call it, so we named it The PI. We had a cluster named The PI. I'm sure that they made very interesting conversations in their internal group meetings.
Zane Hamilton:
That's fantastic. Anybody else?
Gary Jung:
I'll share one about myself. I'll say one that didn't make it, and then I'm glad it didn't, but we decided we have a cluster being built right now. Dell was on site installing it in racks yesterday. As Greg would say, they would do these large procurements and come in and do the installation and wiring. We decided to let the people in the research name it. And so there was a poll, and I won't say who it was, but I'll say that the second place name was Cluster McCluskey Face. I'm glad that I did not make it number one.
Zane Hamilton:
Oh, that's great.
John Hanks:
Since I worked at Cal, Gaussian was the last time I was involved in a big deployment of a single machine. Now I don't name clusters anymore. I name environments because the environment grows over time, nodes come, nodes go, and the OS upgrades. But we never have a single, large deployment anymore.
Greg Kurtzer:
Did you see Todd's comment? That was very funny.
Zane Hamilton:
That was fantastic.
Greg Kurtzer:
Black And blue.
Zane Hamilton:
Thank you for your comment, Todd.
Greg Kurtzer:
We've had clusters almost fall off the truck as they're unloading them, and sometimes it's really scary, but that happens.
Zane Hamilton:
I had a server get delivered one time when a forklift put a fork through the middle of the server, and they still delivered it and put it in a rack.
Cost Using the Cloud
It's time to have the cost conversation. This one's probably going to spark some interesting conversation. I'm going to start with John because I know the reason you're pulling stuff back into the data center is cost. Talk to me about that.
John Hanks:
There's not much to say there. It's objectively more expensive to run in the cloud for many workloads, and life sciences are one of the ones that are, like a poster child for that. Assuming you have the sump cost of a data center space, then anything you do is going to be more expensive in the cloud than it would be to do the same thing on-prem. The cost that bothers me most about using the cloud that I'm evangelical about is the opportunity cost you lose when you spend your money in the cloud. That was driven home to me again today as I listened to a presentation of a project from Biohub that moved back on-prem from Amazon. They cut their pipeline time from days to 11 hours, and the cost for them effectively went away because they're running on-prem on our cluster now.
And the last comment they made when they explained this was that now we no longer fear reprocessing data when a new algorithm comes out or we want to try something new. That's the opportunity cost lost when people spend their money in the cloud. If you buy those machines and put them on-prim, you can always use them for something. That only applies when you're paying by the hour. That's why I hate to see scientists that should be using their brain power to do science worrying about how much it will cost when I hit the inner key.
Data Egress Fee
Zane Hamilton:
Gary, we talked a little bit earlier about people wanting to allow others to access that data, and we look at the cloud, even if you have, and we'll talk about committed spending in a minute. That data egress fee, does that become a problem when people start pushing data back out, having other researchers come in and pull that data down?
Gary Jung:
The University of California manages Berkeley lab so we could take advantage of academic discounts. Without actually saying what the discount is, a lot of the discounts are between academic institutions and cloud providers. They'll wave the network egress cost so long that your compute spend is less than a certain percentage of your compute spin. The egress cost for us goes away. That's not an issue for us, but it could be for others.
Zane Hamilton:
I have seen very large egress data bills from an enterprise where you move stuff. You don't realize you try to pull it back on-prem. Following John's point, you have a massive bill you should have anticipated. The egress bills are very high, and they catch people unaware.
John Hanks:
I have about two petabytes of data right now that I sent the deep archive because it was too expensive to bring home. I just realized I'm going to bring home through Gary's cluster.
Zane Hamilton:
There you go.
John Hanks:
Problem Solved .
Cost of GPUs in the Cloud
Zane Hamilton:
One of the other things that have become interesting from my perspective over the last several years is these large hyperscalers go in and make agreements with companies. It is typical. You have a committed spend. It's usually a very large amount of money you're going to spend. Gary, this may be what you guys are in, that you have a number you've agreed on. This is what we'll commit to spending to you over this period. I've always seen that from the enterprise side rather than the HPC side, and I can see some of these higher end resources. Forrest, you and I talked about how expensive some GPUs are in the cloud. You could blow through that amount of money pretty quickly. Is that something that would help make it easier, or could that make that next bill even bigger? Is it a good thing?
Gary Jung:
To make sure I understood, is it something where you're like cost averaging or where you're committed to spending so that you're committed to a certain dollar amount?
Zane Hamilton:
That's more you're buying a discount, Gary. You commit to whatever, 20 million over the next 12 months. I get so many resources over anything that I pay for, but I get such a massive discount of what that 20 million will buy me. It just becomes a free for all. But in a large research environment, the resources are typically heavily discounted if it's considered a free for all, and you can do whatever you want. That you could still blow through that pretty quickly when you're doing web services, maybe not as much, but some of these high end GPUs that cost thousands of dollars a day could go fast.
Gary Jung:
Well, we have something like that that we're trying out with one of our providers. Where we've committed, we've looked at the spend and say, let's set a number based on history and what it should be and lock it in. That's what it's going to be for the next 12 months. If you run under it is still the same amount. If you go over it is still the same amount. What works out well is that people who do blow through a lot of their cost runs or their mistakes, which happen where people have made some very expensive mistakes, then you're protected against that. The only thing that we caution people about is that we renew it. The plan is to renew it at the end of 12 months, and for us, that'll be coming up in December. Then we'll look at the usage, and it'll reset to whatever that is. If everybody were going nuts because it was at a fixed rate, the dollar figure would be much higher when we go to re-up.
Zane Hamilton:
This goes back to John's point earlier. Gary, is that something that everybody watches over time so everybody can see where you are in that process? Does that make people nervous, especially the last month or two of that contract, to hit enter to submit their work?
Gary Jung:
This is our first time doing it. It is our first year. The spend was x, we set it at x, and it increased enough when we renewed it based on usage. That the base level will be 2x, which means everybody was paying roughly a fixed amount because we proportion it to all the researchers. They're paying about double for their cloud usage. They may have started off not using a lot and then ramped it up and were thrilled that it cost them the same amount. But now they're going to be hit with a bill being twice as much.
Zane Hamilton:
Forrest, Jonathon, are you running across these types of conversations when talking to customers and doing POCs and implementations about cloud usage and fear?
Jonathon Anderson:
From a customer perspective, I've encountered people who are already well down one path or the other. Maybe they're talking about either that or they're in one of those camps, and they want to go hybrid, but it's not to the point yet where they've encountered that fear, so not yet. Forrest, anything that you've seen?
Forrest Burt:
People I've discussed this with know the costs associated with both traditional HPC on the cloud. They're aware that you can easily burn through that budget. For the most part, I see more excited about moving to the cloud and seeing what those possibilities are than apprehension from the cis admin side. There are concerns about the cost, but in general, the theme is that we're aware that the cost of the cloud can come up, but we're interested in seeing how this can improve our operations. There's concern for cost, but there's excitement for the possibilities of the cloud and what it provides. That makes it worth it for them to investigate despite that apprehension.
Jonathon Anderson:
Gary, one of the things I'm thinking about is how to alleviate some of the fear around the possible future billing. That's the story I always hear: people did a lot of work and needed to realize how much it would cost. We say blow through a budget, but they get a huge bill they should have anticipated. That's all this postpay model where you get whatever compute you ask for, and then a bill appears at the end of the month or whatever. Are you aware of anyone offering any prepay model? Like even in on-prem when we were doing resource allocations. I was always a proponent of not just trying to theoretically bill people after the fact but always having a bucket of allocation that they have access to. If they run out, it doesn't run on the cluster for the month or period. Or they could be deprioritized. Are any hyperscalers doing that where you can put money in a bucket per account or something like that, and then their instances just shut down when they run out?
Gary Jung:
What we're doing is we've had, as I mentioned, an instance where there was a big cost overrun. We do have set limits. We'll put some reasonable limit, and you have to do it per project so that it won't run past a certain point, or you'll get a notification. Is that what you're asking?
Jonathon Anderson:
Is that a hard limit where it will stop and not bill past that? Or it says, Hey, by the way, you're exceeding your budget, and you might want to take action.
Gary Jung:
I think we set it up as a hard limit. But I'd have to go back and check.
Zane Hamilton:
That would upset people if it just hard shut off. But it is a great way to improve people's attention.
Jonathon Anderson:
It depends on what you want. If, if you're approaching this and you're like, I would want to do cloud computing stuff, but I have heard these stories about these unexpected bills coming in. Then having this available to you would give you the confidence that you can do whatever you want and you're not going to wake up to a huge bill.
Gary Jung:
Depending on the institution, the amount of cybersecurity you have for people running in a cloud may not be as good as what you have for on-prem because you're on a campus or institution with a network perimeter that you can monitor you can see all kinds of stuff. But you don't necessarily have that visibility into the cloud. In that case, a cost overrun, somebody starts hitting something really expensive like a big query, and it goes nuts over the weekend. The cost overrun thing is accurate; it could be tens of thousands of dollars in short order. Or, the other one, as I was starting to say about the cybersecurity, the hackers, somebody hacks into this, then that's a great place for them to get some free crypto mining done, which could run up fast. Having some hard shop is useful because the dollars can increase in hours.
Future of Scientific Research in the Cloud
Zane Hamilton:
Gary, where do you see the future of scientific research in the cloud? Are we, are we there? Are we at that point where we are where we are? Is this going to become more prevalent, and will more stuff move to the cloud at some point? Is it going to take over on-prem, where most research goes to the cloud?
Gary Jung:
We're moving into this just past doing computation. Before, for small researchers running just in a cloud or on on-prem, that was not a difficult problem to solve. When you're hosting large collaborations, people now expect this to have some web presence. They expect it to be up all the time. Where you might not have had the availability on your research cluster that you would have on your enterprise systems, now we're starting to see expectations where people want reliability and availability. That means accounting for outages due to work on a data center or your campus or your site or or because of some natural disaster multi-site.
We're seeing people start thinking a lot more about that when they're talking about large multi-site collaborations about the availability. Sometimes you're limited by what site you happen to be located at. We see interested people do that. The other thing is that large collaborations now do more than just computing in one place. There's more of a move to use computing wherever. They're doing multi-site collaborations and a portfolio of computing, having something available and reliable all the time to reach out and use different computing at different places. A cloud could be a good place to be the locus for that and use other things.Regarding the tools coming out, CIQ was working on some really interesting stuff that makes it location-independent. We're getting closer than ever before, and it would be nice if we can get to something that's truly hybrid, more suitable for scientific computing, and at a cost that people come to expect for scientific computing tools as opposed to, say, enterprise tools. People do multi-site computing for enterprises, but the tools are just cost-prohibitive for any scientific research institution.
Zane Hamilton:
Thank you, Gary. Greg, where do you see the future of cloud and HPC?
Greg Kurtzer:
I alluded to this earlier. It's a hybrid, from my perspective. We have to figure out how to do this in a way that is transparent to users, researchers, and the sources of the jobs. Come up with really good ways of doing this. I wonder if we call it meta orchestration or meta scheduling, but some level of higher scheduling. To figure out whether this is going to land on-prem, is this going to go to a different system, on-prem, or different data center, or is this going to the cloud? How are we going to deal with the availability regions of the cloud. How about we do all of this in a way that honestly focuses on making science better and research better and not increase the barrier of entry but lower that barrier of entry? How do we do that across cloud? Again, I'm slightly biased because that's what we've been working on. That's where the future of this needs to go. It shouldn't be either on-prem or cloud. I get it that is how we're approaching it right now because a lot of the tools still need to be better. But in the future, I think it'd be better to hybridize it all and merge it into virtual, federated systems.
Zane Hamilton:
Thank you, Greg. John, where do you see the future of the cloud?
John Hanks:
I still get Nigerian prints and spam. Since I still get that, that scam still works. I doubt the cloud will be popular in the future because there's a sucker born every minute.
John Hanks:
More seriously, we're using it as a tape archive. Rather than buy tape, we put stuff in a deep archive. There are certainly plenty of legitimate use cases for the cloud. The bursting into the cloud and standing up big, large, scale infrastructures in the cloud, that's very dependent on available finances, and it's all fun and games the last five years, six years because we've been in a bubble. That bubble burst, and that money to spend on the cloud is going to go away. When people start tightening their budget, that will be the first thing they cut. Either they'll either start doing it on-prem cheaper or not do that work because they don't have the budget for it. It'll always be there. It's got its uses, but for these large projects, it will always depend on the financial backing behind it and the ability to waste money.
Gary Jung:
I wonder if we have hit it hard enough, but I want to cover something about the cloud versus on-prem costs. The reason is that I had done an extensive study recently on that cost at Berkeley Laboratory. We did it about 11 years ago at NERSC, the Magellan project. But now we're at the point where we're at the limits of our current data center, and we would have to make some large capital investments in a new data center or modular data center. Now we're doing your due diligence. Let's look at the cost of cloud versus on-prem. Before we say what, we found out, we have 20 years of experience in buying hardware and software, so we know what the hardware investment is over a sustained period. Then the same with the data center. The data center's gone through several upgrades, including a recent $4 million upgrade to replace the transformers at a chiller so that we can get some additional capacity out of that. I included all those costs with the labor costs and cybersecurity. To cut to the chase, the amount of what we deliver in cycles and storage, not capacity, is just what we deliver to researchers. It's about a list price five times more expensive in a cloud. With our institutional discounts, it is four times as much. Then if you try to whittle that down further and use reserved instances or committed instances, you can get it down to like 3x.
FIRM Lab had a paper out there. If you go look it up and they said, okay, we're only going to do high throughput workloads, we're only going to use spot instances where we can get it at 25% of the pot price. They did it all, made all these concessions to the workload, and the best they could do is make it one and a half times as the cloud was still one and a half times more than on-prem costs. After we had done this, we talked to some other institutions, so some other large institutions took a similar approach and came up with similar numbers. I feel good about the analysis. If people are interested, I can walk them through it. But I wanted to say something about the cost because of an analysis we did, which was thorough.
Zane Hamilton:
That's great, Gary because Donovan has a question. Donovan Campbell asked, how do we predict future costs and how to migrate? That's a, maybe, a sidebar conversation, but that is a great question. Sorry, Gary.
Gary Jung:
Oh, that's it. I'm done.
Zane Hamilton:
Very good. We are right up at the end of time, guys. I'm sorry to cut it off; I want to be respectful. I appreciate Gary, and John, as always, really appreciate your time. Greg, Jonathon, and Forrest, it's always good to see you. Thanks for stopping by, guys. If you like this, go like and subscribe. We enjoy the interaction with you, and we're looking forward to next week. Thanks, guys. Thank you.