What I Saw at the Hyperion HPC User Forum Fall 2024
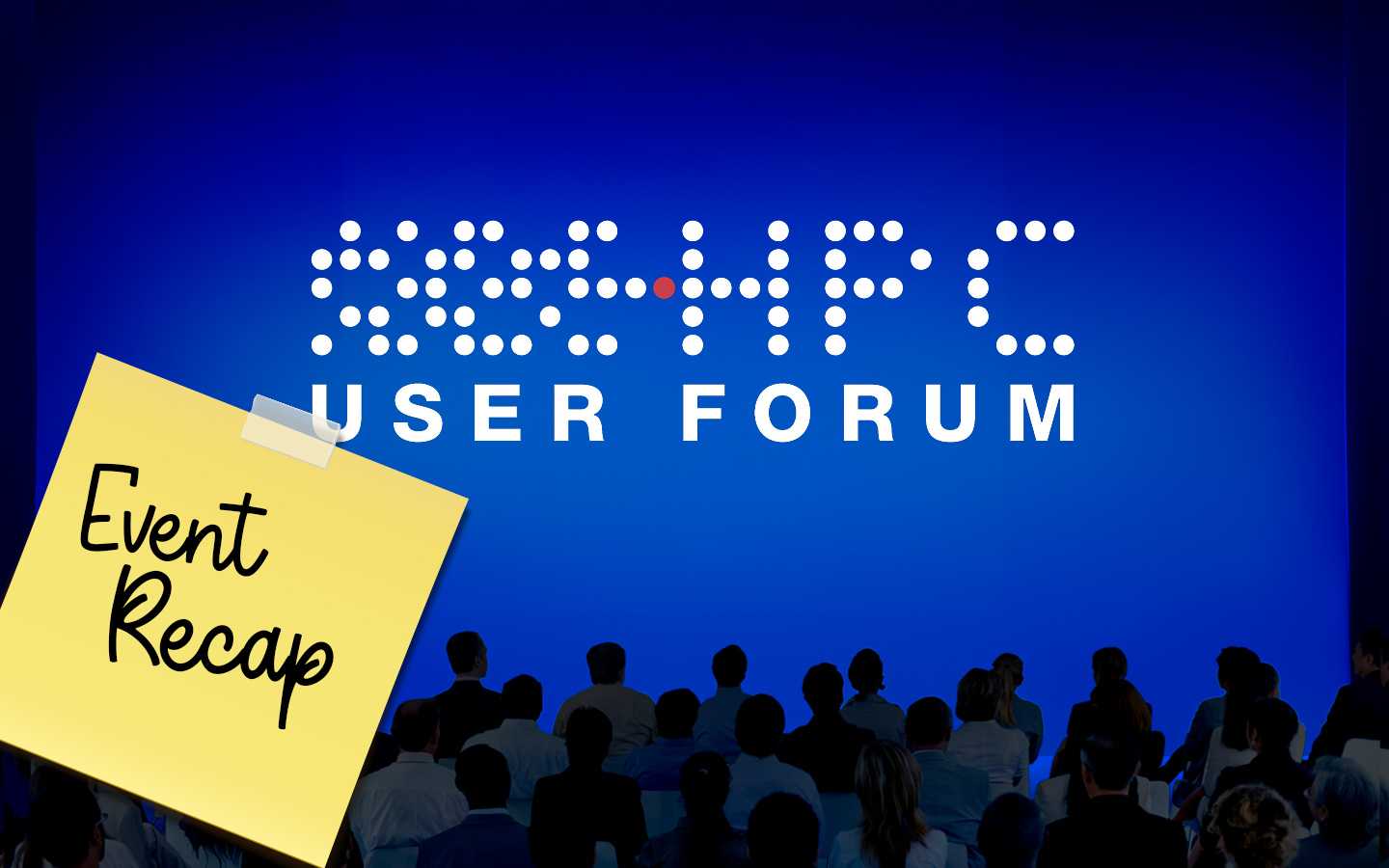
I had a great time attending and speaking about CIQ at the recent HPC User Forum at Argonne National Lab in early September, and wanted to put together a quick post about what I saw at it, what stood out, and what the conference experience was like.
The Setting: Argonne National Lab
Argonne National Lab is located on the outskirts of Chicago - which I've never been to before. The lab is located in a wooded area - Chicago proper is only occasionally visible on the way there, and the city itself most prominently only made an appearance on the way back to the airport - when I caught just a quick glimpse of the Sears Tower and all - and on climbout from MDW - when I got a beautiful view of the skyline. The national lab facilities are always fun to get to visit.
The Content: Two Days of HPC and AI
The conference was two days of mostly non-stop attention to the finer details of HPC (high performance computing) and AI (artificial intelligence). But - with a serious bent to it. Far from the usual hype about generative AI that's out there these days, this conference featured heavy hitters from the defense, national lab, and private industry spaces, people who are actually using and iterating on the forefront of AI. I was struck by how real the use cases were - autonomous control of nuclear reactors, real-time data processing interfaces for supercomputing clusters - right now, it seems like we are throwing AI at everything and just seeing what works and what doesn’t. There's currently a lot of hype in the tech world about what AI can really do - but there's also a lot of serious promise around AI that's worth paying attention to.
The Technology: A Forefront of AI and Supercomputing
Overall, I was impressed with the state of technology presented by the other speakers. First off - there are a ton of different techniques being worked on to improve AI right now beyond just being flat models that we interact with via text queries. What I saw here is the rise of the agentic AI model - where an AI like a large language model isn't just limited to what it knows, but can essentially work independently in certain ways - like spin up other copies of itself ("task decomposition"), consult a panel of other AI experts that collectively form an answer ("mixture of experts"), or even retrieve data from sources like the internet ("retrieval-augmented generation, or RAG").
Task decomposition is allowing an AI to realize that its own context/memory window is shorter than necessary to solve a complex problem, so instead, this AI is used to generate a set of tasks that are then farmed out to other copies of itself that are spun up for that purpose. This "decomposition" of a task allows for an AI-optimized solution to be put together for more and more complex problems presented to the AI, and it allows for the AI to work towards this optimal solution much more independently than before. With context windows getting longer and longer, already increasing naturally what a single instance of a model can do, giving them the ability to coordinate with each other is only making LLMs more powerful.
A "mixture of experts" system is essentially a bunch of different models under the hood, each one finely tuned for a different purpose - thus being an individual "expert" in a certain topic. A mixture of experts system could include just a handful of different models all working together, or up to over a hundred in the case of one software/hardware appliance platform I saw presented at the conference. This differentiation of experts potentially allows for better answers to more specific subjects than can be achieved with a more monolithic model.
You may have noticed ChatGPT got the ability a while back to search the internet as a part of generating a response - this is essentially retrieval-augmented generation. Training an AI model is expensive in any case and takes a lot of time - and retraining on new information is a similarly heavy task. This need to constantly update models, for example, to keep up with current events, can thus be difficult and time-consuming. Allowing a model to search for and peruse live information from the internet and then use its native ability to understand information to synthesize and contextualize that information is a promising way to keep models more up to date than with constant retraining.
Hyperion's talks about the status of the industry were incredibly informative and, as I always hope for with these analytics companies, helped to challenge a lot of what I thought about the industry. With the number of large cluster deployments still being done outside of the cloud, especially for high security and defense applications, it's evident that on-prem HPC is here to stay. It’s also evident that AI is only becoming a larger and larger use case as people realize how generically industrially useful it can be when applied correctly.
Among other talks, we saw information about autonomous detection of nuclear reaction cybersecurity threats, a GPT-like system being deployed on the Aurora supercomputer to enable real-time processing of research data for ANL researchers, and a number of great vendor talks about the offerings of the conference sponsors around HPC - including from yours truly partnered with Montis Technologies, linked here and above.
The People: A Who’s Who of Modern HPC
HPC is a small field and it's always a lot of fun to run into folks from the space around and about at these events. Among the highlights were an old friend from Inspire Semiconductor who had some great info about where their RISC-V chips are at; a new connection in an HPC engineer from ORNL who is in their early career much like the author of this post and was interesting to discuss as much with; along my own colleagues David Godlove and Michael Ford, who I always enjoy an opportunity to catch up with. We were there to represent CIQ at our vendor table and enjoyed the opportunity to meet so many folks at the conference and discuss our company offerings. Michael showed us the best spot for pizza in Chicago one night, and in general, the lab was a great setting to chill out at during our conference evenings.
Tours of ANL’s Cutting-Edge Facilities: Aurora Cluster and the APS At the end of the first day, we got the opportunity to tour the HPC machine rooms at ANL - including a view of the Aurora supercomputer, the world's fastest AI supercomputer, which was incredible to see. The scale of on-prem HPC deployments can only really be appreciated by seeing them in-person and up-close, and this was an incredible chance to see a production environment. Imagine - all those cooling loops, all that network hardware, all those blinking lights - what a sight.
Though a difficult second, almost as cool as the Aurora cluster was getting to see the Advanced Photon Source on the second day - a particle accelerator that generates hard x-rays brighter than almost anything else on earth. As our tour guide put it, “X-rays allow us to illuminate things on a molecular level - so we do all kinds of different science here." The scale of engineering it takes to bring something like that to life is awe-inspiring, and seeing part of the interior ring with a thousand tubes, pipes, and banks of electronics was quite a treat. The control room of a particle accelerator is really something to see - seats for the operators in front of banks upon banks of monitors, with diagrams of the ring status shown on mainframes at the back in green CRT.
Wrap-Up: Conclusions on the Future of HPC and AI
Overall, we had a an educational and productive conference. The talks advanced my understanding of HPC and gave me a great insight into what's actually going on in AI right now at some of the most advanced research centers in the United States. While the HPC/AI space remains fluid and no one technology is guaranteed to stick around in the fast-moving space that makes up modern HPC and AI in production, there's a lot of extremely dedicated people working to figure out what's worthwhile and what isn't - and regardless of anything, AI in every form is here to stay - and HPC continues to be the primary driver of scientific and engineering progress as the computational backbone of nearly everything we do today.